Robust Spatio-Temporal Trajectory Modeling Based on Auto-Gated Recurrent Unit.
SmartWorld/UIC/ScalCom/DigitalTwin/PriComp/Meta(2022)
摘要
With the huge amount of crowd mobility data generated by the explosion of mobile devices, deep neural networks (DNNs) are applied to trajectory data mining and modeling, which make great progresses in those scenarios. However, recent studies have demonstrated that DNNs are highly vulnerable to adversarial examples which are crafted by adding subtle, imperceptible noise to normal examples, and leading to the wrong prediction with high confidence. To improve the robustness of modeling spatiotemporal trajectories via DNNs, we propose a collaborative learning model named “Auto-GRU”, which consists of an autoencoder-based self-representation network (SRN) for robust trajectory feature learning and gated recurrent unit (GRU)-based classification network which shares information with SRN for collaborative learning and strictly defending adversarial examples. Our proposed method performs well in defending both white and black box attacks, especially in black-box attacks, where the performance outperforms state-of-the-art methods. Moreover, extensive experiments on Geolife and Beijing taxi traces datasets demonstrate that the proposed model can improve the robustness against adversarial examples without a significant performance penalty on clean examples.
更多查看译文
关键词
gated recurrent unit,spatio-temporal trajectory,adversarial examples
AI 理解论文
溯源树
样例
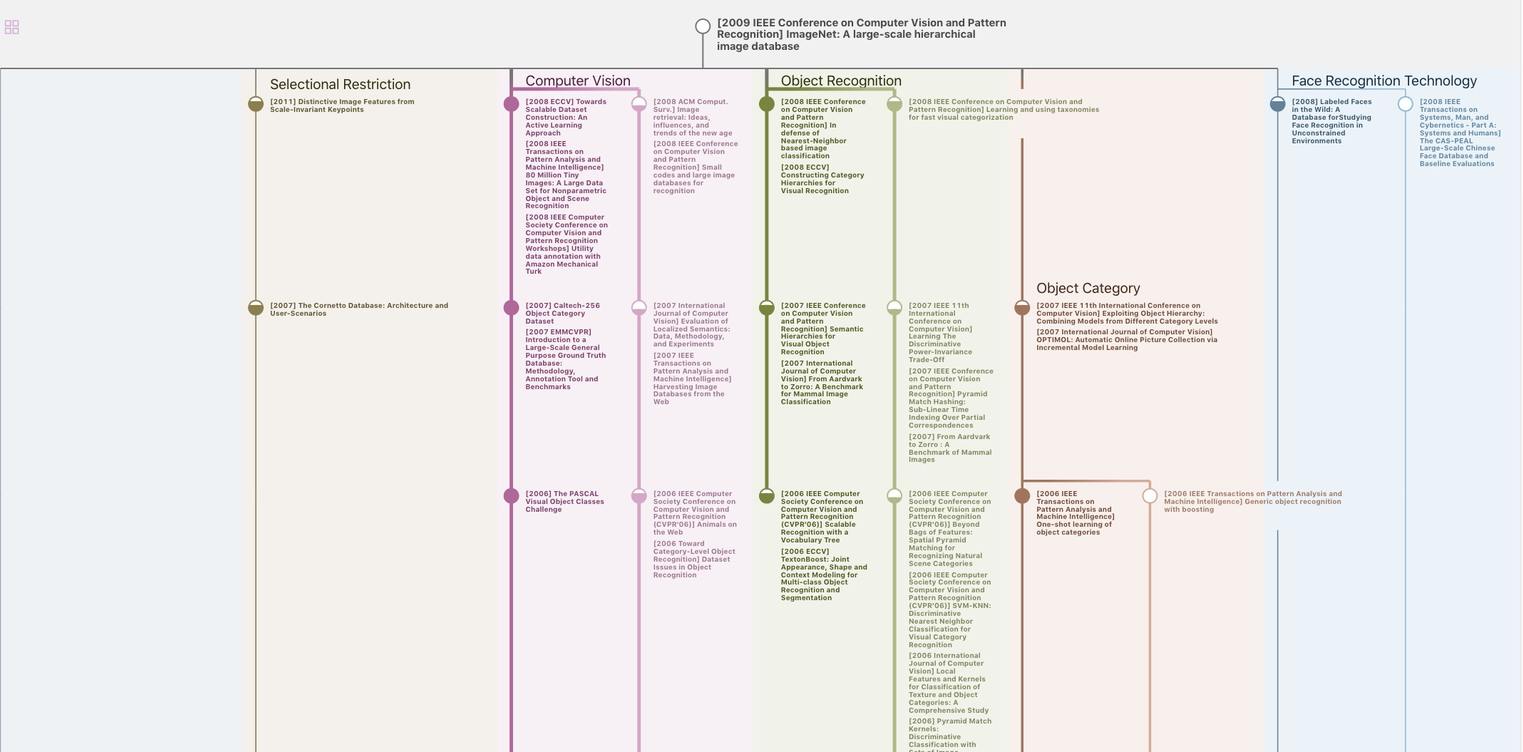
生成溯源树,研究论文发展脉络
Chat Paper
正在生成论文摘要