Investigations on Self-supervised Learning for Script-, Font-type, and Location Classification on Historical Documents
PROCEEDINGS OF THE 2023 INTERNATIONAL WORKSHOP ON HISTORICAL DOCUMENT IMAGING AND PROCESSING, HIP 2023(2023)
摘要
In the context of automated classification of historical documents, we investigate three contemporary self-supervised learning (SSL) techniques (SimSiam, Dino, and VICReg) for the pre-training of three different document analysis tasks, namely script-type, font-type, and location classification. Our study draws samples from multiple datasets that contain images of manuscripts, prints, charters, and letters. The representations derived via pre-text training are taken as inputs for k-NN classification and a parametric linear classifier. The latter is placed atop the pre-trained backbones to enable fine-tuning of the entire network to further improve the classification by exploiting task-specific label data. The network's final performance is assessed via independent test sets obtained from the ICDAR2021 Competition on Historical Document Classification. We empirically show that representations learned with SSL are significantly better suited for subsequent document classification than features generated by commonly used transfer learning on ImageNet.
更多查看译文
关键词
self-supervised learning,document analysis,classification
AI 理解论文
溯源树
样例
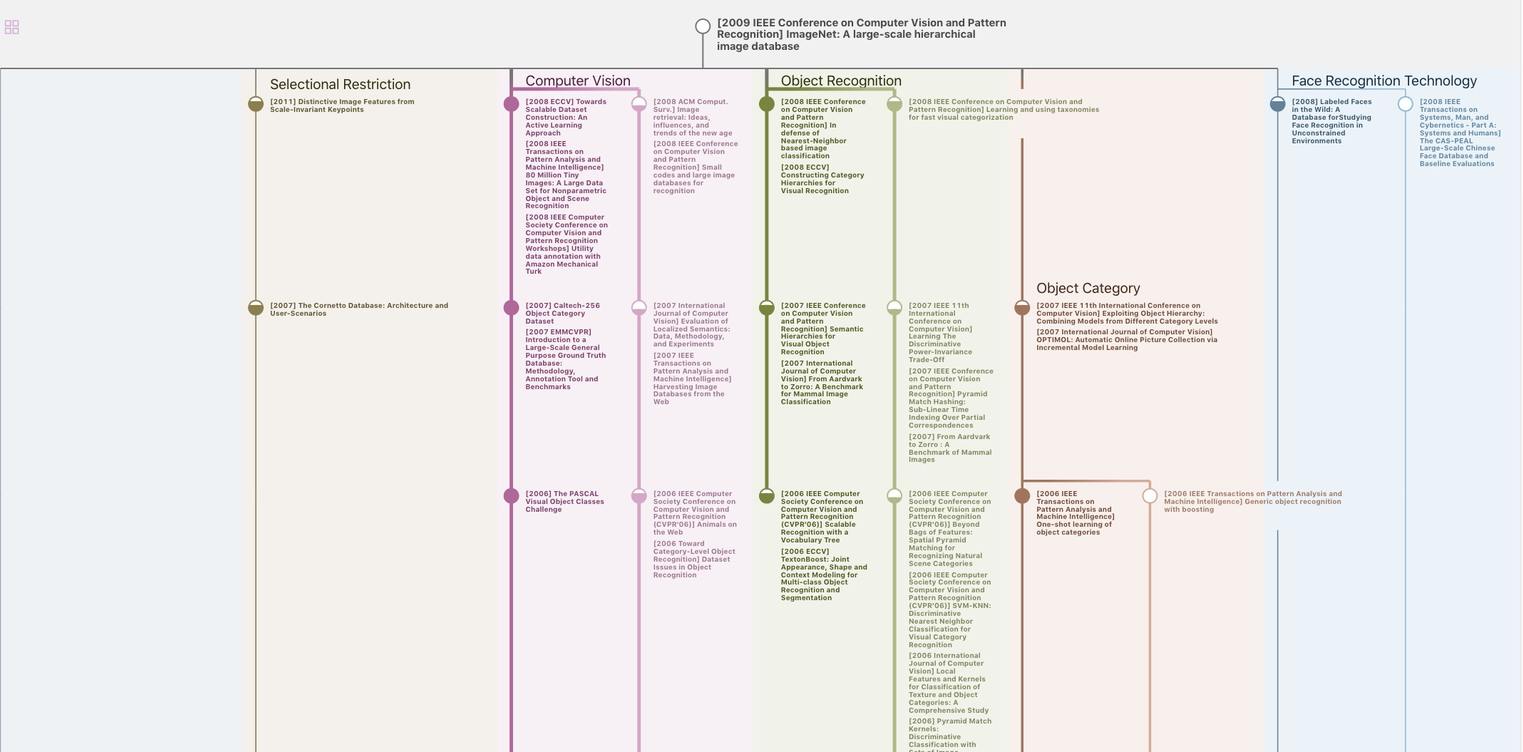
生成溯源树,研究论文发展脉络
Chat Paper
正在生成论文摘要