DAC-PPYOLOE plus : A Lightweight Real-time Detection Model for Early Apple Leaf Pests and Diseases under Complex Background
COMPSAC(2023)
摘要
Early detection of apple pests and diseases is beneficial to ensure the healthy development of the apple industry. However, previous detectors typically suffer from low accuracy and poor timeliness, limiting their further application in real scenarios as well as complex backgrounds. A real-time early apple leaf pests and diseases detection model is proposed in this paper, dubbed DAC-PPYOLOE+. Firstly, an efficient adaptive feature fusion strategy is utilized to improve the detection capability of the model under complex backgrounds. Meanwhile, a new ESPBlock with dilated convolution implemented by depthwise separable convolution is designed, which greatly reduces the number of parameters and enhances the adaptability to different scale targets. Furthermore, a novel skip information transmission structure is proposed to fully exploit the information of deep and shallow feature maps, which is specifically used for small target detection. Compared to the baseline model, DAC-PPYOLOE + has a smaller number of parameters and achieves 44.9% AP on the COCO test, which is 3.6% higher, and the inference speed is 10.0 ms, faster about 2 ms. Experimental results of comparison with various advanced detection methods show that the proposed model outperforms various advanced algorithms in handling early apple leaf pests and diseases detection tasks, indicating that DAC-PPYOLOE+ provides effective technical support for real-time and accurate detection of early apple leaf pests and diseases under complex backgrounds.
更多查看译文
关键词
Apple leaf pests and diseases,PPYOLOE+,complex background,real-time inference
AI 理解论文
溯源树
样例
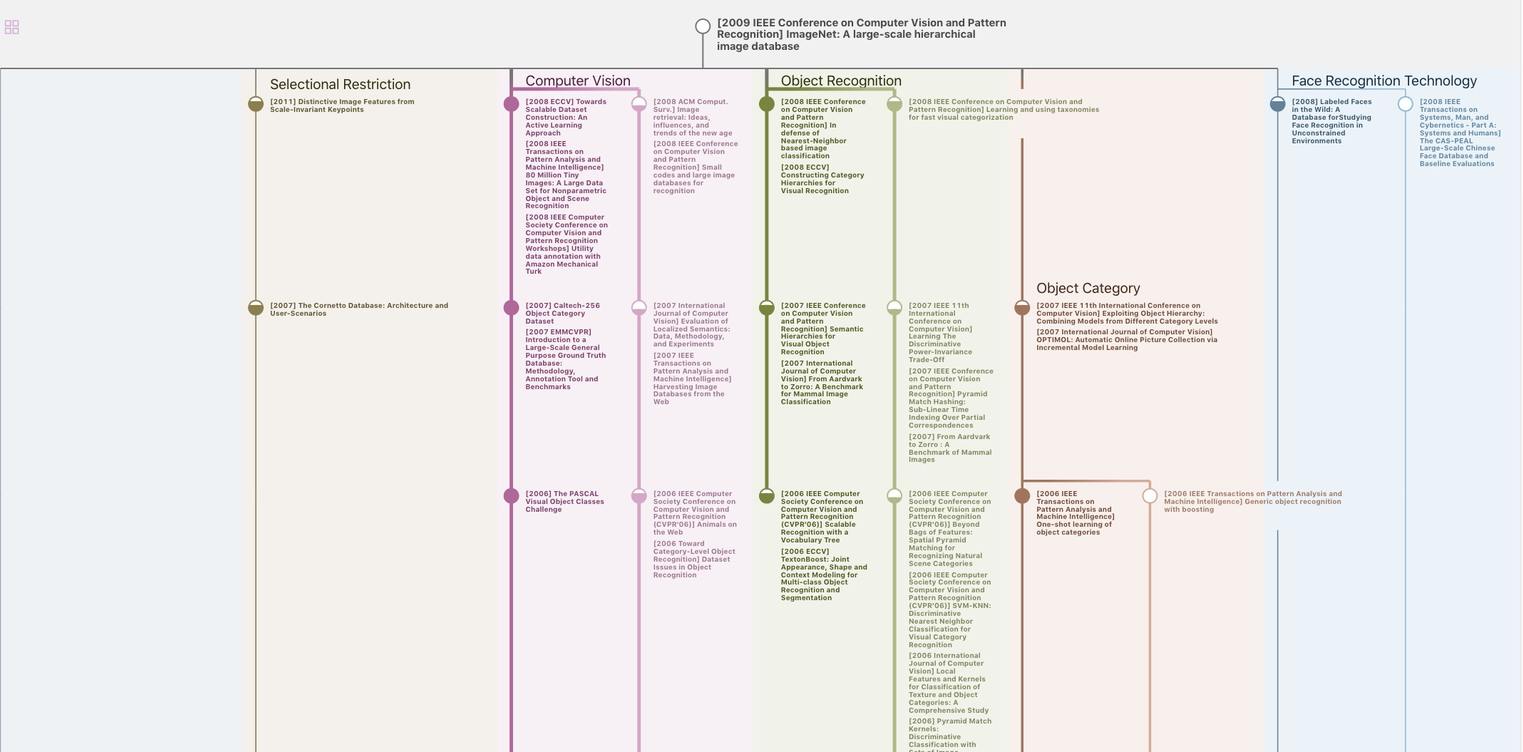
生成溯源树,研究论文发展脉络
Chat Paper
正在生成论文摘要