Deep Q-Learning Enabled Energy-Efficient Resource Allocation and Task Deployment for MEC.
SmartWorld/UIC/ScalCom/DigitalTwin/PriComp/Meta(2022)
摘要
Aiming at improving resource utilization and network capacity of Mobile Edge Computing (MEC) system, we study the problem of computation offloading, resource allocation and task deployment in MEC system consisting of multiple Mobile Devices (MDs) and multiple Base Stations (BSs) integrating with a MEC server. Each MD can execute its partial task locally and accomplish its remaining task remotely in a MEC server. The computation offloading task selects one from multiple MEC server to complete the task. The objective is to achieve the optimal scheduling scheme that can minimize the overall energy consumption of all the MDs and MEC server, subject to the constraints of task deployment strategy, communication and computing resources, CPU frequency, transmission power and latency. The optimal scheme is designed with respect to three decision parameters: computation offloading decision, resource allocation strategy and task deployment strategy. We propose a deep Q-learning enabled resource allocation and task deployment (DQRD) algorithm to solve the formulated integer-mixed non-convex optimization problem. We obtain the optimal task deployment strategy by utilizing neural network, and achieve the optimal resource allocation strategy by using convex optimization technology with given task deployment strategy. Simulation results demonstrate that the multi-MD multi-server MEC system makes significant achievements in energy saving. Compared with other baselines, our proposed algorithm not only achieves the suboptimal solution, but also reduces the execution latency.
更多查看译文
关键词
Deep Q-Learning,Resource Allocation,Task Deployment,Computation Offloading,Convex Optimization
AI 理解论文
溯源树
样例
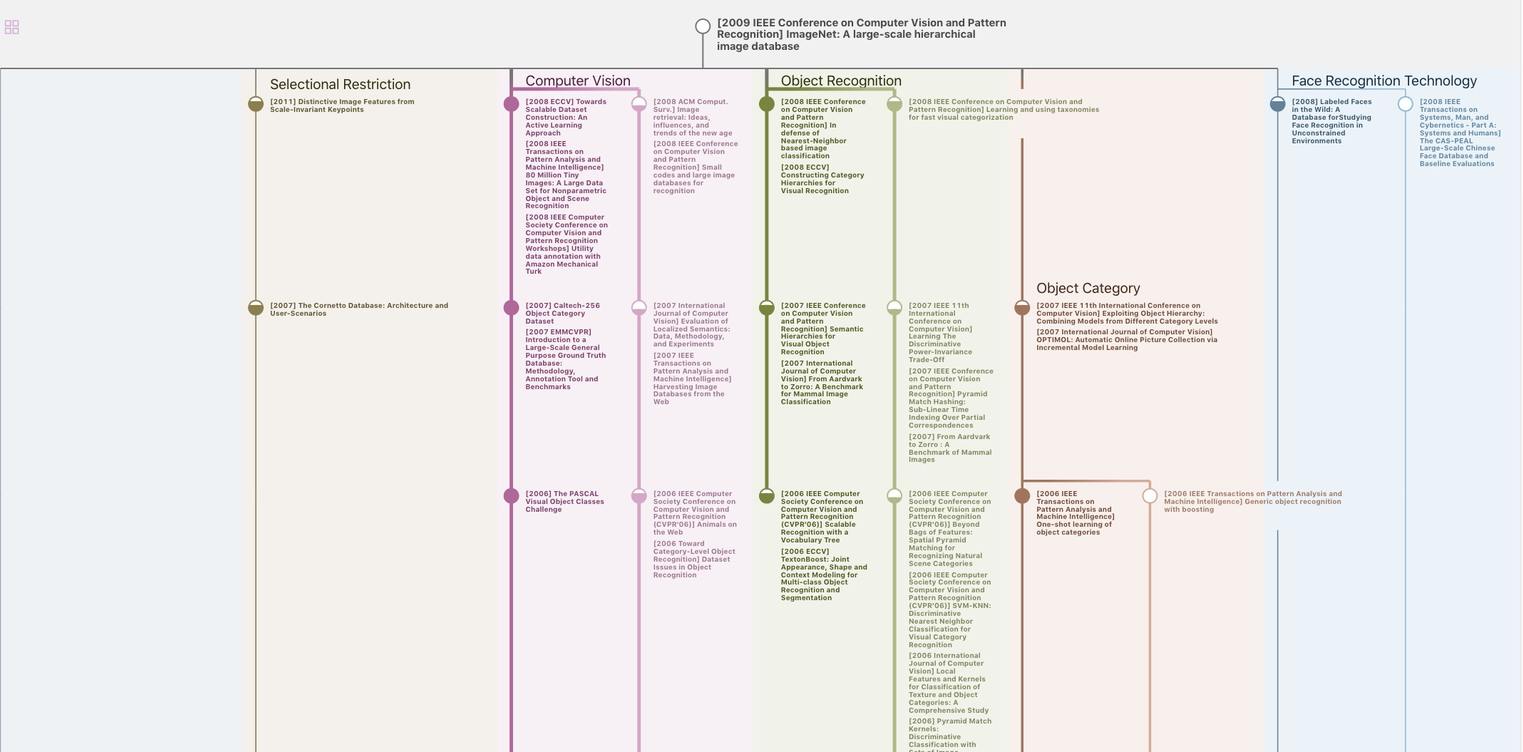
生成溯源树,研究论文发展脉络
Chat Paper
正在生成论文摘要