Less Labels, More Modalities: A Self-Training Framework to Reuse Pretrained Networks.
ICPR Workshops (3)(2022)
摘要
Remote sensing largely benefits from recent advances in deep learning. Beyond traditional color imagery, remote sensing data often features some extra bands (e.g. multi or hyperspectral imagery) or multiple sources, leading to the so-called multimodal scenario. While multimodal data can lead to better performances, it also requires to design specific deep networks, to collect specifically-annotated datasets, and to perform full retraining of the models. However, a major drawback of deep learning is the large number of annotations that is required to ensure such a training phase. Besides, for some given task and modality combination, annotated data might not be available, thus requiring a tedious labeling phase. In this paper, we show how to benefit from additional modalities without requiring additional labels. We propose a self-training framework that allows us to add a modality to a pretrained model in order to improve its performance. The main features of our framework are the generation of pseudo-labels that act as annotations on the new modality, but also the generation of a pseudo-modality corresponding to the labeled monomodal dataset. Experiments on the ISPRS Potsdam dataset, where we complement color orthophotography with a digital surface model, shows the relevance of our approach, especially for land cover classes that can take advantage of the two modalities.
更多查看译文
关键词
networks,less labels,self-training
AI 理解论文
溯源树
样例
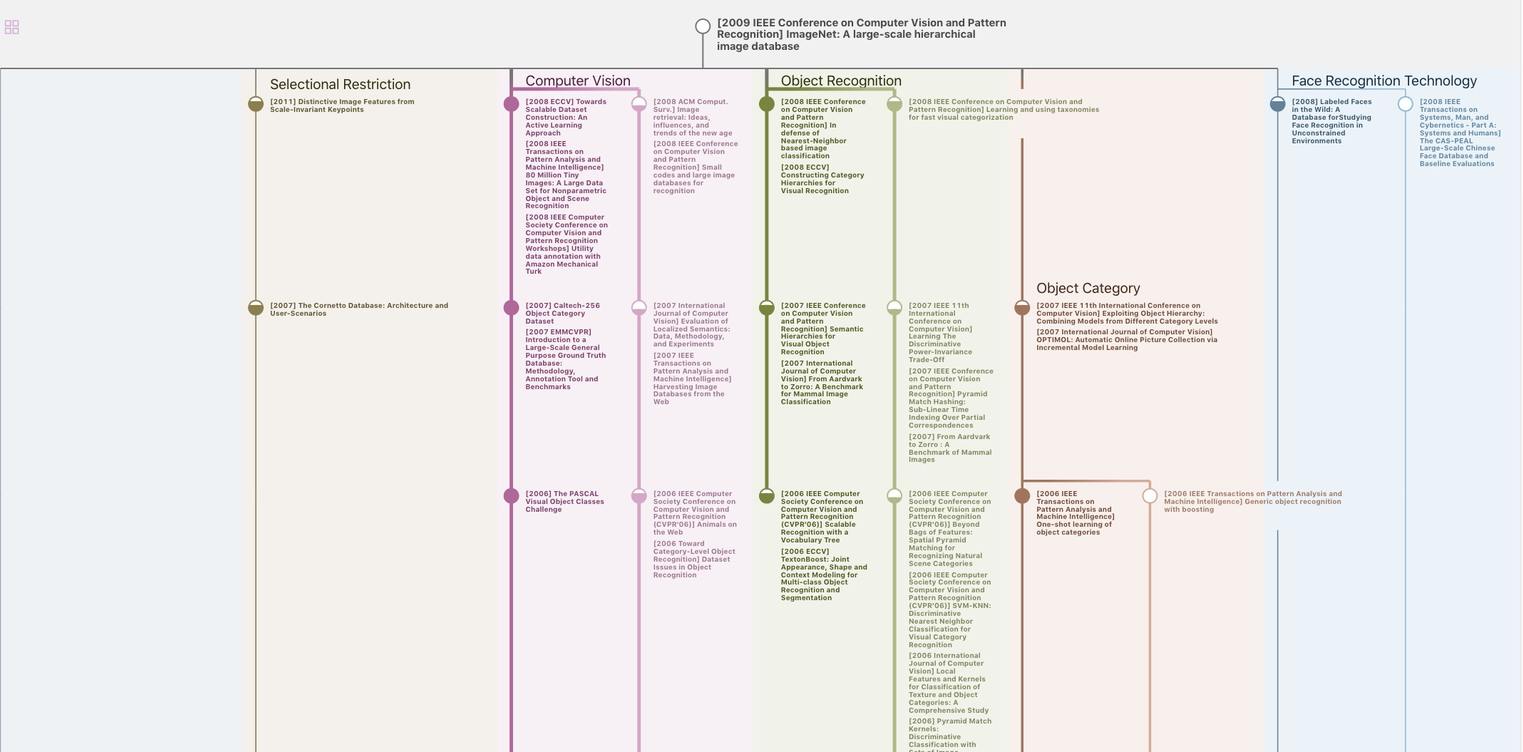
生成溯源树,研究论文发展脉络
Chat Paper
正在生成论文摘要