C-ISTA: Iterative Shrinkage-Thresholding Algorithm for Sparse Covariance Matrix Estimation.
SSP(2023)
摘要
Covariance matrix estimation is a fundamental task in many fields related to data analysis. As the dimension of the covariance matrix becomes large, it is desirable to obtain a sparse estimator and an efficient algorithm to compute it. In this paper, we consider the covariance matrix estimation problem by minimizing a Gaussian negative log-likelihood loss function with an l(1) penalty, which is a constrained non-convex optimization problem. We propose to solve the covariance estimator via a simple iterative shrinkage-thresholding algorithm (C-ISTA) with provable convergence. Numerical simulations with comparison to the benchmark methods demonstrate the computational efficiency and good estimation performance of C-ISTA.
更多查看译文
关键词
Covariance matrix, sparsity, iterative shrinkage-thresholding algorithm, maximum likelihood, non-convex optimization
AI 理解论文
溯源树
样例
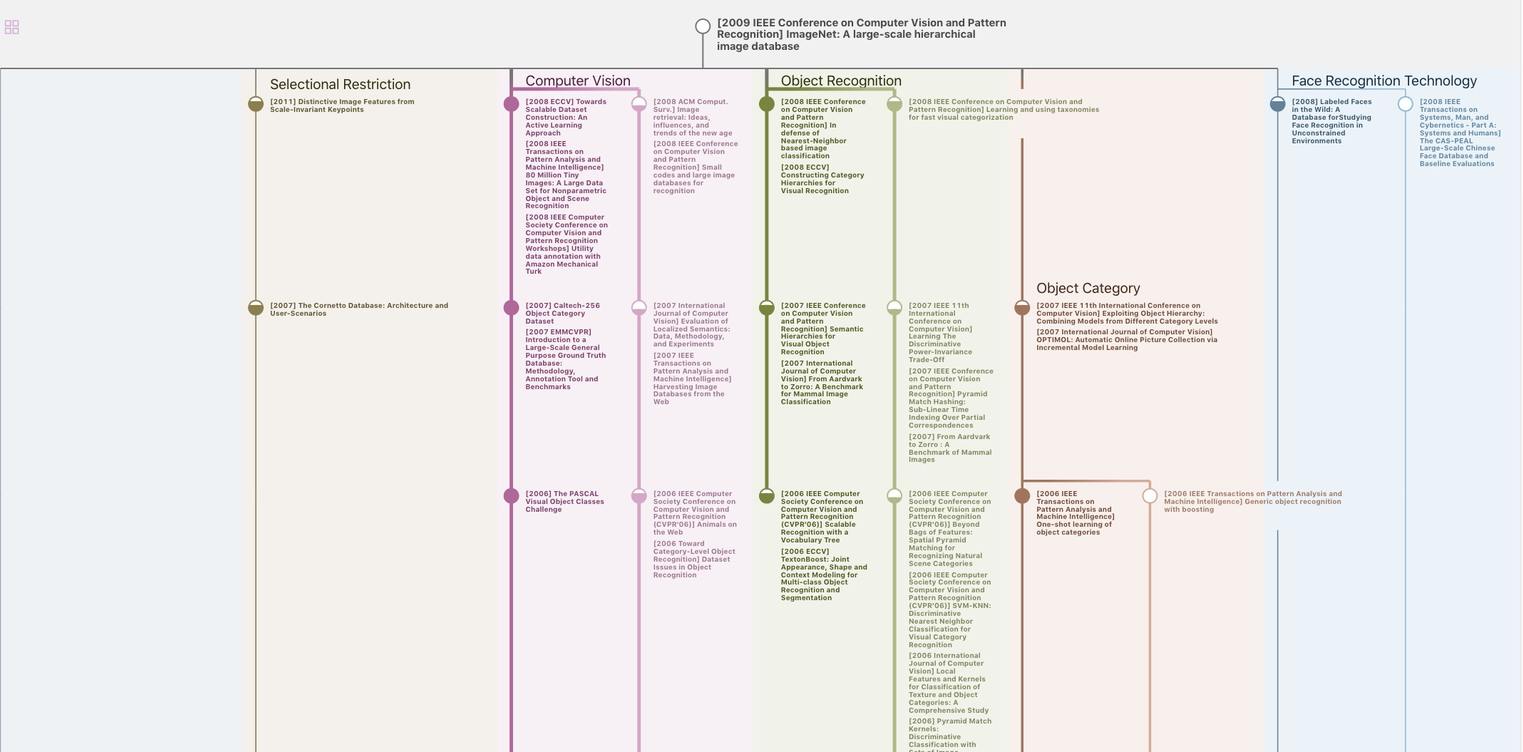
生成溯源树,研究论文发展脉络
Chat Paper
正在生成论文摘要