Towards Next-Generation Intelligent Assistants Leveraging LLM Techniques
PROCEEDINGS OF THE 29TH ACM SIGKDD CONFERENCE ON KNOWLEDGE DISCOVERY AND DATA MINING, KDD 2023(2023)
摘要
Virtual Intelligent Assistants take user requests in the voice form, perform actions such as setting an alarm, turning on a light, and answering a question, and provide answers or confirmations in the voice form or through other channels such as a screen. Assistants have become prevalent in the past decade, and users have been taking services from assistants like Amazon Alexa, Apple Siri, Google Assistant, and Microsoft Cortana. The emergence of AR/VR devices raised many new challenges for building intelligent assistants. The unique requirements have inspired new research directions such as (a) understanding users' situated multi-modal contexts (e.g. vision, sensor signals) as well as language-oriented conversational contexts, (b) personalizing the assistant services by grounding interactions on growing public and personal knowledge graphs and online search engines, and (c) on-device model inference and training techniques that satisfy strict resource and privacy constraints. In this tutorial, we will provide an in-depth walk-through of techniques in the afore-mentioned areas in the recent literature. We aim to introduce techniques for researchers and practitioners who are building intelligent assistants, and inspire research that will bring us one step closer to realizing the dream of building an all-day accompanying assistant. Additionally, we will highlight the significant role that Large Language Models (LLMs) play in enhancing these strategies, underscoring their potential to reshape the future landscape of intelligent assistance.
更多查看译文
关键词
conversational AI,large language models,multi-modal conversation,knowledge grounding,personalization,federated learning
AI 理解论文
溯源树
样例
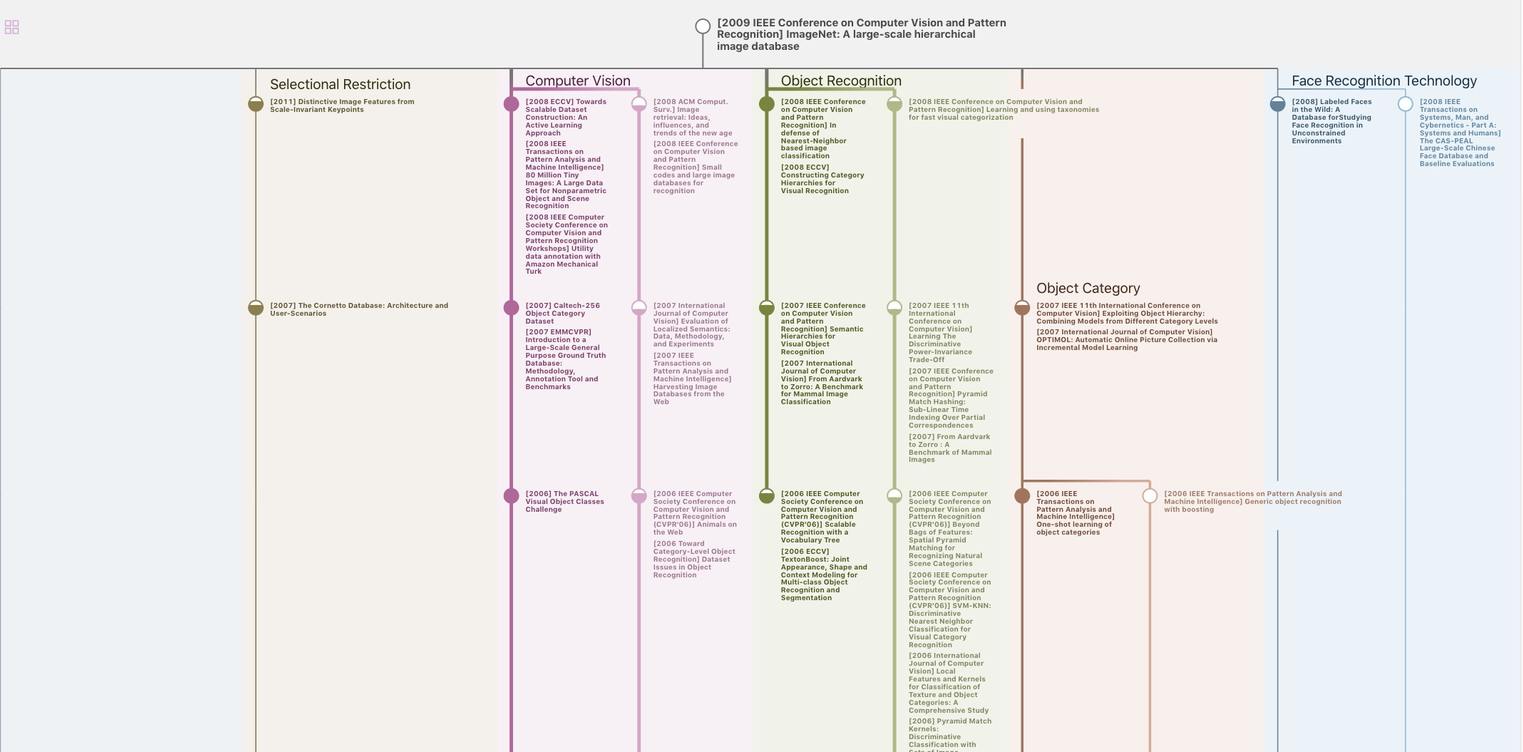
生成溯源树,研究论文发展脉络
Chat Paper
正在生成论文摘要