Keeping people active and healthy at home using a reinforcement learning-based fitness recommendation framework
IJCAI(2023)
摘要
Recent years have seen a rise in smartphone applications promoting health and well being. We argue that there is a large and unexplored ground within the field of recommender systems (RS) for applications that promote good personal health. During the COVID-19 pandemic, with gyms being closed, the demand for at-home fitness apps increased as users wished to maintain their physical and mental health. However, maintaining long-term user engagement with fitness applications has proved a difficult task. Personalisation of the app recommendations that change over time can be a key factor for maintaining high user engagement. In this work we propose a reinforcement learning (RL) based framework for recommending sequences of bodyweight exercises to home users over a mobile application interface. The framework employs a user simulator, tuned to feedback a weighted sum of realistic workout rewards, and trains a neural network model to maximise the expected reward over generated exercise sequences. We evaluate our framework within the context of a large 15 week live user trial, showing that an RL based approach leads to a significant increase in user engagement compared to a baseline recommendation algorithm.
更多查看译文
AI 理解论文
溯源树
样例
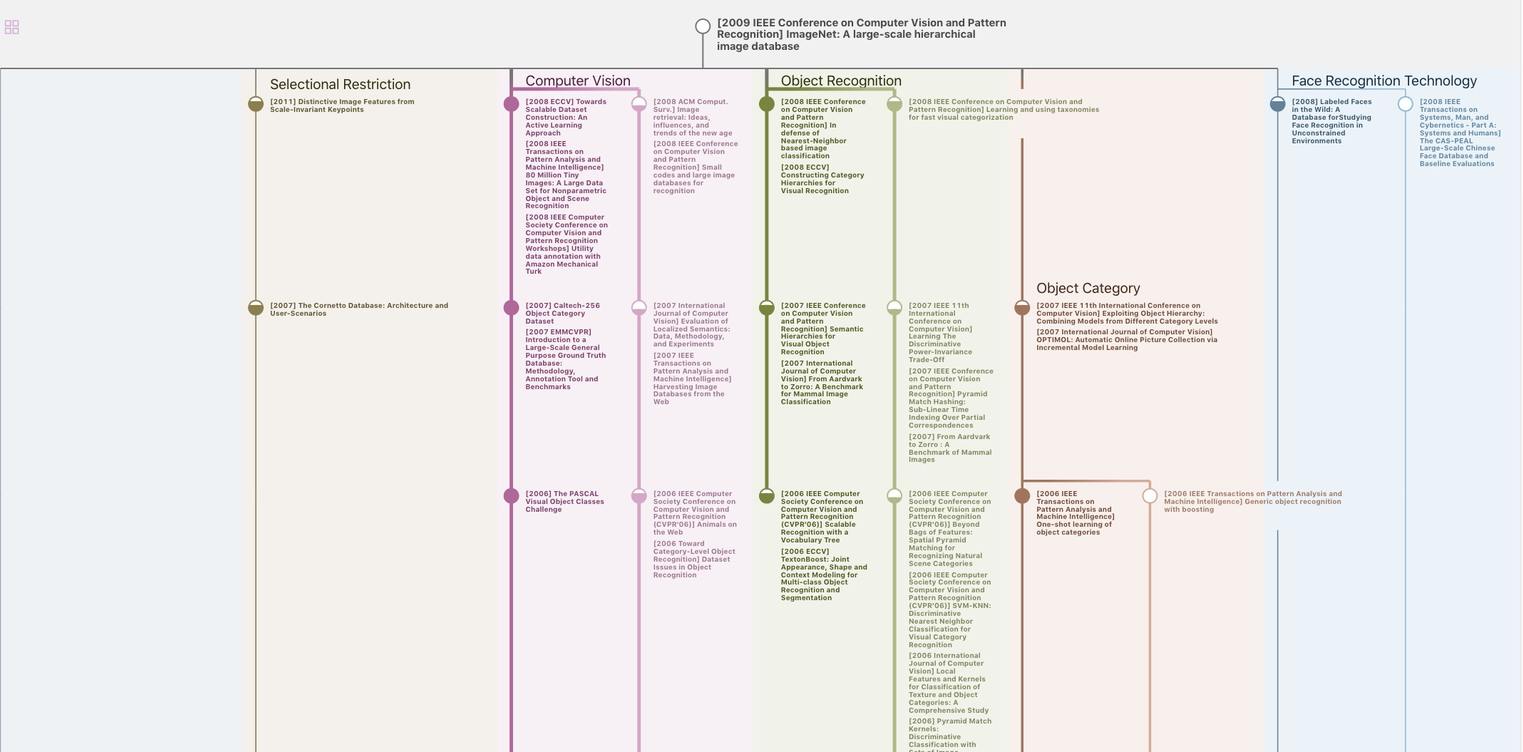
生成溯源树,研究论文发展脉络
Chat Paper
正在生成论文摘要