MixOT: Graph Representation Learning based on Mix-order Sampling and Transport Aggregator for Social Networks.
SmartWorld/UIC/ScalCom/DigitalTwin/PriComp/Meta(2022)
摘要
Graph Neural Networks (GNNs), one of the highly regarded graph representation learning methods, have achieved excellent performance in social networks. Graph convolution performs neighborhood aggregation, which is one of the most important graph operations. However, one layer in these methods considers only the nearest neighbors, and most existing GNNs are inherently limited by over-smoothing when going deeper into larger receptive domains. In this paper, we propose MixOT address this problem, which follows the idea of decoupling representation transformation and propagation in graph convolution operations. In MixOT, we first design an unbiased node sampling probability to precompute the mixed-order matrix. Then, we use top-k truncation to sample the neighborhood information of each order, thus implementing a sampler that avoids ‘‘neighbor explosion Based on this, we develop a transport aggregation method that efficiently measures the importance of neighbors of each order using optimal transport theory. Extensive experiments on graph benchmark datasets show that MixOT outperforms state-of-the-art GNN baselines in node classification and effectively alleviates the over-smoothing problem.
更多查看译文
关键词
Graph neural networks,Node sampling,Optimal transport,Social networks
AI 理解论文
溯源树
样例
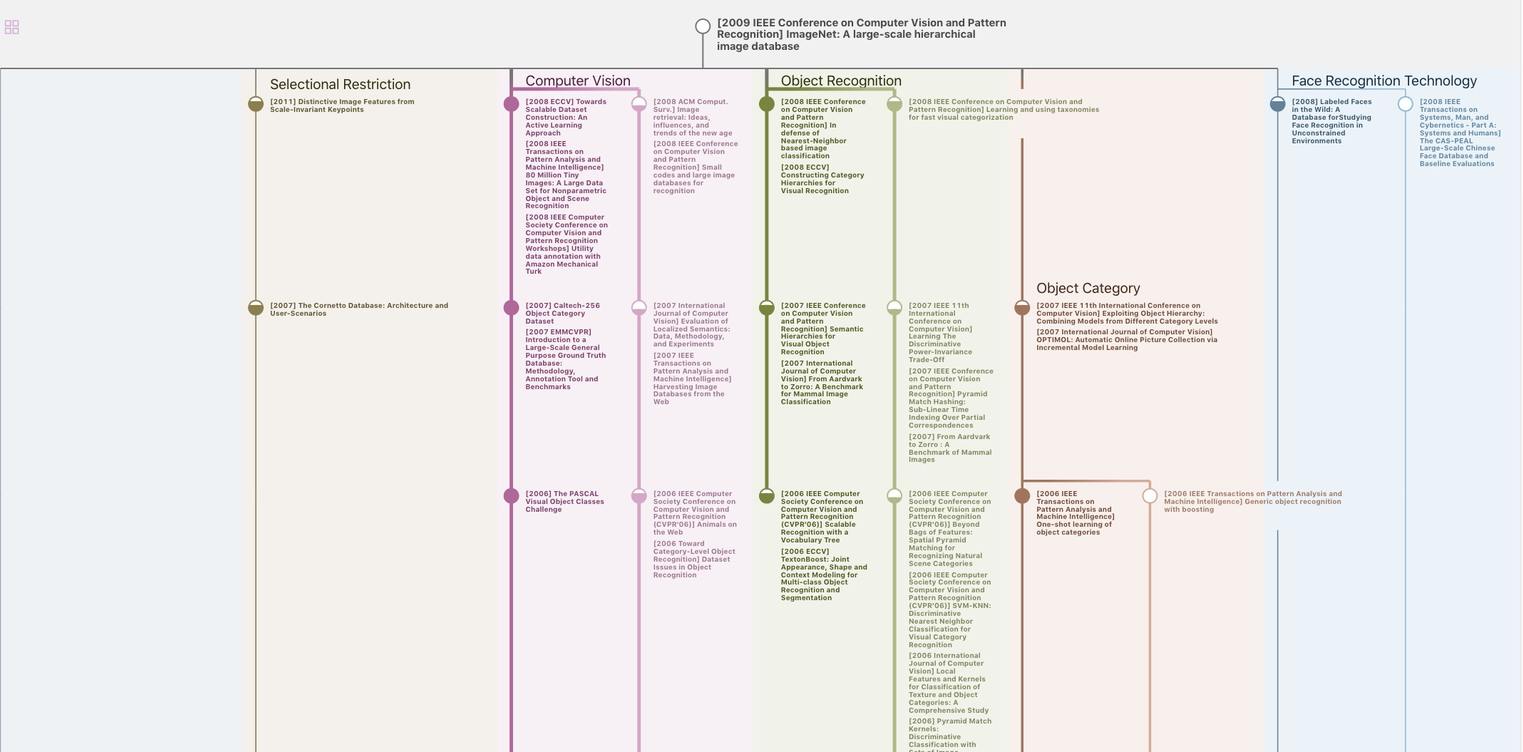
生成溯源树,研究论文发展脉络
Chat Paper
正在生成论文摘要