Enhancing Retail Checkout through Video Inpainting, YOLOv8 Detection, and DeepSort Tracking.
CVPR Workshops(2023)
摘要
The retail industry has witnessed a remarkable upswing in the utilization of cutting-edge artificial intelligence and computer vision techniques. Among the prominent challenges in this domain is the development of an automated checkout system that can address the multifaceted issues that arise in real-world checkout scenarios, including object occlusion, motion blur, and similarity in scanned items. In this paper, we propose a sophisticated deep learning-based framework that can effectively recognize, localize, track, and count products as they traverse in front of a camera. Our approach, which we call RetailCounter, is founded on a detect-then-track paradigm, wherein we apply tracking on the bounding box of the detected objects. Furthermore, we have incorporated an automatic identification of the detection region of interest (ROI) and efficient removal of unwanted objects from the ROI. The performance of our proposed framework is competitive, as evidenced by our F1 score of 0.8177 and the fourth-place ranking that we achieved in track 4 of the 2023 AI City Challenge.
更多查看译文
关键词
2023 AI City Challenge,automated checkout system,bounding box,computer vision techniques,count products,cutting-edge artificial intelligence,DeepSort tracking,detect-then-track paradigm,detection region,motion blur,multifaceted issues,object occlusion,prominent challenges,real-world checkout scenarios,remarkable upswing,retail checkout,retail industry,ROI,similarity in scanned items,sophisticated deep learning-based framework,unwanted objects,video inpainting,YOLOv8 detection
AI 理解论文
溯源树
样例
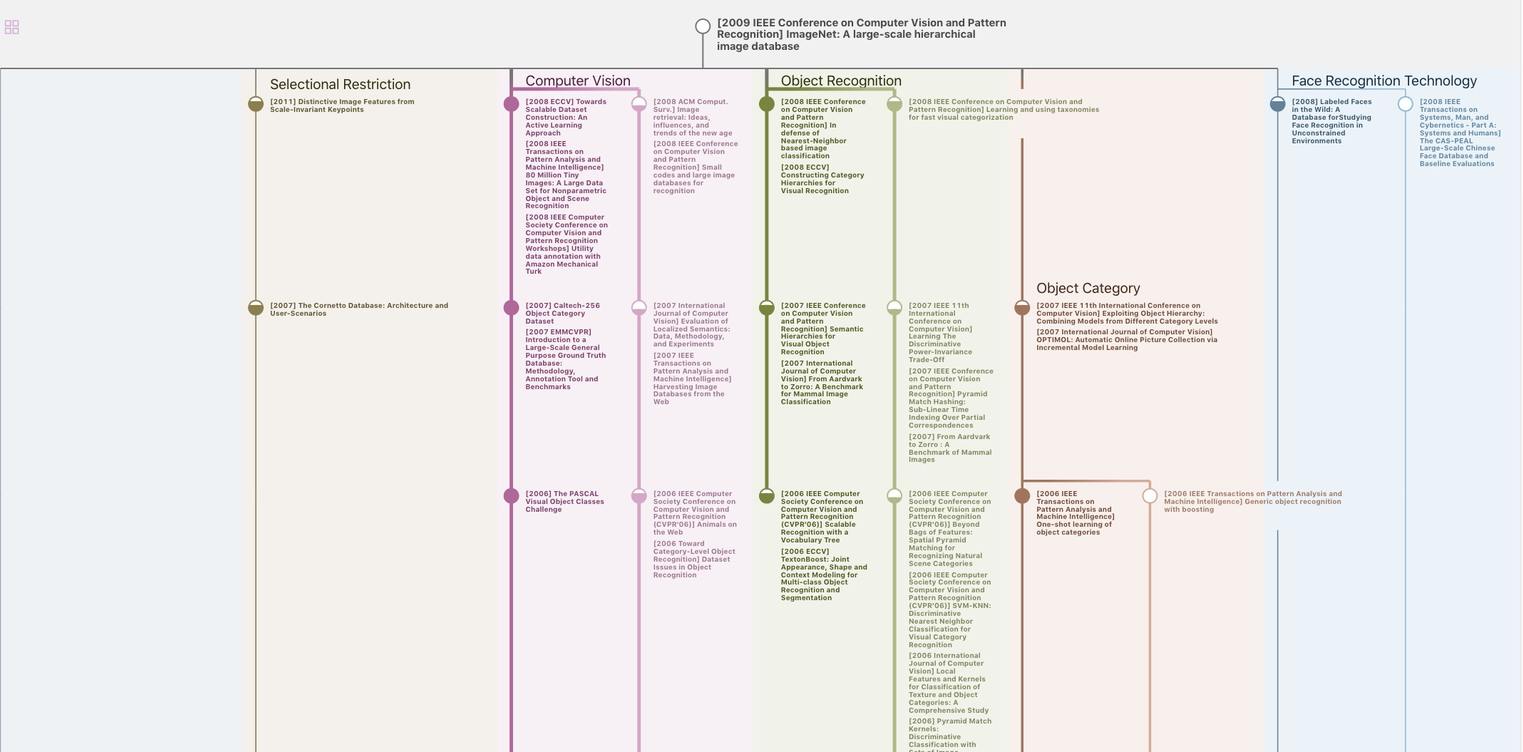
生成溯源树,研究论文发展脉络
Chat Paper
正在生成论文摘要