RelKD 2023: International Workshop on Resource-Efficient Learning for Knowledge Discovery
PROCEEDINGS OF THE 29TH ACM SIGKDD CONFERENCE ON KNOWLEDGE DISCOVERY AND DATA MINING, KDD 2023(2023)
摘要
Modern machine learning techniques, especially deep neural networks, have demonstrated excellent performance for various knowledge discovery and data mining applications. However, the development of many of these techniques still encounters resource constraint challenges in many scenarios, such as limited labeled data (data-level), small model size requirements in real-world computing platforms (model-level), and efficient mapping of the computations to heterogeneous target hardware (system-level). Addressing all of these metrics is critical for the effective and efficient usage of the developed models in a wide variety of real systems, such as large-scale social network analysis, large-scale recommendation systems, and real-time anomaly detection. Therefore, it is desirable to develop efficient learning techniques to tackle challenges of resource limitations from data, model/algorithm, or (and) system/hardware perspectives. The proposed international workshop on "Resource-Efficient Learning for Knowledge Discovery (RelKD 2023)" will provide a great venue for academic researchers and industrial practitioners to share challenges, solutions, and future opportunities of resource-efficient learning.
更多查看译文
AI 理解论文
溯源树
样例
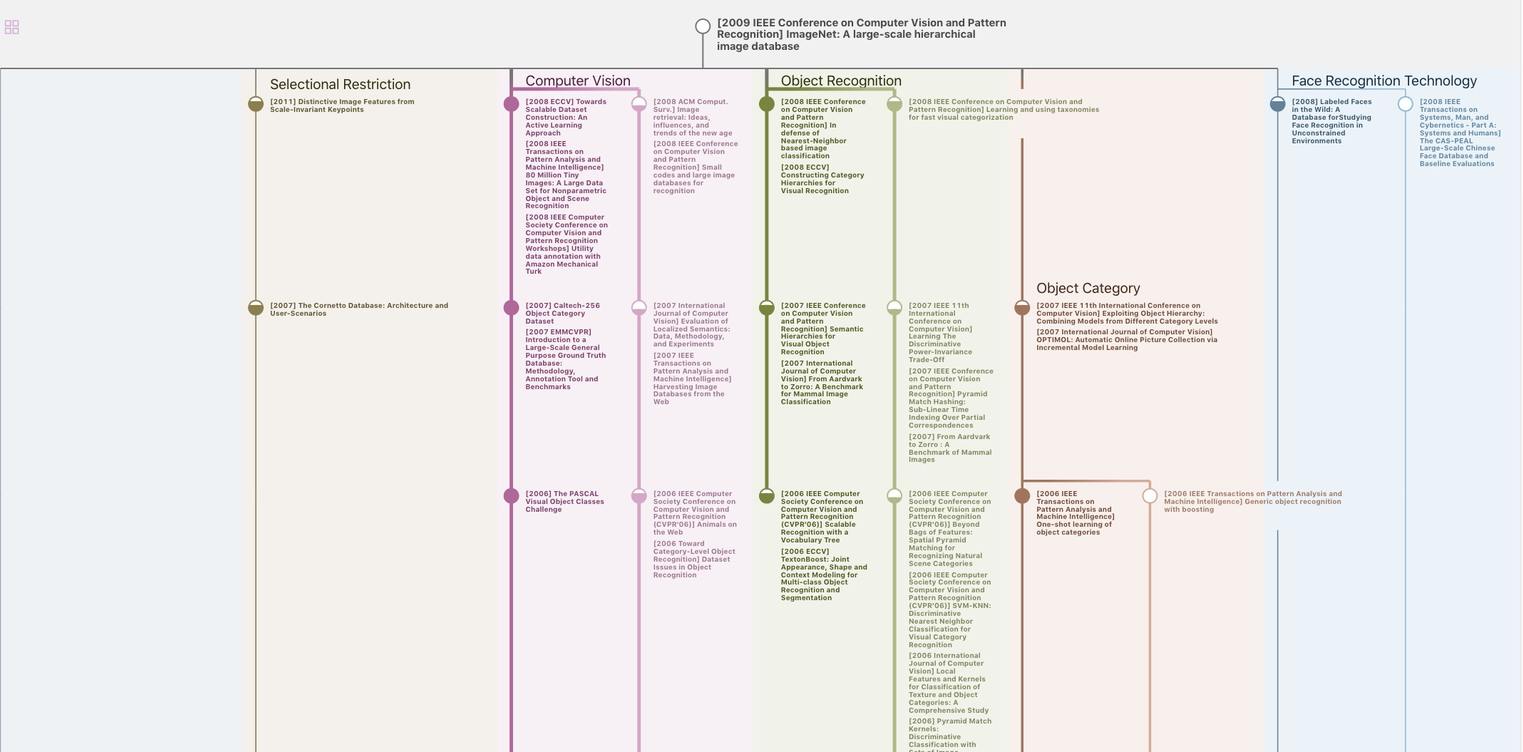
生成溯源树,研究论文发展脉络
Chat Paper
正在生成论文摘要