Attention-Based Knowledge-aware Multi-Interest Intelligent Model for Sequential Recommendation.
SmartWorld/UIC/ScalCom/DigitalTwin/PriComp/Meta(2022)
摘要
As a fundamental means of intelligent service, recommender systems have shown a trend of diversification. Sequential recommendation, a class of time-aware methods, aims to predict the user’s next click. Multi-interest representation is a hot topic in recommender systems, but the research on how to apply multi-interest into sequences is still in the minority. And many methods will introduce knowledge graph to improve the representation of items, but how to consider both knowledge graph and user’s multi-interest in sequential recommendation has become a temporary blank. In this paper, we propose an attentive sequential recommendation with knowledge-aware multi-interest module (AKAMI). Items are embedded from the sequence’s structure and user’s interests. Specifically, we represent items by stacking self-attention layers to mine the internal correlation of the sequence. Meanwhile, we segment the sequence via the common neighbors in the knowledge graph and cast the user’s multi-interest based on the aggregation of subsequences. Multi-interests are integrated into item representation by the gating network adaptively. Comprehensive experiments on two real-world datasets show that the proposed model performs better than various representative methods under different evaluation metrics. Additionally, we also visually explain the model through a case study.
更多查看译文
关键词
Recommender Systems,Attention Mechanism,Sequential Recommendation,Multi-Interest,Knowledge Graph
AI 理解论文
溯源树
样例
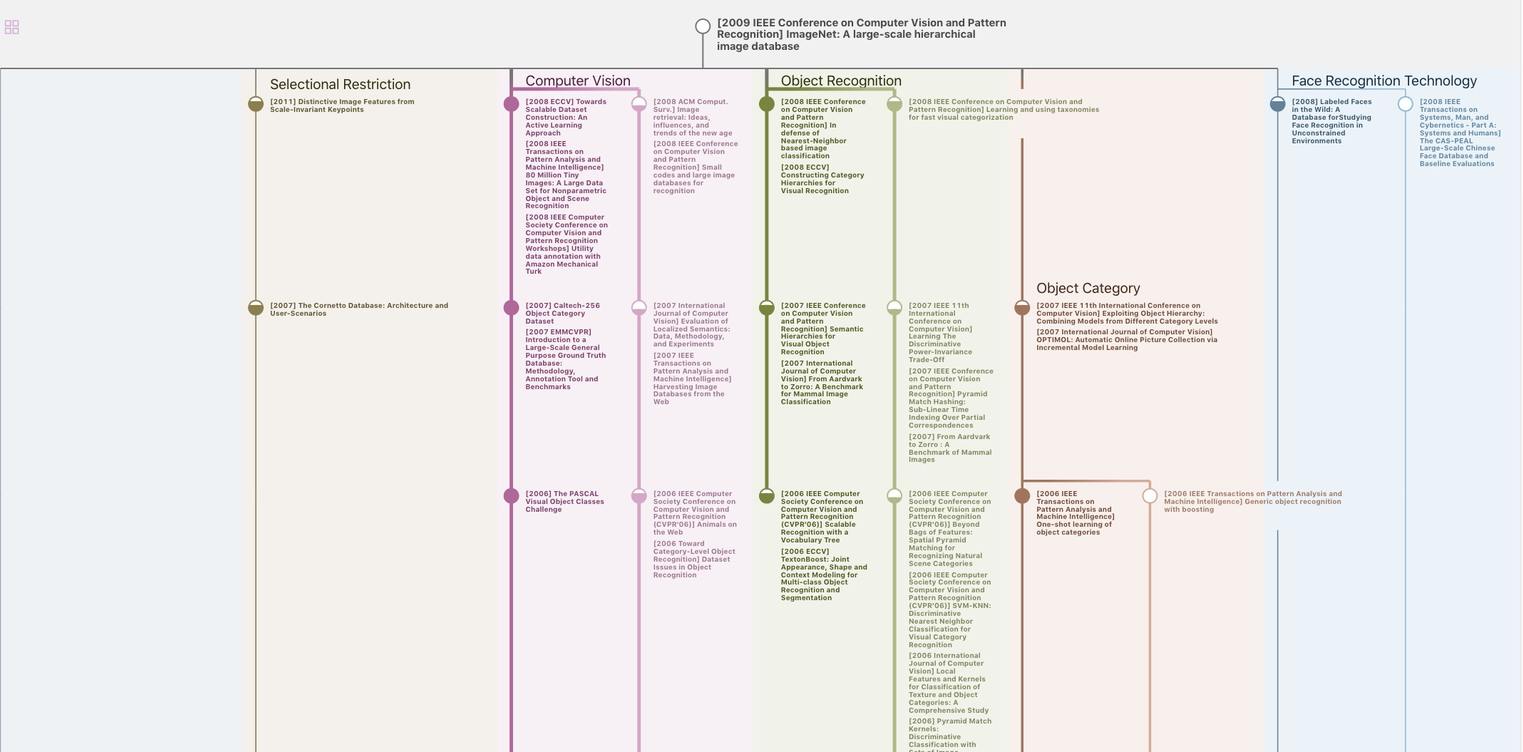
生成溯源树,研究论文发展脉络
Chat Paper
正在生成论文摘要