A Theoretical Analysis of Out-of-Distribution Detection in Multi-Label Classification.
International Conference on the Theory of Information Retrieval(2023)
摘要
The ability to detect out-of-distribution (OOD) inputs is essential for safely deploying machine learning models in an open world. Most existing research on OOD detection, and more generally uncertainty quantification, has focused on multi-class classification. However, for many information retrieval (IR) applications, the classification of documents or images is by nature not multi-class but multi-label. This paper presents a pure theoretical analysis of the under-explored problem of OOD detection in multi-label classification using deep neural networks. First, we examine main existing approaches such as MSP (proposed in ICLR-2017) and MaxLogit (proposed in ICML-2022), and summarize them as different combinations of label-wise scoring and aggregation functions. Some existing methods are shown to be equivalent. Then, we prove that JointEnergy (proposed in NeurIPS-2021) is indeed the optimal probabilistic solution when the class labels are conditionally independent with each other for any given data sample. This provides a more rigorous explanation for the effectiveness of JointEnergy than the original joint-likelihood interpretation, and also reveals its reliance upon the assumption of label independence rather than the exploitation of label relationships as previously thought. Finally, we discuss potential future research directions in this area.
更多查看译文
关键词
multi-label classification,out-of-distribution detection,uncertainty quantification
AI 理解论文
溯源树
样例
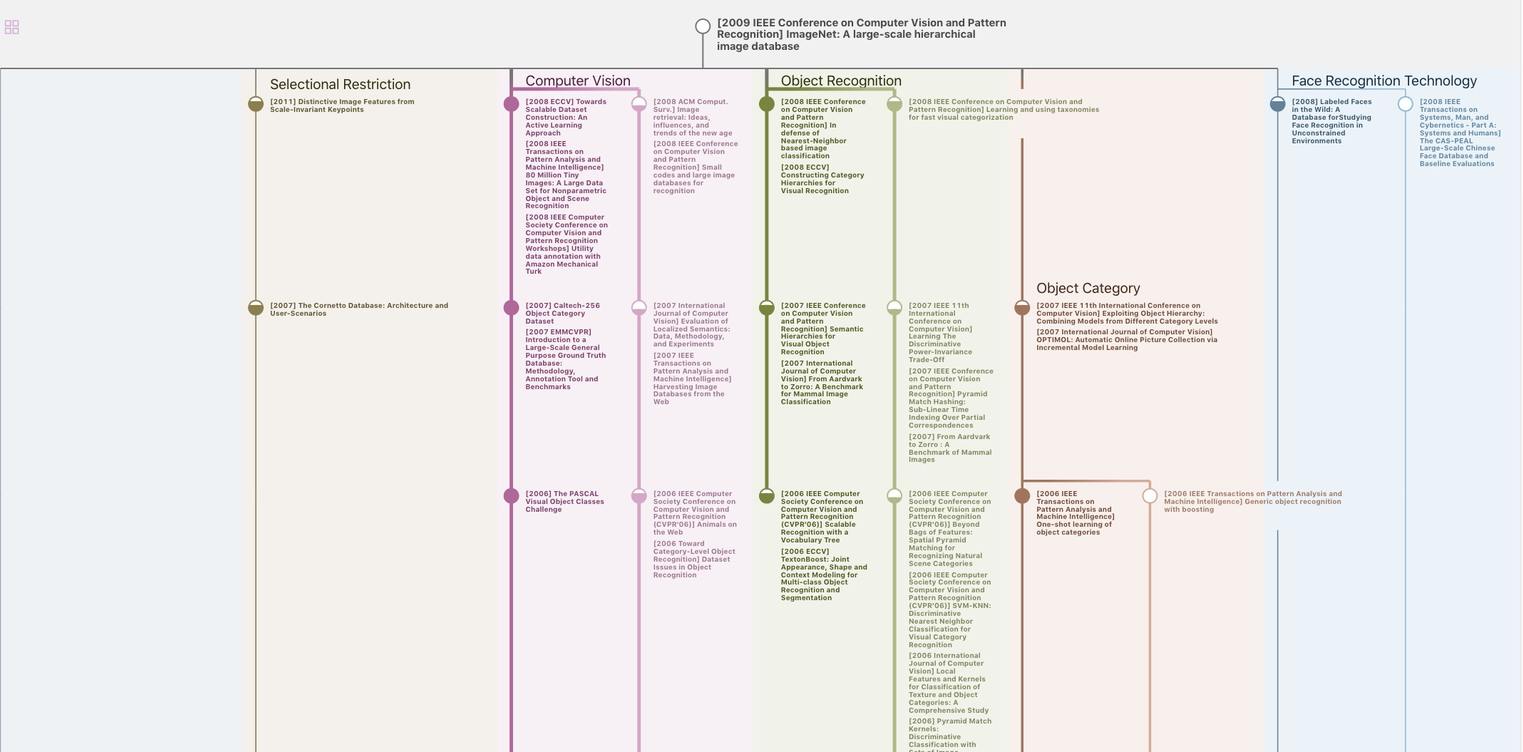
生成溯源树,研究论文发展脉络
Chat Paper
正在生成论文摘要