Generalization of Fine Granular Extractions from Charts.
ICDAR (2)(2023)
摘要
Current approaches for high precision fine-grained visual extractions from charts are highly data intensive requiring thousands of annotated samples. Annotating a dataset and retraining for every new chart type with a shift in the spatial composition of chart elements, text role regions, legend preview styles, chart element shapes and text-role definitions, is a time-consuming and costly affair. Current approaches struggle to generalize to new chart types with a distributional shift across the above dimensions. In this paper, we define a novel attention and dynamic filtering based approach for extracting chart elements and identifying text-role regions, achieving SOTA results for visual extraction on charts for the PlotQA dataset, surpassing existing approaches by an mAP of 2.81% @0.90 IOU. More importantly, the methods proposed are designed to adapt to unseen chart types having the above mentioned shifts in chart element distributions. We demonstrate the generalization capabilities of our models trained on the PlotQA train set by providing chart extraction results on out-of-distribution charts selected from the LeafQA dataset. We achieve an mAP of 90.64% and 92.18% for @0.90 IOU for this well-curated out-of-distribution chart data in zero and few-shot settings, respectively.
更多查看译文
关键词
fine granular extractions,charts,generalization
AI 理解论文
溯源树
样例
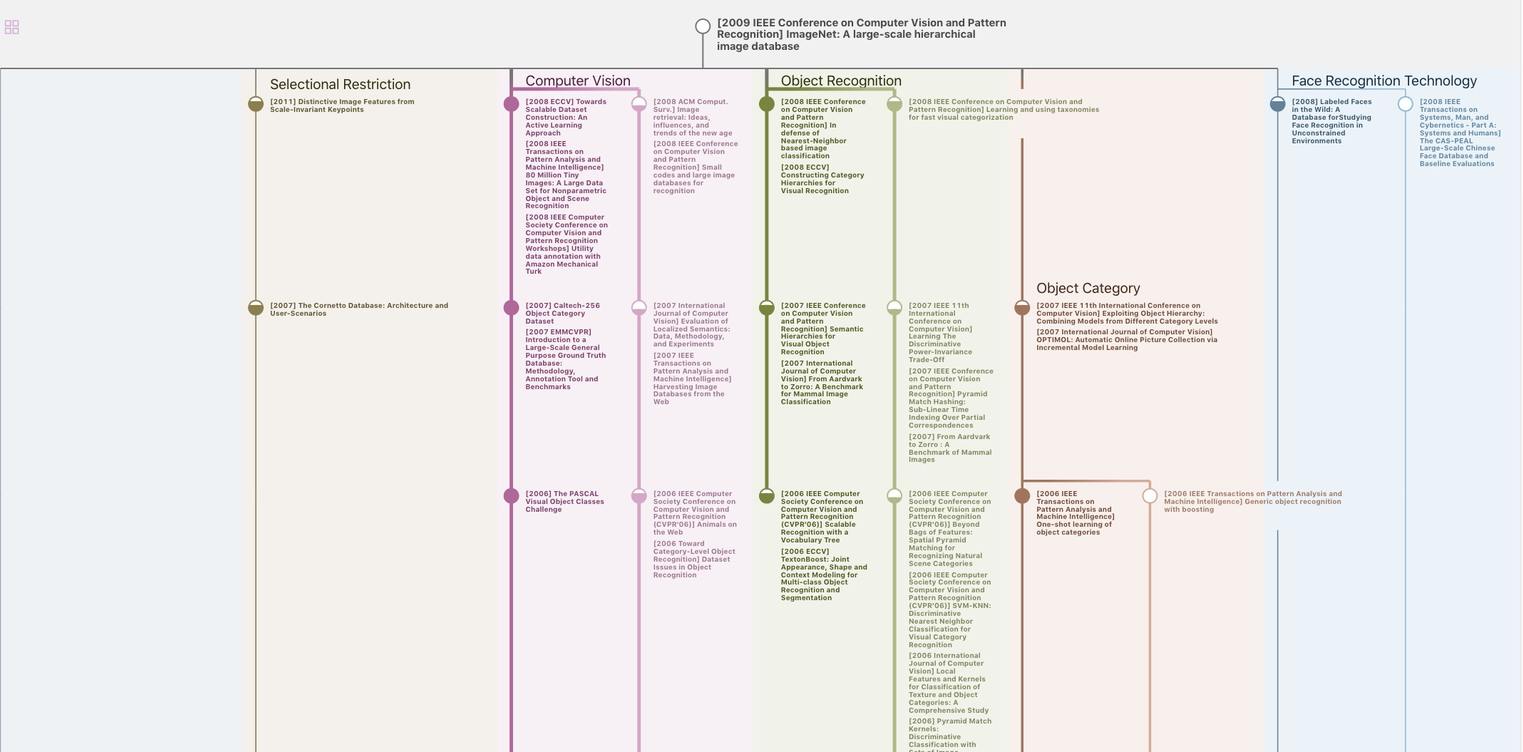
生成溯源树,研究论文发展脉络
Chat Paper
正在生成论文摘要