Geometric-Aware Calibration Mechanism for Self-Supervised Depth Estimation.
SmartWorld/UIC/ScalCom/DigitalTwin/PriComp/Meta(2022)
摘要
Due to the lack of ground-truth, nearly all self-supervised depth estimation methods use reprojection errors as the main supervised signal. We find that it fails to train the network with pixels reprojection errors in some special regions, such as textureless, boundary, occlusion and motion regions. The reason is that the reprojection error-based loss produces an unexpected deviation because of the irregular illumination change and the wrong reprojection matching of pixels. As a result, we propose a novel geometric-inspired calibration mechanism (GCM) that contains two parts to rectify this problem. The first part is a supervised signal based on the geometric distance, which is used to improve the performance of the network in textureless and boundary regions. The second is a rectify signal to deal with the deviation of the reprojection error. Then, we implement GCM by the Cycle Consistency Loss (CCL) and the Matching Filter. The CCL is designed to optimize the performance of the network in depth estimation, and the Matching Filter, based on CCL and reprojection errors, aims to rectify the deviation of reprojection errors. The final experiments demonstrate the effectiveness and applicability of our method compared with other methods on the KITTI dataset and get the SOTA result.
更多查看译文
关键词
Self-Supervised,Geometric Consistency,Depth Estimation
AI 理解论文
溯源树
样例
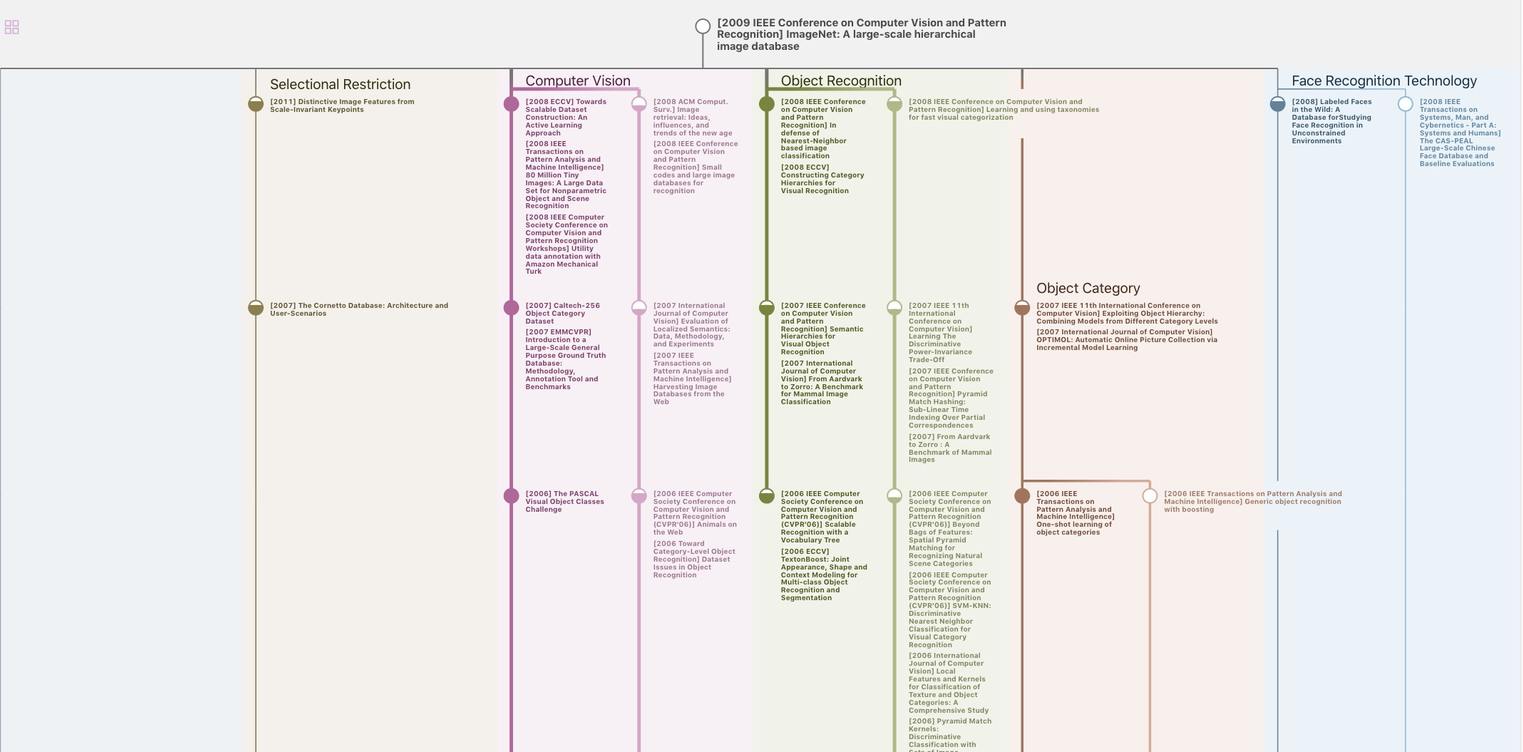
生成溯源树,研究论文发展脉络
Chat Paper
正在生成论文摘要