Video Analytics for Detecting Motorcyclist Helmet Rule Violations.
CVPR Workshops(2023)
摘要
The use of helmets is essential for motorcyclists' safety, but non-compliance with helmet rules remains a common issue. In this study, we extend the frontier of AI video analytic technologies for detecting violations of helmet rules among motorcyclists. Our method can handle highly challenging conditions for traditional methods, including occlusions, fast vehicle movement, shadows, large viewing angles, poor illumination and weather conditions. We adopt the widely used YOLOv7 object detector and develop a first baseline using YOLOv7-E6E. We further develop two improved versions, namely YOLOv7-CBAM and YOLOv7-SimAM that better address the challenges. Experiments are performed on the 2023 AI City Challenge Track 5 contest benchmark. Evaluation on the 100 test videos of the contest demonstrates the effectiveness of our approach. The baseline YOLOv7-E6E model trained with image size 1920 achieves 0.6112 mAP. The YOLOv7-CBAM achieves 0.6389 mAP, and YOLOv7-SimAM achieves 0.6422 mAP, where both are trained with image size 1280. These models rank sixth, fifth, and fourth on the public leaderboard, respectively, which outperforms over 36 global participating teams. The code for our models is available at: https://github.com/cmtsai2023/AICITY2023_Track5_DVHRM.
更多查看译文
关键词
100 test videos,2023 AI City Challenge Track 5 contest benchmark,AI video analytic technologies,baseline YOLOv7-E6E model,fast vehicle movement,helmet rules,helmets,highly challenging conditions,image size 1280,motorcyclist helmet rule violations,motorcyclists,poor illumination,video analytics,weather conditions,widely used YOLOv7 object detector,YOLOv7-CBAM,YOLOv7-SimAM
AI 理解论文
溯源树
样例
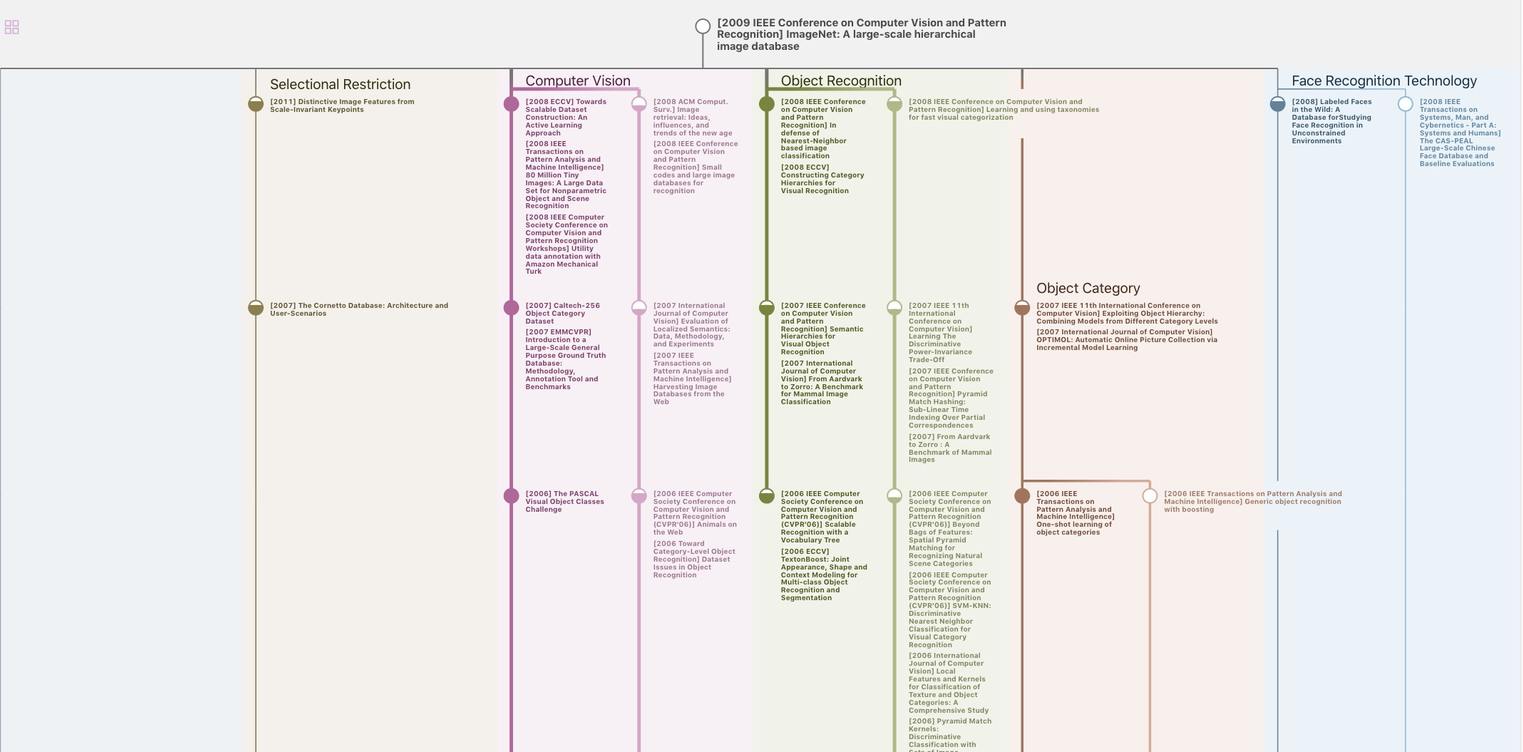
生成溯源树,研究论文发展脉络
Chat Paper
正在生成论文摘要