Enhancing the Linear Probing Performance of Masked Auto-Encoders.
ICPR Workshops (1)(2022)
摘要
This paper especially investigates the linear probing performance of MAE models. The recent Masked Image Modeling (MIM) approach is shown to be an effective self-supervised learning approach. These models usually mask out some patches of images and require the model to predict specific properties of those missing patches, which can either be raw pixel values or discrete visual tokens learned by pre-trained dVAE. Despite the promising performance on fine-tuning and transfer learning, it is often found that linear probing accuracy of MAE is worse than that of contrastive learning. This is concerning, demonstrating that features out of MAE network may not be linear separable. To investigate this problem, we consider incorporating contrastive learning into the MAE modeling, to examine the mechanism behind linear probing. We design specific head architectures to associate with MAE, which allows us to include additional feature constraints inspired from Barlow Twins method. The motivation behind is our hypothesis that features learned by MIM would focus more on image style and high-frequency details, while features learned by Barlow Twins will focus more on image content. Our motivation then is to select a trade-off between the two types of features in order to improve the linear probing accuracy of MAE without hurting fine-tuning and transfer learning performance. Empirical results demonstrate the effectiveness of our method. We achieve 27.7% top1 accuracy at linear probing with ViT-Tiny as our backbone, which outperforms the MAE protocol under the same settings by 1.6%.
更多查看译文
AI 理解论文
溯源树
样例
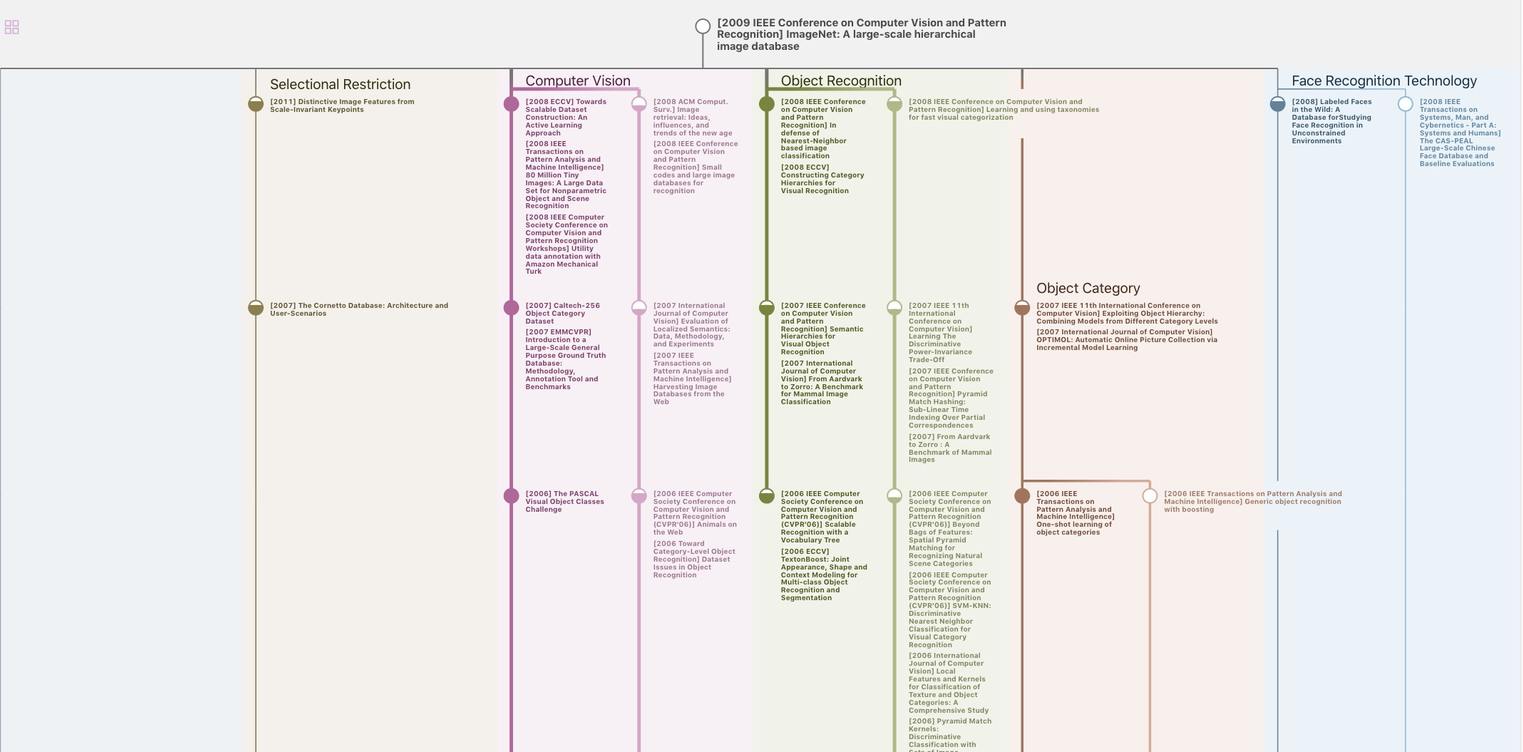
生成溯源树,研究论文发展脉络
Chat Paper
正在生成论文摘要