Multi-Resolution Diffeomorphic Image Registration with Convolutional Vision Transformer Network.
CNIOT(2023)
摘要
In recent years, the research of medical image registration based on convolutional neural network (CNN) has attracted much attention. In particular, the deformable image registration method based on diffeomorphism seems to have achieved promising results due to its unique topology conservation and transformation reversibility. However, the results of most existing learning-based approaches are not necessarily diffeomorphic. Moreover, due to local receptive fields caused by convolutional inductive bias, CNNs usually have limitations in catching the global and remote spatial relationships between points in anatomical images. Vision Transformer (ViT) shows tremendous advantages in modeling long-term dependencies in sequential images due to its embedded self-attention mechanism. Therefore, we propose a hybrid convolution Vision Transformer Network (CViT) model based on multi-resolution diffeomorphism. The model employs a multi-resolution strategy to learn global connectivity and local context of medical images in the diffeomorphic mapping space, which can simultaneously integrate the advantages of CNN and ViT to provide a better understanding of spatial correspondence. We evaluate our approach respectively on a large scale and a small scale dataset of 3D brain MRI scans, gaining an average Dice of 0.813 on the OASIS dataset. Extensive quantitative and qualitative results show that our method achieves state-of-the-art performance while maintaining desirable diffeomorphism.
更多查看译文
关键词
multi-resolution
AI 理解论文
溯源树
样例
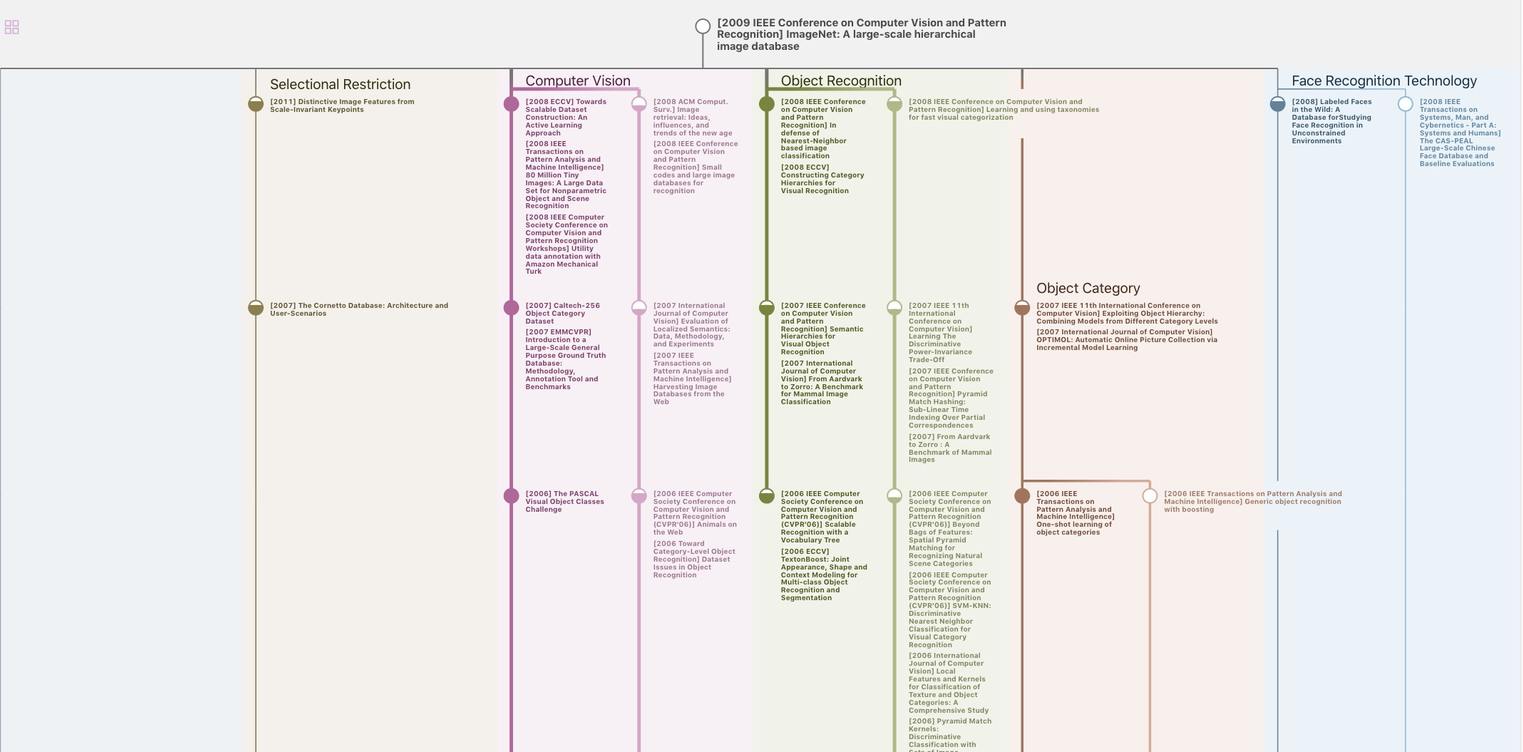
生成溯源树,研究论文发展脉络
Chat Paper
正在生成论文摘要