Towards Accelerating the Adoption of Federated Learning for Heterogeneous Data
PROCEEDINGS OF THE 16TH ACM INTERNATIONAL CONFERENCE ON PERVASIVE TECHNOLOGIES RELATED TO ASSISTIVE ENVIRONMENTS, PETRA 2023(2023)
摘要
Federated Machine Learning (FML) is a distributed machine learning approach that solves basic AI and data problems such as data heterogeneity, privacy preservation, and data ownership. This technology enables organizations to collaborate on the model building while retaining control over their data, making it particularly useful when data is sensitive or too large to be collected in a central location. Numerous open-source frameworks for FML have been developed, each with different capabilities. In this paper, we use a popular framework to implement a proposed algorithm and tackle the significant problem of data heterogeneity in AI. Specifically, we integrated the FEDMA algorithm to simulate the data heterogeneity problem with the FEMNIST dataset, a widely used benchmark dataset in the research community.
更多查看译文
关键词
Federated Machine Learning,Data heterogeneity,Personalised Machine Learning
AI 理解论文
溯源树
样例
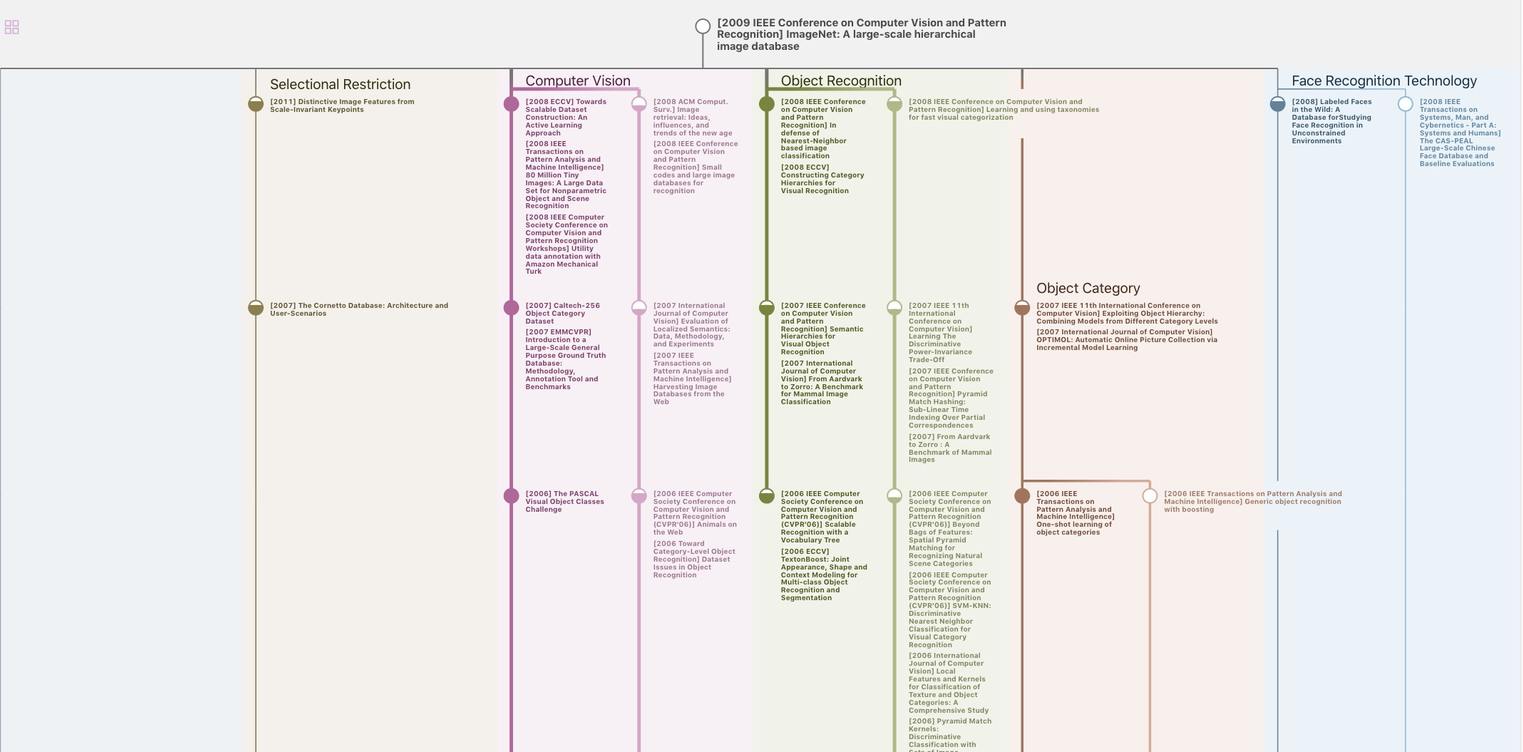
生成溯源树,研究论文发展脉络
Chat Paper
正在生成论文摘要