Latent Dynamic Token Vision Transformer for Pedestrian Attribute Recognition.
SmartWorld/UIC/ScalCom/DigitalTwin/PriComp/Meta(2022)
摘要
Pedestrian attribute recognition(PAR), as a subarea of multi-label classification, requires models to qualify multiple class-specific features learning ability. However, existing multi-label classification models, such as those based on CNN or Transformer, tend to form a single class-specific attention map for all labels. In order to further improve the model’s ability to capture multiple class-specific attention regions, we propose a Latent Dynamic Token Vision Transformer, termed LDT-ViT, which constructs a sequential LDT attention module to generate multiple latent tokens dynamically. Compared with the single class-token, the multiple latent tokens produced by the LDT module can learn more class-specific attention regions corresponding to different attributes of a pedestrian. Moreover, we also design a parallel multi-stage voting mechanism to aggregate hierarchical ignored feature information from the intermediate layers. The voting mechanism can produce the maximum responses across different attention layers to take advantage of complementary intermediate features, which are more suitable for multi-label tasks. Comprehensive experiments show that the proposed LDT-ViT model achieves state-of-the-art performance on PETA and PA-100K pedestrian attribute datasets.
更多查看译文
关键词
pedestrian attribute recognition,human-computer interaction,vision transformer,latent dynamic tokens,hierarchical feature aggregation
AI 理解论文
溯源树
样例
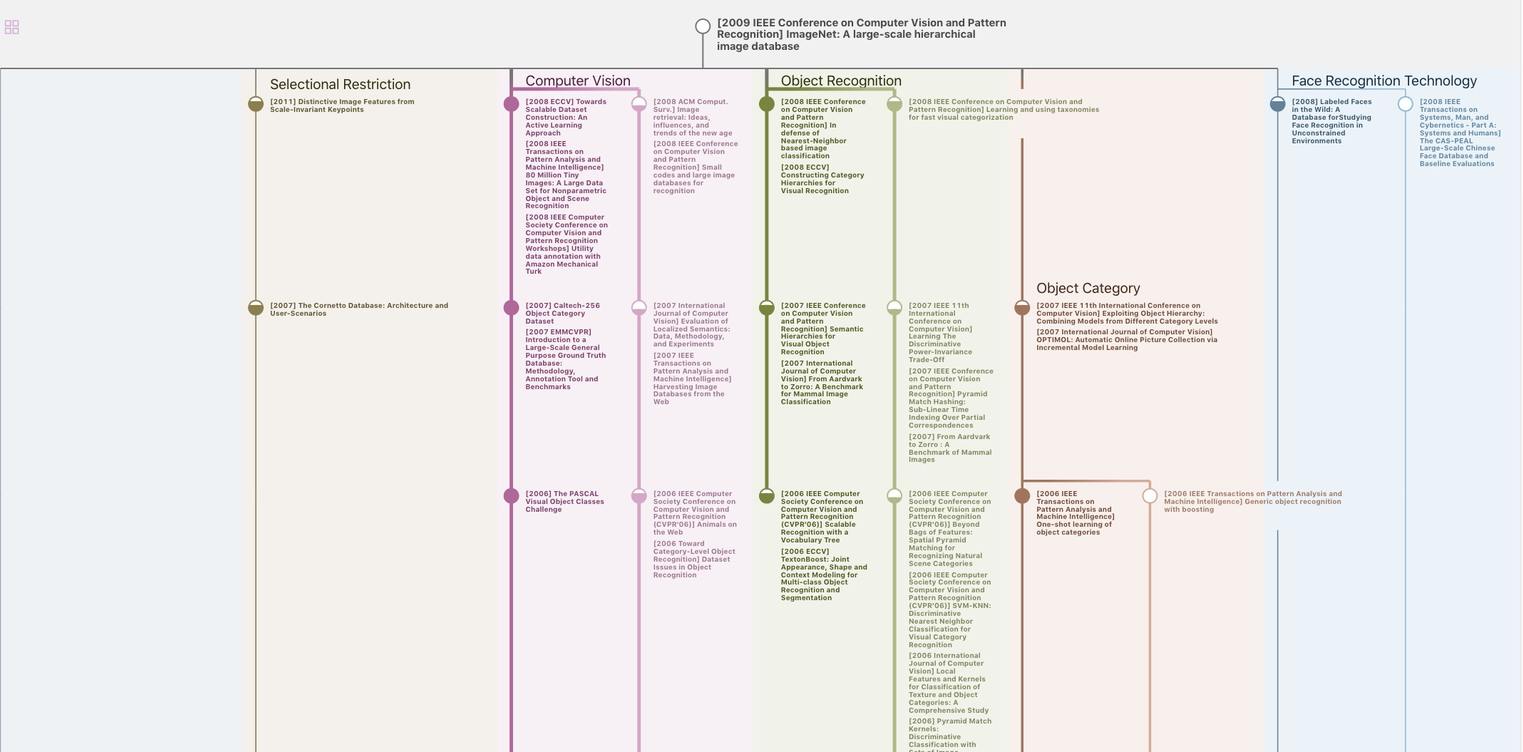
生成溯源树,研究论文发展脉络
Chat Paper
正在生成论文摘要