LSTC-rPPG: Long Short-Term Convolutional Network for Remote Photoplethysmography.
CVPR Workshops(2023)
摘要
Remote photoplethysmography (rPPG) is a non-contact technique for measuring blood pulse signals associated with cardiac activity. Although rPPG is considered an alternative to traditional contact-based photoplethysmography (PPG) because of its non-contact nature, obtaining reliable measurements remains a challenge owing to the sensitiveness of rPPG. In recent years, deep learning-based methods have improved the reliability of rPPG, but they suffer from certain limitations in utilizing long-term features such as periodic tendencies over long durations. In this paper, we propose a deep learning-based method that models long short-term spatio-temporal features and optimizes the long short-term features, ensuring reliable rPPG. The proposed method is composed of three key components: i) a deep learning architecture, denoted by LSTC-rPPG, which models long short-term spatio-temporal features and combines the features for reliable rPPG, ii) a temporal attention refinement module that mitigates temporal mismatches between the long-term and short-term features, and iii) a frequency scale invariant hybrid loss to guide long-short term features. In experiments on the UBFC-rPPG database, the proposed method demonstrated a mean absolute error of 0.7, root mean square error of 1.0, and Pearson correlation coefficient of 0.99 for heart rate estimation accuracy, outperforming contemporary state-of-the-art methods.
更多查看译文
关键词
blood pulse signals,deep learning architecture,deep learning-based method,long durations,long short-term convolutional network,long-short term features,long-term features,LSTC-rPPG,noncontact nature,noncontact technique,reliable rPPG,remote photoplethysmography,short-term features,short-term spatio-temporal features,temporal attention refinement module,traditional contact-based photoplethysmography,UBFC-rPPG database
AI 理解论文
溯源树
样例
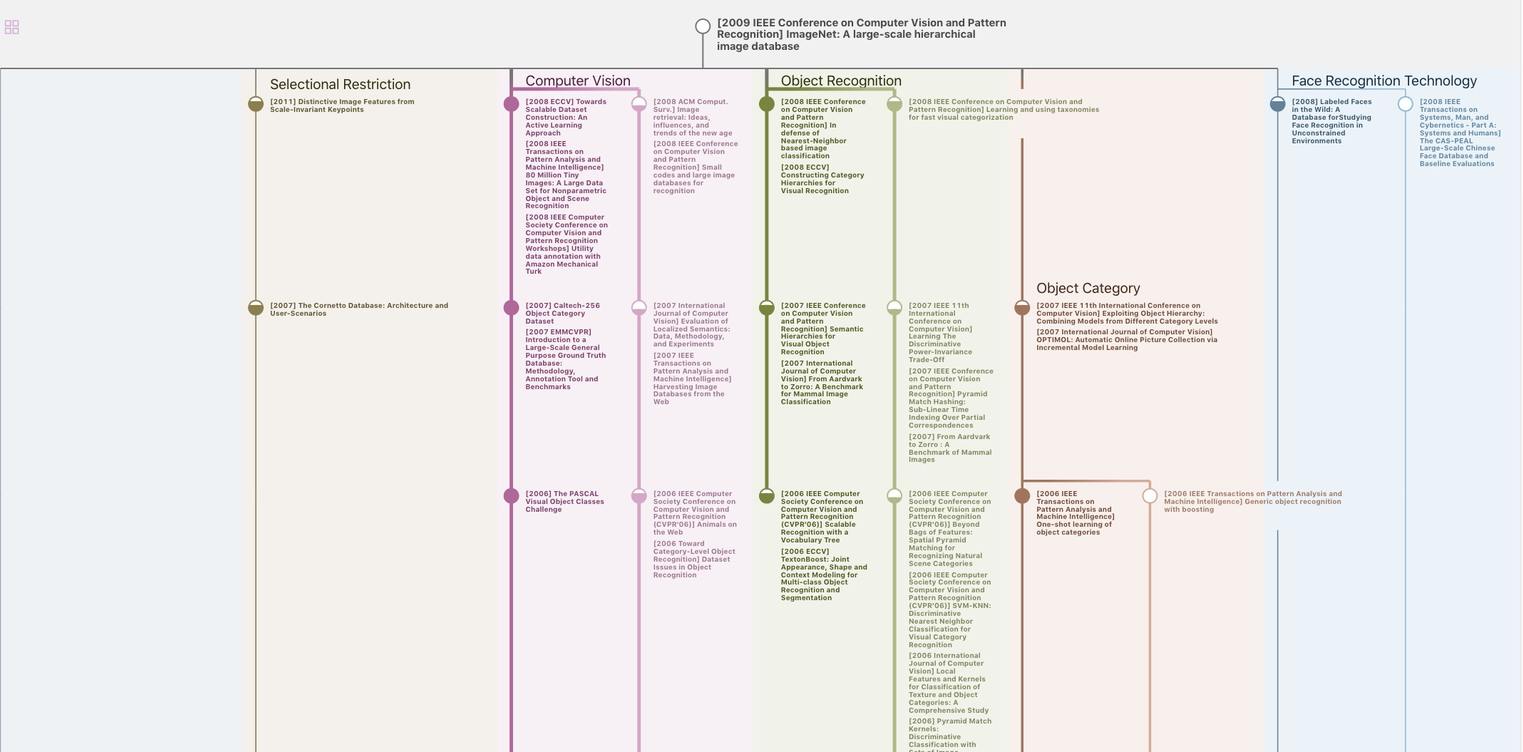
生成溯源树,研究论文发展脉络
Chat Paper
正在生成论文摘要