An Approach for Traffic Flow Prediction Combining 3D Convolution and Attention Mechanism
SmartWorld/UIC/ScalCom/DigitalTwin/PriComp/Meta(2022)
摘要
Highly reliable traffic flow forecasting is essential to improve the management efficiency of intelligent transportation systems. However, traffic flow data contains complex spatio-temporal relationships, while this complex relationship is an organic whole. Therefore, improving traffic flow prediction performance is a challenging task. Some existing works have demonstrated the existence of spatio-temporal correlation in traffic flow data, but not all the features in the data are fully mined, mainly because the spatio-temporal diversity of traffic flow data is not taken into account, and the modeling approach of multi-network structure is used for separate modeling, which destroys the overall principle of traffic flow and eventually makes it difficult to achieve the expected prediction effect of the model. Within this paper, we propose a deep learning model ST-3DANet with a single network structure to model the spatio-temporal raster data features of traffic flow to improve the prediction effect.ST-3DANet combines 3D convolution and residual structure, and introduces an attention mechanism to automatically capture the spatio-temporal correlation of traffic flow data. Specifically, the spatio-temporal data for traffic flow prediction is taken as a whole, and the spatio-temporal diversity in traffic data is automatically mined by 3D convolution, and the model’s ability to discover spatiotemporal diversity and long-range spatial correlation is enhanced by using the attention mechanism. Experiments were conducted on a real dataset in New York, and the results showed that our model outperformed other benchmark models in terms of predictive effectiveness.
更多查看译文
关键词
Traffic flow prediction,spatio-temporal correlations,diversity,temporal indivisibility,3D convolution,attention mechanism
AI 理解论文
溯源树
样例
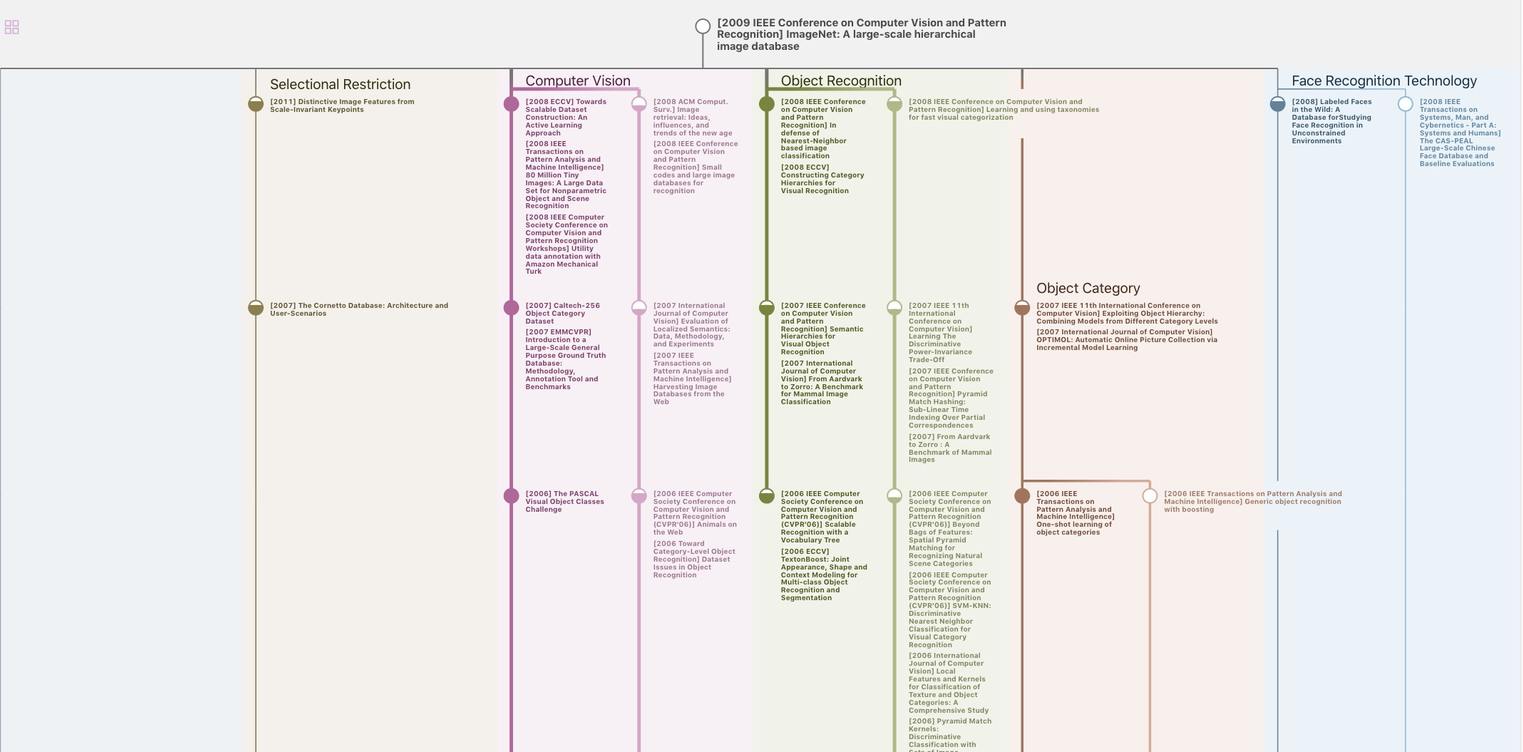
生成溯源树,研究论文发展脉络
Chat Paper
正在生成论文摘要