Algorithm-hardware co-design for efficient brain-inspired hyperdimensional learning on edge (extended abstract)
IJCAI(2023)
摘要
In this paper, we propose an efficient framework to accelerate a lightweight brain-inspired learning solution, hyperdimensional computing (HDC), on existing edge systems. Through algorithm-hardware co-design, we optimize the HDC models to run them on the low-power host CPU and machine learning accelerators like Edge TPU. By treating the lightweight HDC learning model as a hyper-wide neural network, we exploit the capabilities of the accelerator and machine learning platform, while reducing training runtime costs by using bootstrap aggregating. Our experimental results conducted on mobile CPU and the Edge TPU demonstrate that our framework achieves 4.5 times faster training and 4.2 times faster inference than the baseline platform. Furthermore, compared to the embedded ARM CPU, Raspberry Pi, with similar power consumption, our framework achieves 19.4 times faster training and 8.9 times faster inference.
更多查看译文
AI 理解论文
溯源树
样例
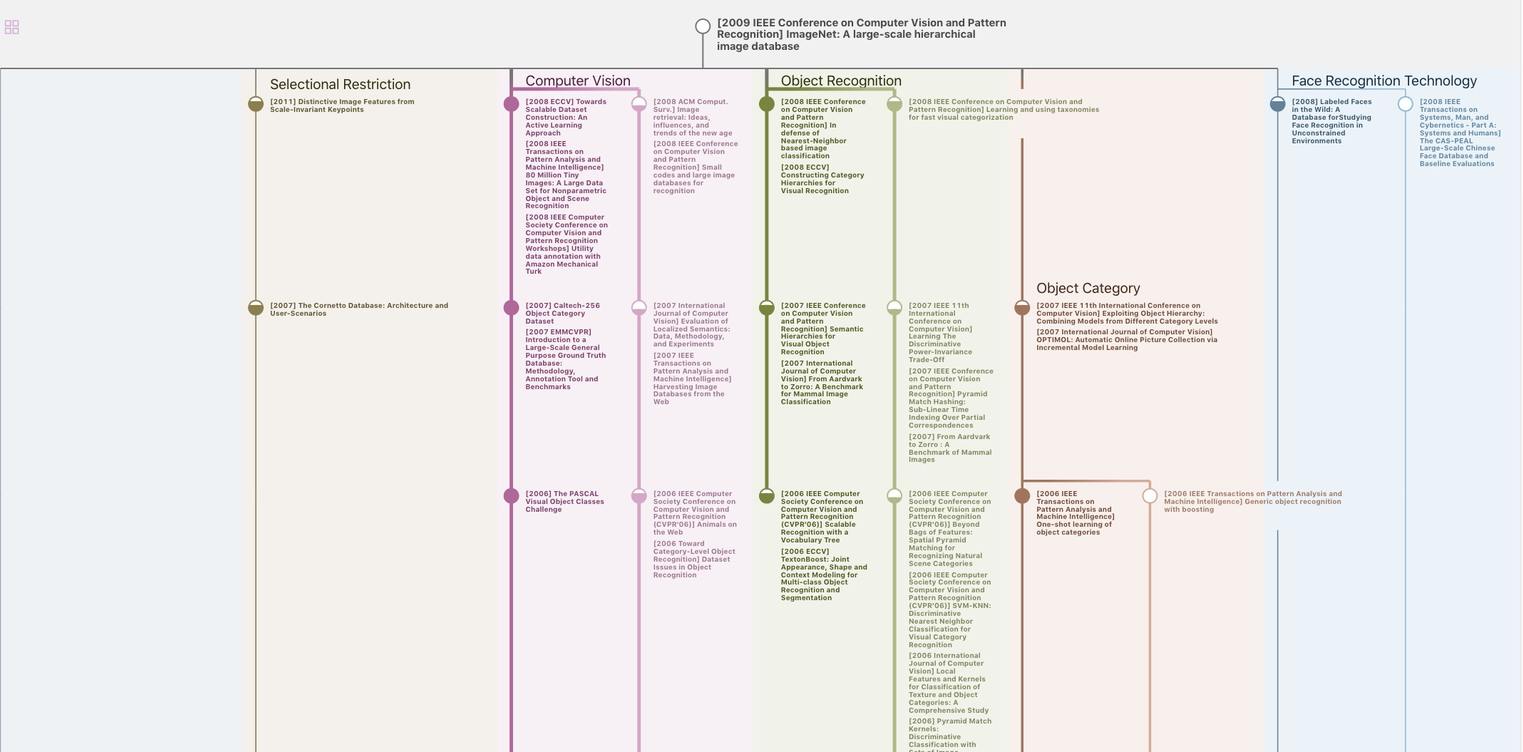
生成溯源树,研究论文发展脉络
Chat Paper
正在生成论文摘要