Multivariate Time Series Anomaly Detection with Improved Encoder-Decoder Based Model.
CSCloud/EdgeCom(2023)
Key words
anomaly detection,multivariate time series,graph structure,cluster embedding,attention mechanism
AI Read Science
Must-Reading Tree
Example
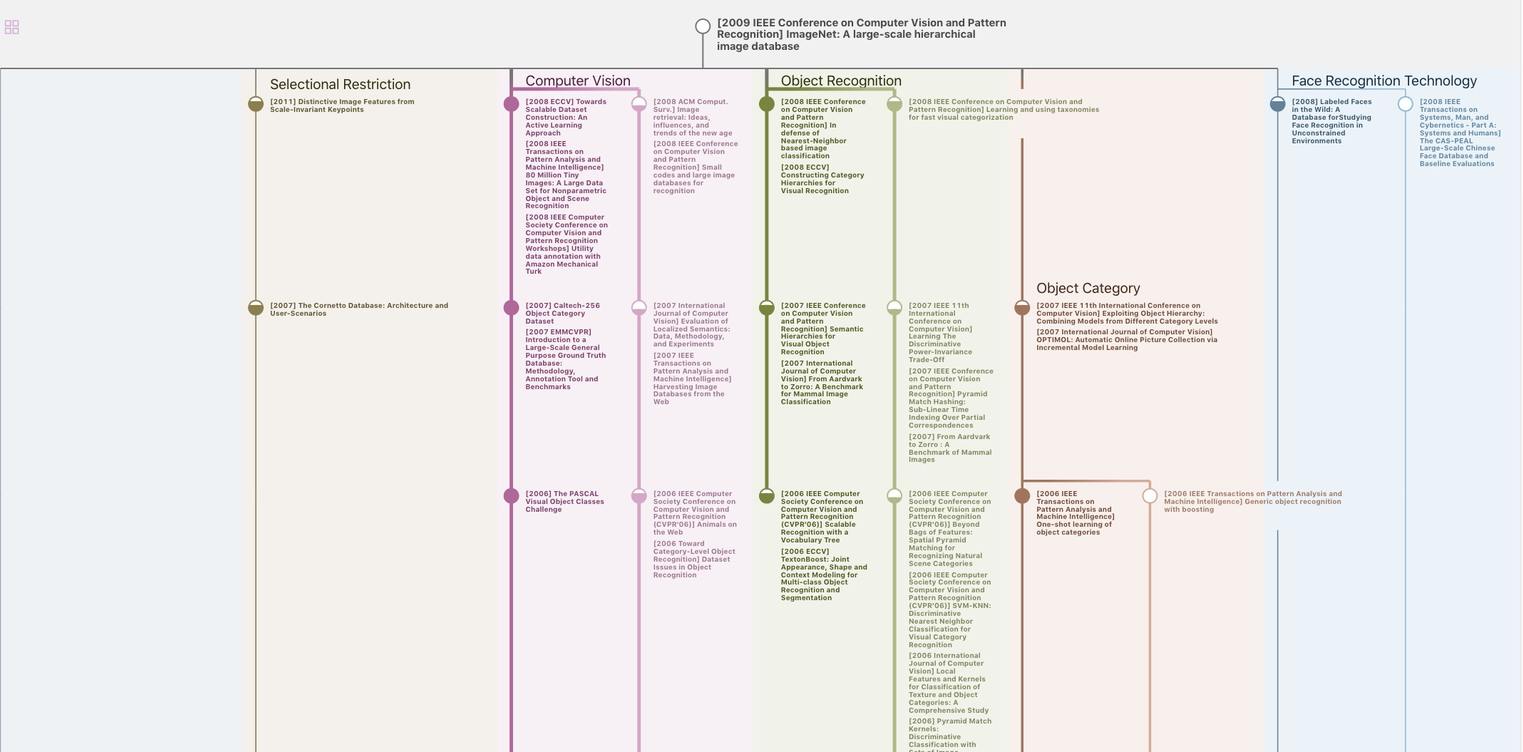
Generate MRT to find the research sequence of this paper
Chat Paper
Summary is being generated by the instructions you defined