FewSOME: One-Class Few Shot Anomaly Detection with Siamese Networks.
CVPR Workshops(2023)
摘要
Recent Anomaly Detection techniques have progressed the field considerably but at the cost of increasingly complex training pipelines. Such techniques require large amounts of training data, resulting in computationally expensive algorithms that are unsuitable for settings where only a small amount of normal samples are available for training. We propose ‘Few Shot anOMaly dEtection’ (FewSOME), a deep One-Class Anomaly Detection algorithm with the ability to accurately detect anomalies having trained on ‘few’ examples of the normal class and no examples of the anomalous class. We describe FewSOME to be of low complexity given its low data requirement and short training time. FewSOME is aided by pretrained weights with an architecture based on Siamese Networks. By means of an ablation study, we demonstrate how our proposed loss, ‘Stop Loss’, improves the robustness of FewSOME. Our experiments demonstrate that FewSOME performs at stateof-the-art level on benchmark datasets MNIST, CIFAR-10, F-MNIST and MVTec AD while training on only 30 normal samples, a minute fraction of the data that existing methods are trained on. Moreover, our experiments show FewSOME to be robust to contaminated datasets. We also report F1 score and balanced accuracy in addition to AUC as a benchmark for future techniques to be compared against. Code available; https://github.com/niamhbelton/FewSOME.
更多查看译文
关键词
fewsome shot anomaly detection,siamese networks,anomaly detection,one-class
AI 理解论文
溯源树
样例
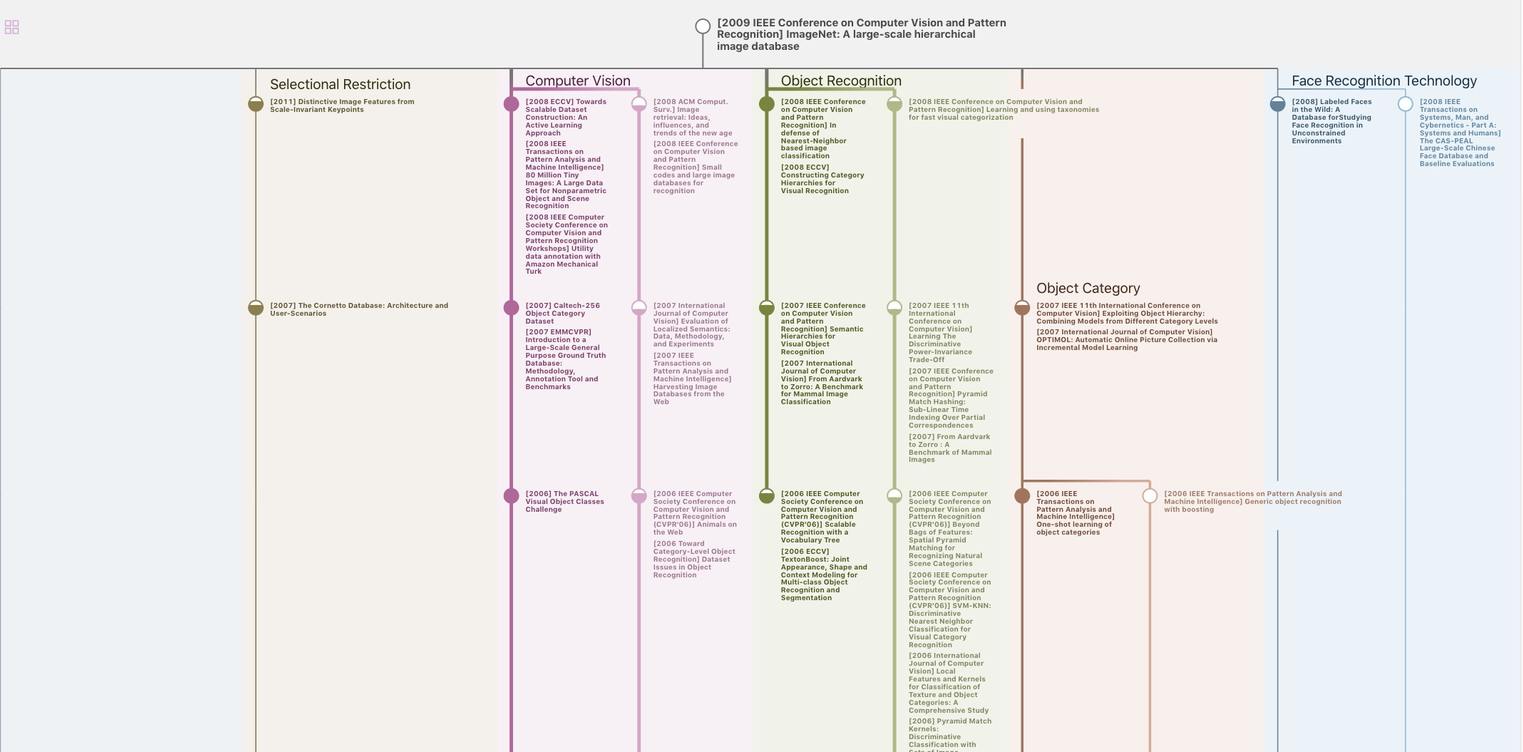
生成溯源树,研究论文发展脉络
Chat Paper
正在生成论文摘要