MetaLive: Meta-Reinforcement Learning Based Collective Bitrate Adaptation for Multi-Party Live Streaming.
Euro-Par(2023)
摘要
Multi-party interactive live video streaming applications have attracted millions of daily active users and are anticipated a blooming market in the next few years. A fundamental research problem in live streaming is bitrate coordination, which selects the proper upload/download bitrate for multiple participants in the system to maximize the users’ quality of experience (QoE). The existing bitrate adaptation methods fail to achieve optimal performance across a broad set of network conditions with conflict QoE objectives, and lack of the ability of adaptation to dynamic user requirements. In this paper, we proposed a novel meta-reinforcement learning based solution called MetaLive for multi-party live video streaming bitrate adaptation. The proposed framework formulates the bitrate coordination problem as a reinforcement learning task, and introduces a meta-training method to train an agent to learn to carry out various complex tasks from historical experience, and generate bitrate adaptation policies to maximize expected QoEs in diverse environments. We implement MetaLive based on an emulation platform, and use real-world network traces to evaluate its performance. Extensive experiments show that MetaLive achieves the best comprehensive QoE compared with the state-of-the-arts in a variety of network scenarios.
更多查看译文
关键词
collective bitrate adaptation,meta-reinforcement,multi-party
AI 理解论文
溯源树
样例
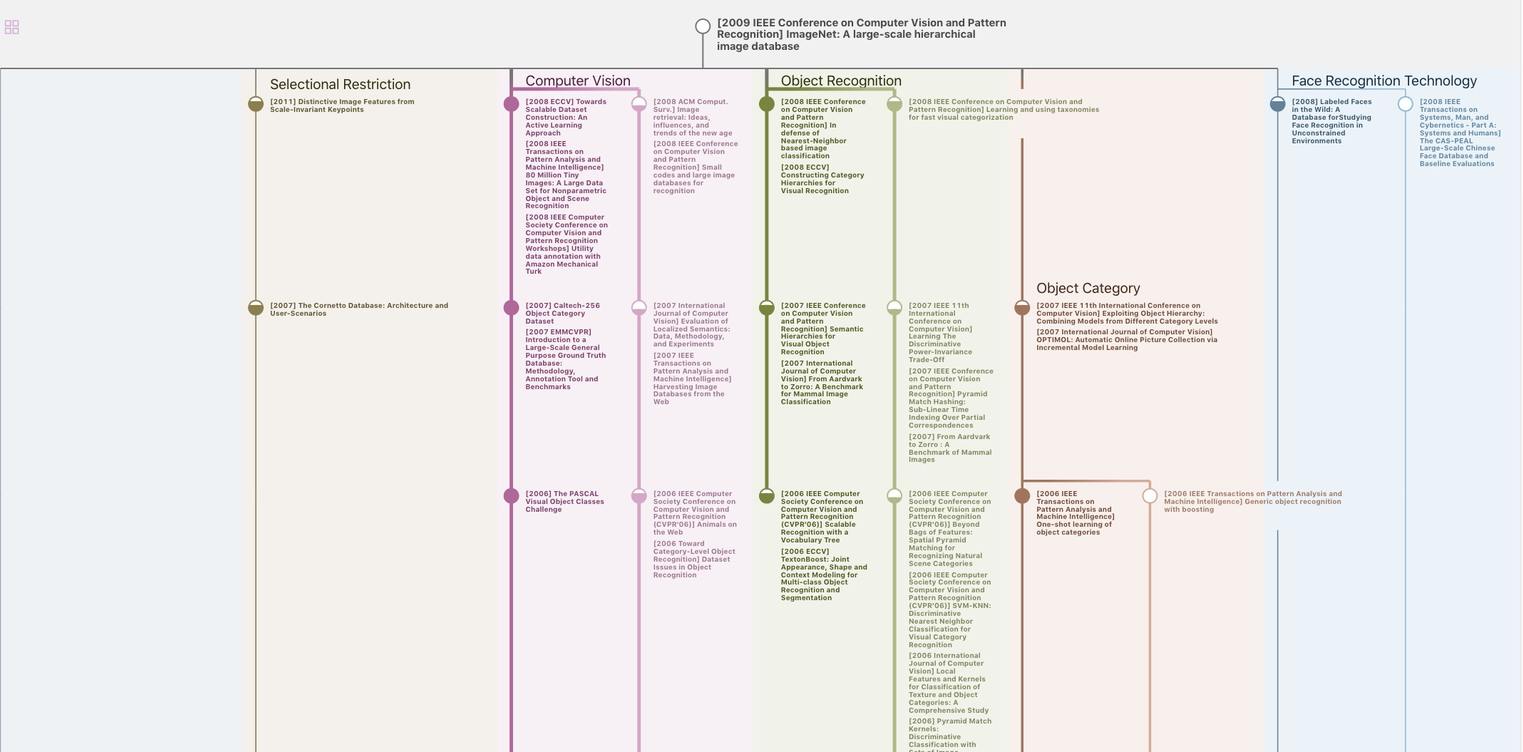
生成溯源树,研究论文发展脉络
Chat Paper
正在生成论文摘要