A Computer Vision Approach for Detecting Discrepancies in Map Textual Labels
35TH INTERNATIONAL CONFERENCE ON SCIENTIFIC AND STATISTICAL DATABASE MANAGEMENT, SSDBM 2023(2023)
摘要
Maps provide various sources of information. An important example of such information is textual labels such as cities, neighborhoods, and street names. Althoughwe treat this information as facts, and despite the massive effort done by providers to continuously improve their accuracy, this data is far from perfect. Discrepancies in textual labels rendered on the map are one of the major sources of inconsistencies across map providers. These discrepancies can have significant impacts on the reliability of the derived information and decision-making processes. Thus, it is important to validate the accuracy and consistency in such data. Most providers treat this data as their propriety data and it is not available to the public, thus we cannot compare the data directly. To address these challenges, we introduce a novel computer vision-based approach for automatically extracting and classifying labels based on the visual characteristics of the label, which indicates its category based on the format convention used by the specific map provider. Based on the extracted data, we detect the degree of discrepancies across map providers. We consider three map providers: Bing Maps, Google Maps, and OpenStreetMaps. The neural network we develop classifies the text labels with an accuracy up to 93% in all providers. We leverage our system to analyze randomly selected regions in different markets. The studied markets are USA, Germany, France, and Brazil. Experimental results and statistical analysis reveal the amount of discrepancies across map providers per region. We calculate the Jaccard distance between the extracted text sets for each pair of map providers, which represents the discrepancy percentage. Discrepancies percentages as high as 90% were found in some markets.
更多查看译文
关键词
detectron2,azure cognitive services,maps discrepancies,computer-vision,geospatial data,textual labels,faster-rcnn,neural networks
AI 理解论文
溯源树
样例
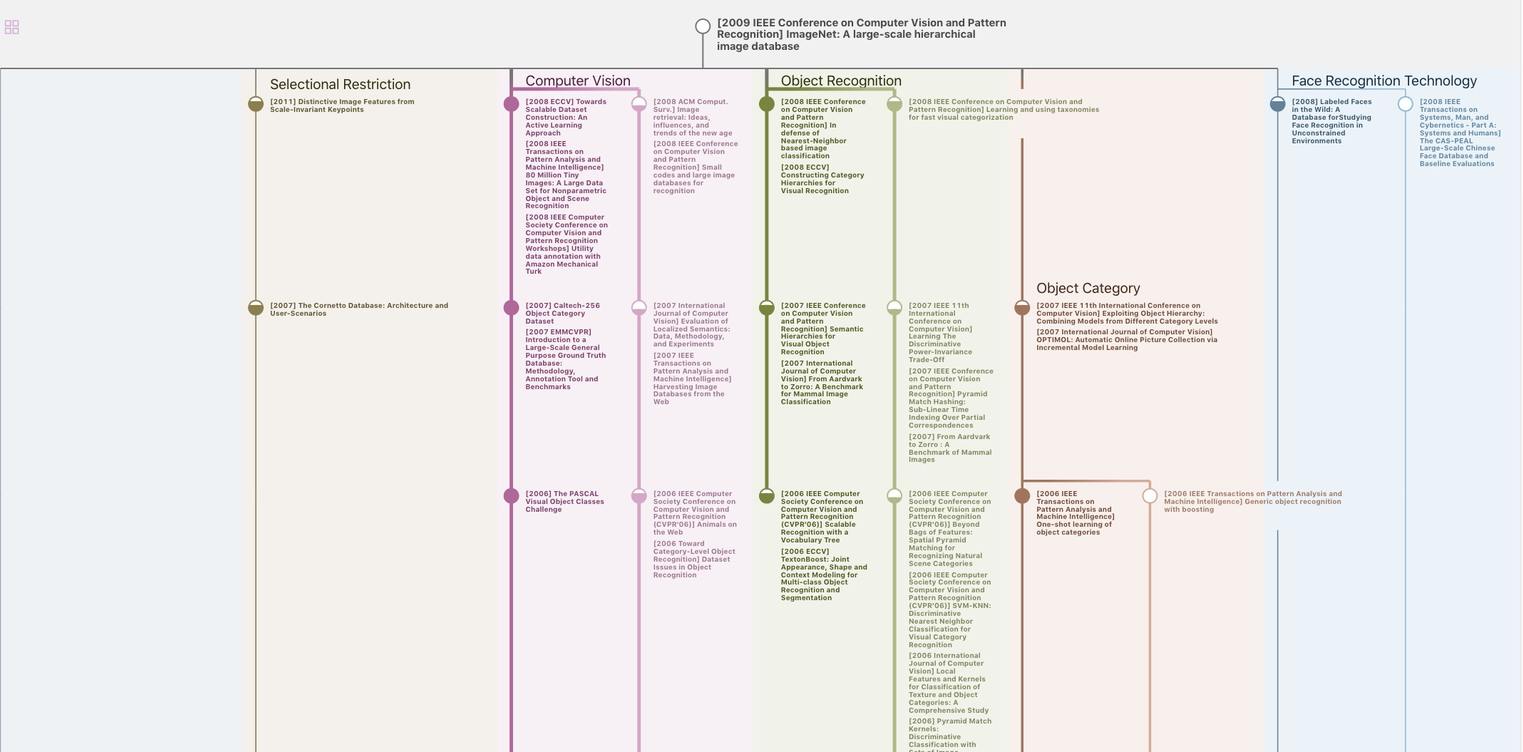
生成溯源树,研究论文发展脉络
Chat Paper
正在生成论文摘要