FedCML: Federated Clustering Mutual Learning with non-IID Data.
Euro-Par(2023)
摘要
Federated learning (FL) enables multiple clients to collaboratively train deep learning models under the supervision of a centralized aggregator. Communicating or collecting the local private datasets from multiple edge clients is unauthorized and more vulnerable to training heterogeneity data threats. Despite the fact that numerous studies have been presented to solve this issue, we discover that deep learning models fail to attain good performance in specific tasks or scenarios. In this paper, we revisit the challenge and propose an efficient federated clustering mutual learning framework (FedCML) with an semi-supervised strategy that can avoid the need for the specific empirical parameter to be restricted. We conduct extensive experimental evaluations on two benchmark datasets, and thoroughly compare them to state-of-the-art studies. The results demonstrate the promising performance from FedCML, the accuracy of MNIST and CIFAR10 can be improved by $$0.53\%$$ and $$1.58\%$$ for non-IID to the utmost extent while ensuring optimal bandwidth efficiency ( $$4.69\times $$ and $$4.73\times $$ less than FedAvg/FeSem for the two datasets).
更多查看译文
关键词
federated clustering mutual learning,non-iid
AI 理解论文
溯源树
样例
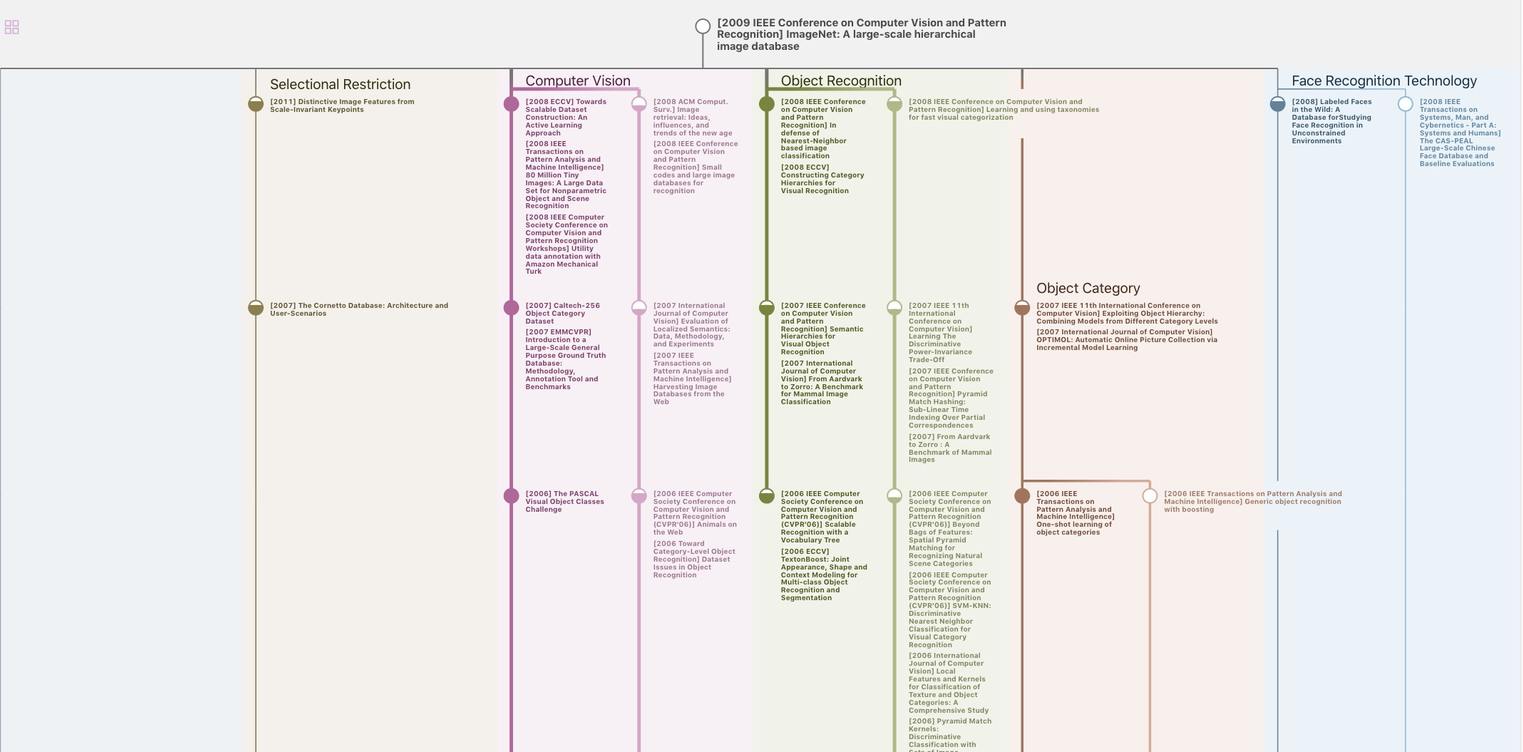
生成溯源树,研究论文发展脉络
Chat Paper
正在生成论文摘要