Decoupled Graph Neural Architecture Search with Variable Propagation Operation and Appropriate Depth.
35TH INTERNATIONAL CONFERENCE ON SCIENTIFIC AND STATISTICAL DATABASE MANAGEMENT, SSDBM 2023(2023)
摘要
To alleviate the over-smoothing problem caused by deep graph neural networks, decoupled graph neural networks (DGNNs) are proposed. DGNNs decouple the graph neural network into two atomic operations, the propagation (P) operation and the transformation (T) operation. Since manually designing the architecture of DGNNs is a time-consuming and expert-dependent process, the DF-GNAS method is designed, which can automatically construct the architecture of DGNNs with fixed propagation operation and deep layers. The propagation operation is a key process for DGNNs to aggregate graph structure information. However, DF-GNAS automatically designs DGNN architecture using fixed propagation operation for different graph structures will cause performance loss. Meanwhile, DF-GNAS designs deep DGNNs for graphs with simple distributions, which may lead to overfitting problems. To solve the above challenges, we propose the Decoupled Graph Neural Architecture Search with Variable Propagation Operation and Appropriate Depth (DGNAS-PD) method. In DGNAS-PD, we design a DGNN operation space with variable efficient propagation operations in order to better aggregate information on different graph structures. We build an effective genetic search strategy to adaptively design appropriate DGNN depths instead of deep DGNNs for the graph with simple distributions in DGNAS-PD. The experiments on five real-world graphs show that DGNAS-PD outperforms state-of-art baseline methods.
更多查看译文
关键词
Decoupled Graph neural networks,Graph neural architecture search learning,Propagation operation
AI 理解论文
溯源树
样例
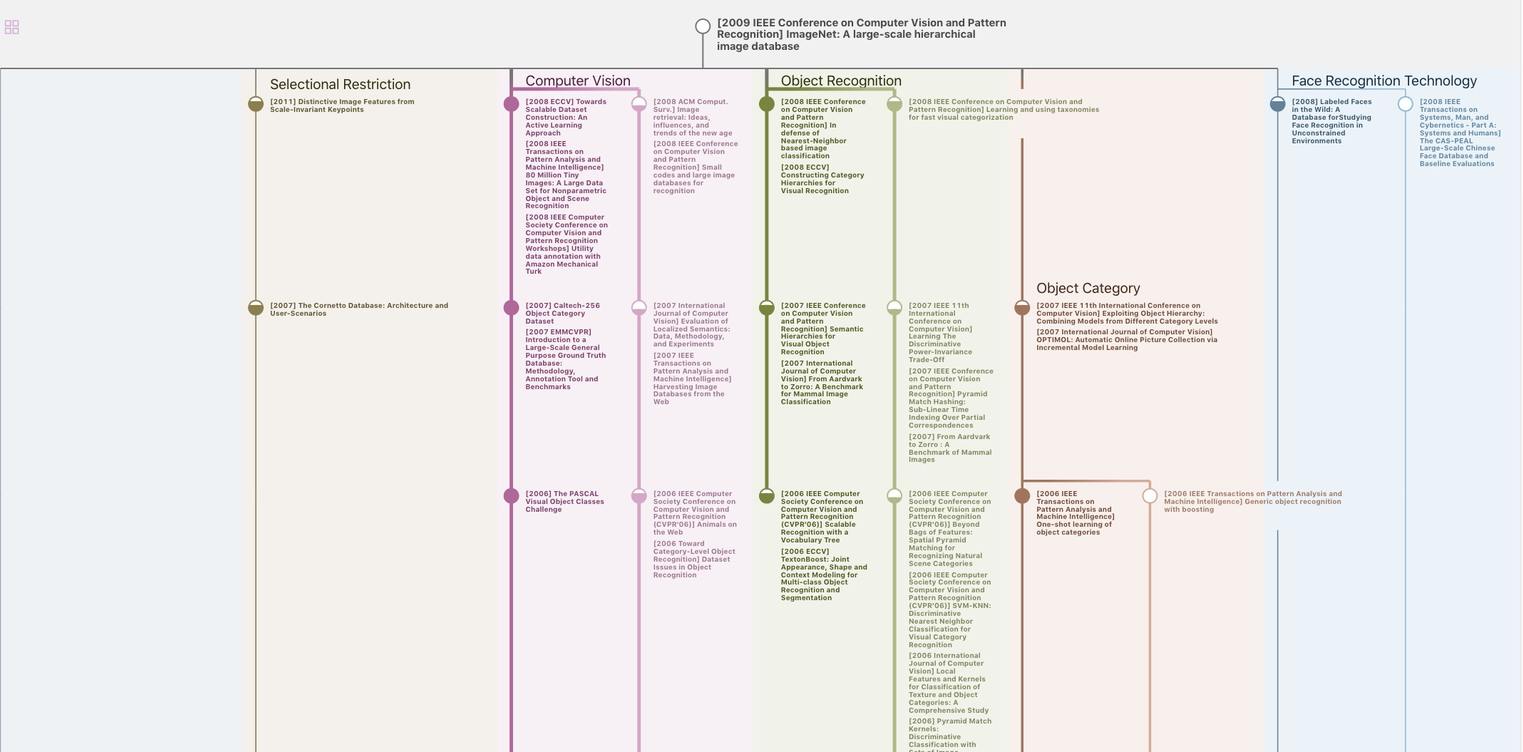
生成溯源树,研究论文发展脉络
Chat Paper
正在生成论文摘要