A System for Dense Monocular Mapping with a Fisheye Camera.
CVPR Workshops(2023)
摘要
We introduce a novel dense mapping system that uses a single monocular fisheye camera as the sole input sensor and incrementally builds a dense surfel representations of the scene’s 3D geometry. We extend an existing hybrid sparse-dense monocular SLAM system, reformulating the mapping pipeline in terms of the Kannala-Brandt fisheye camera model. Each frame is processed in its original undistorted fisheye form, with no attempt to remove distortion. To estimate depth, we introduce a new version of the PackNet depth estimation neural network adapted for fisheye inputs. We reformulate PackNet’s multi-view stereo self-supervised loss in terms of the Kannala-Brandt fisheye camera model. To encourage the network to learn metric depth during training, the pose network is weakly supervised with the camera’s ground-truth inter-frame velocity. To improve overall performance, we additionally provide sparse depth supervision from dataset LiDAR and SICK laser scanners. We demonstrate our system’s performance on the realworld KITTI-360 benchmark dataset. Our experimental results show that our system is capable of accurate, metric camera tracking and dense surface reconstruction within local windows. Our system operates within real-time processing rates and in challenging conditions. We direct the reader to the following video where the system can be seen in operation: https://youtu.be/Y-9q_wfqocs.
更多查看译文
关键词
accurate camera tracking,dense monocular mapping,dense surface reconstruction,dense surfel representations,existing hybrid sparse-dense,fisheye inputs,Kannala-Brandt fisheye camera model,mapping pipeline,metric camera tracking,metric depth,novel dense mapping system,original undistorted fisheye form,PackNet depth estimation neural network,scene,single monocular fisheye camera,sole input sensor,sparse depth supervision
AI 理解论文
溯源树
样例
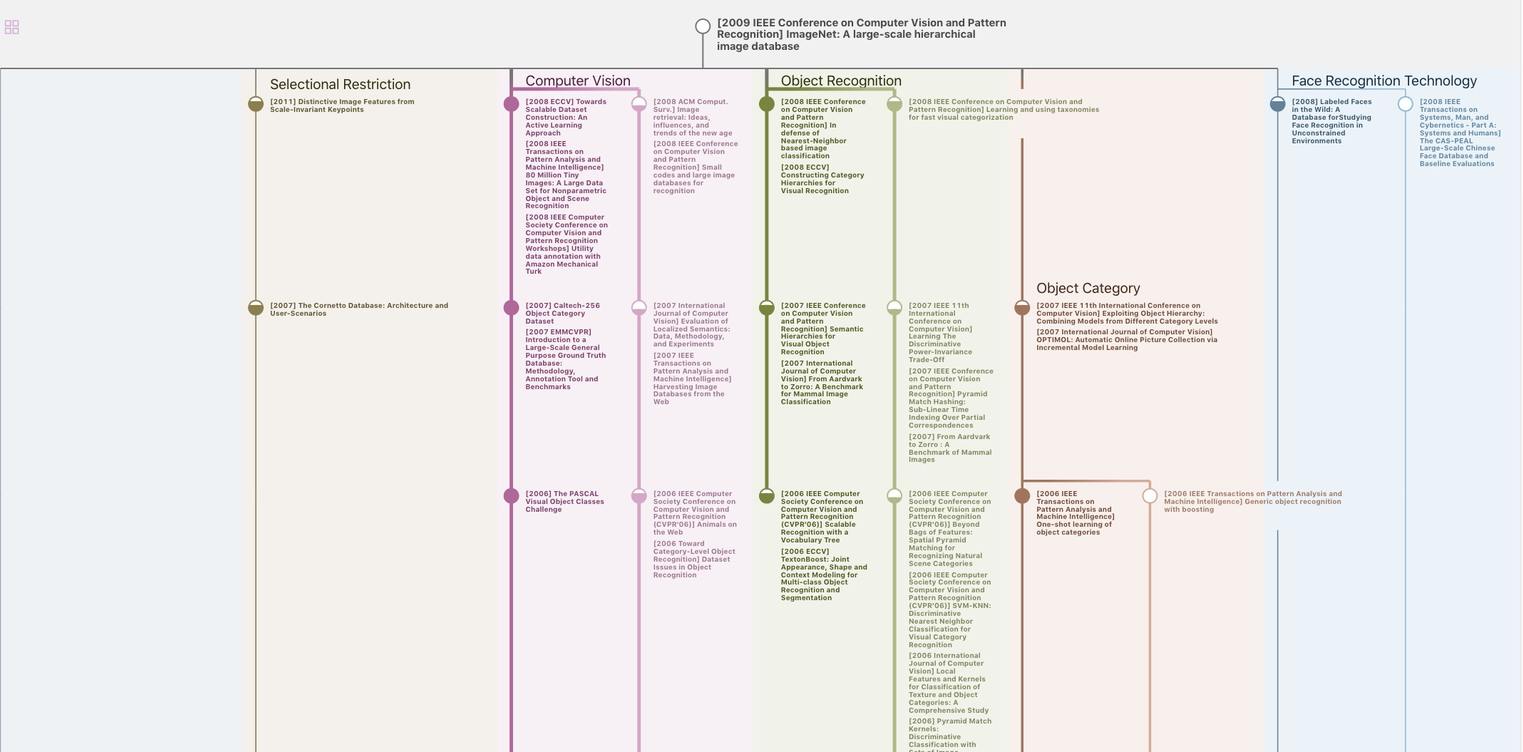
生成溯源树,研究论文发展脉络
Chat Paper
正在生成论文摘要