KALE: Using a K-Sparse Projector for Lexical Expansion
PROCEEDINGS OF THE 2023 ACM SIGIR INTERNATIONAL CONFERENCE ON THE THEORY OF INFORMATION RETRIEVAL, ICTIR 2023(2023)
Abstract
Recent research has proposed retrieval approaches based on sparse representations and inverted indexes, with terms produced by neural language models and leveraging the advantages from both neural retrieval and lexical matching. This paper proposes KALE, a new lightweight method of this family that uses a small model with a k-sparse projector to convert dense representations into a sparse set of entries from a latent vocabulary. The KALE vocabulary captures semantic concepts than perform well when used in isolation, and perform better when extending the original lexical vocabulary, this way improving first-stage retrieval accuracy. Experiments with the MSMARCOv1 passage retrieval dataset, the TREC Deep Learning dataset, and BEIR datasets, examined the effectiveness of KALE under varying conditions. Results show that the KALE terms can replace the original lexical vocabulary, with gains in accuracy and efficiency. Combining KALE with the original lexical vocabulary, or with other learned terms, can further improve retrieval accuracy with only a modest increase in computational cost.
MoreTranslated text
Key words
Neural Information Retrieval,Learned Sparse Representations,Efficiency in Neural Retrieval
AI Read Science
Must-Reading Tree
Example
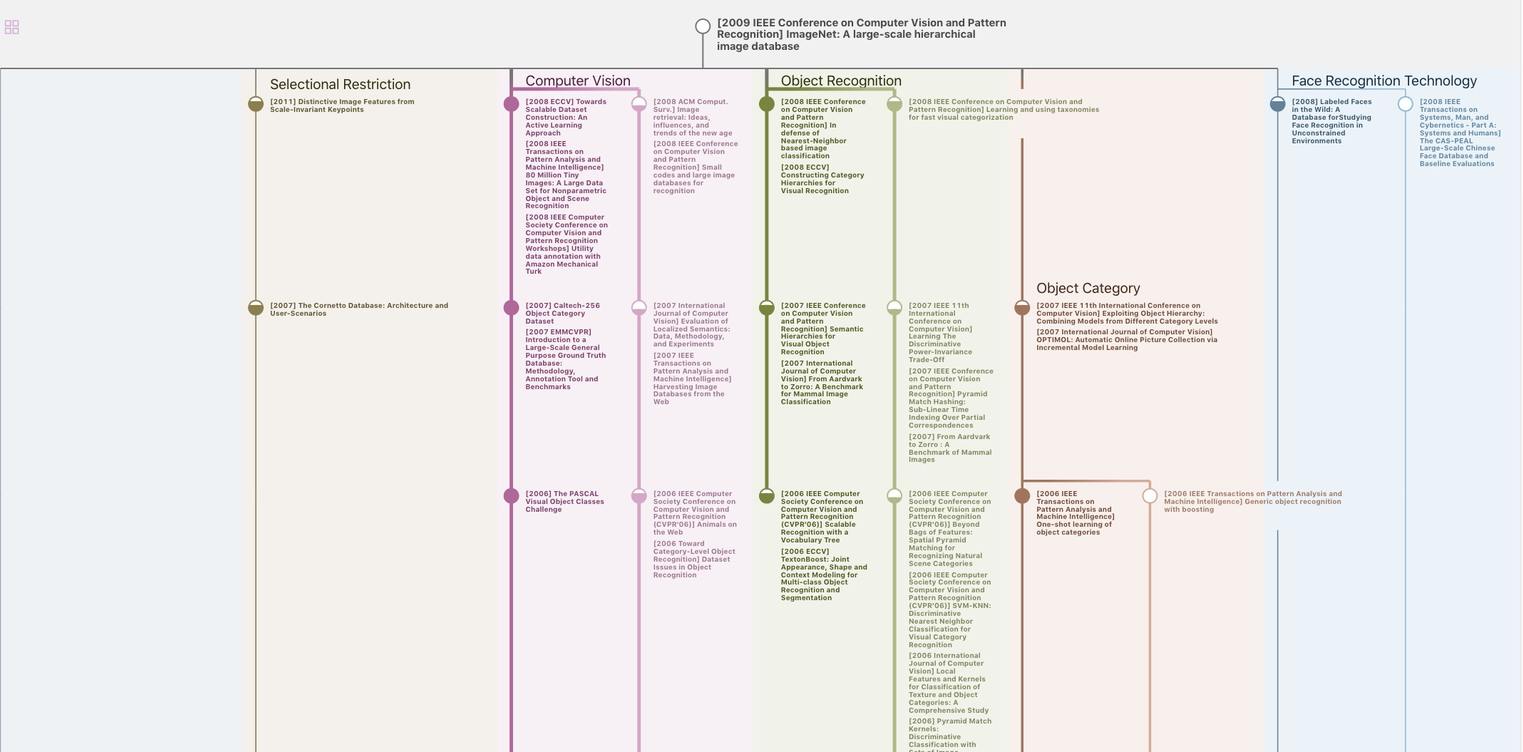
Generate MRT to find the research sequence of this paper
Chat Paper
Summary is being generated by the instructions you defined