Data Augmentation for Tensor Completion via Embedding Gradient Tracking.
CSCloud/EdgeCom(2023)
摘要
How can we enhance the performance of neural tensor completion models for sparse data recovery? The task of tensor completion is crucial for network monitoring since it is the basis of network operation and management. Neural tensor completion models can exploit the multi-dimensional structure of tensors to recover missing entries in partially observed tensors. However, they can produce inaccurate estimations as real-world tensors are very sparse. These models tend to overfit a small amount of data, leading to limited accuracy on recovered data. In this paper, we propose LightTracker, a general data augmentation framework that enhances the prediction accuracy of neural tensor completion models. Specifically, LightTracker first trains a neural tensor completion model and tracks the gradient from the view of embeddings. After training, we use the tracked entity gradient to determine the importance of unknown tensor entries. Then, we aggregate the entries’ importance to calculate the importance of each entity. Finally, LightTracker creates an augmented tensor by mixing observed data and predicted entries with the highest importance. Experiments conducted on two network traffic datasets show that LightTracker effectively enhanced the imputation accuracy of different tensor completion baselines, showing its generality and effectiveness.
更多查看译文
关键词
data augmentation,embeddings,influence function,network traffic,tensor completion
AI 理解论文
溯源树
样例
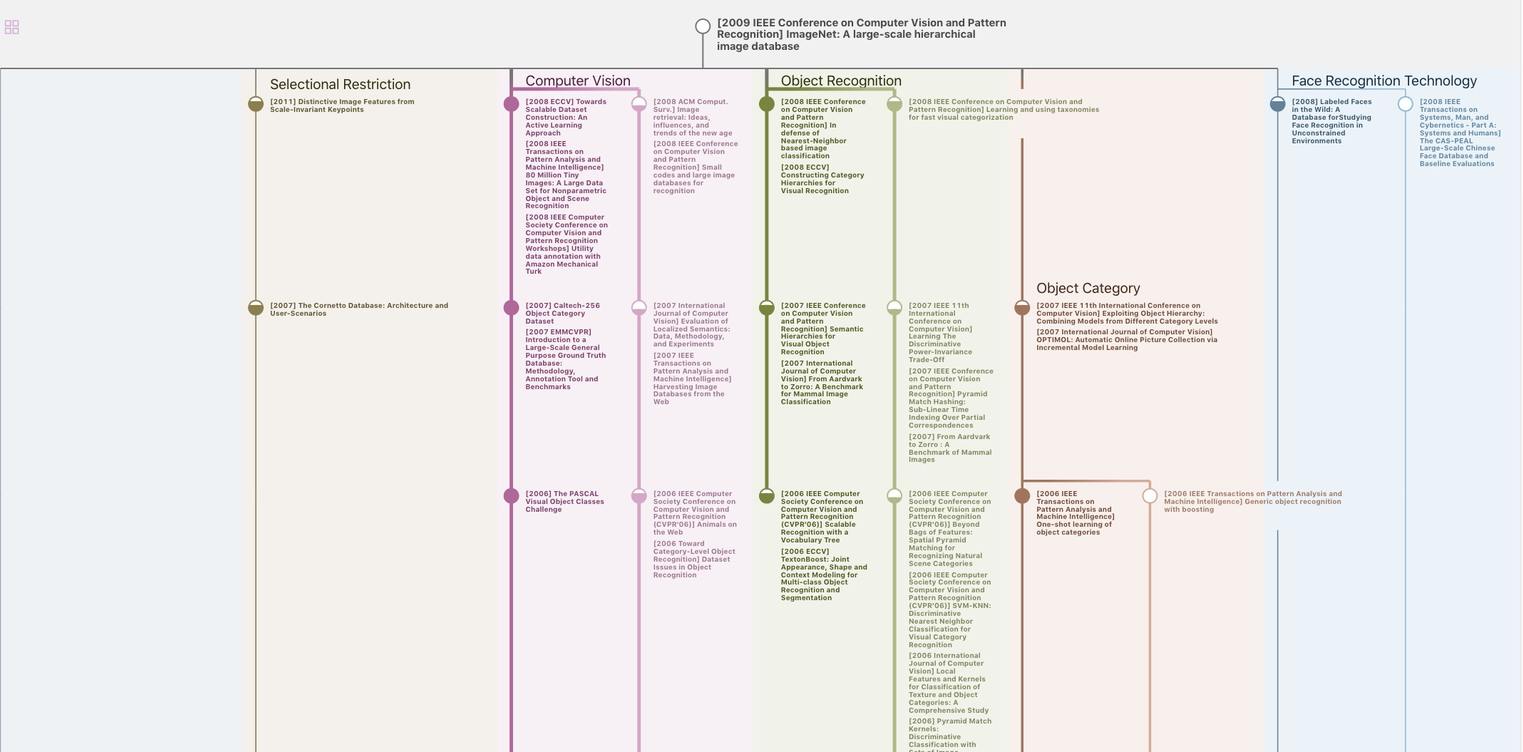
生成溯源树,研究论文发展脉络
Chat Paper
正在生成论文摘要