HUGNet: Hemi-Spherical Update Graph Neural Network applied to low-latency event-based optical flow.
CVPR Workshops(2023)
摘要
Event camera pixels asynchronously output binary events corresponding to local light intensity changes in time. While encoding visual information in this fashion increases sparsity and the temporal detail of motion with respect to frame-based cameras, there is not yet an established machine learning method capable of exploiting these features to increase efficiency, reduce latency and, ultimately, perform optimally in event-based tasks. Graph neural networks are a promising avenue for such a method, but current solutions are too slow to be compatible with the continuous streaming nature of event-data. In this study, we propose a hemi-spherical update event-graph neural network that significantly reduces the complexity and latency of graph updating and event-level prediction. We compare our approach to existing graph neural network methods, as well as to dense-frame convolutional neural networks, on optical flow estimation tasks. Relative to the previous state of the art in event-graphs, we reduce event-graph update latency by more than four orders of magnitude and reduce the number of neural network calculations per second by 70× while predicting optical flow more accurately.
更多查看译文
关键词
asynchronously output binary events,dense-frame convolutional neural networks,established machine learning method,event camera,event-based tasks,event-data,event-graph update,event-graphs,event-level prediction,graph neural network methods,graph neural networks,graph updating,hemi-spherical update event-graph neural network,hemi-spherical update graph neural network,low-latency event-based optical flow,neural network calculations
AI 理解论文
溯源树
样例
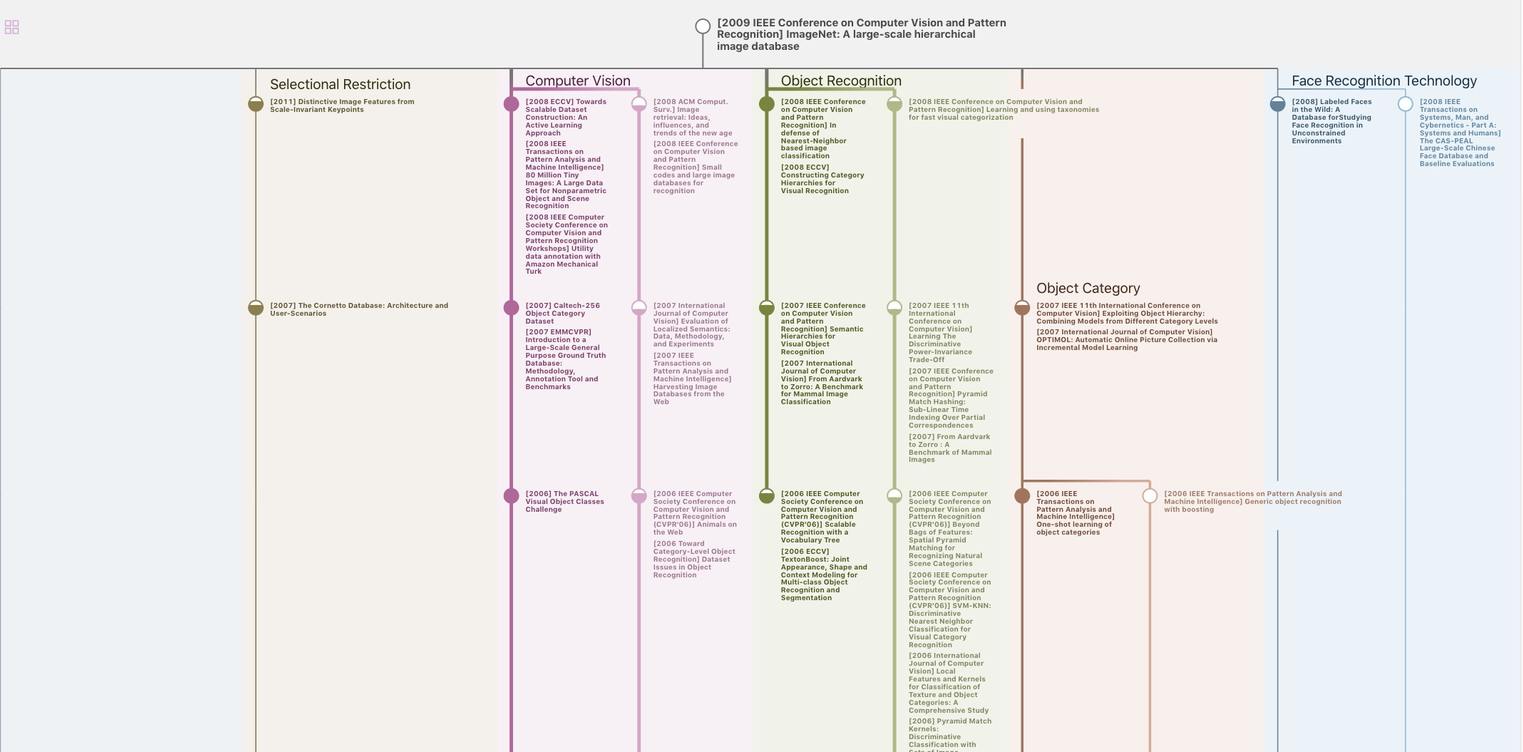
生成溯源树,研究论文发展脉络
Chat Paper
正在生成论文摘要