CBR-fox: A Case-Based Explanation Method for Time Series Forecasting Models.
ICCBR(2023)
摘要
Explainable Artificial Intelligence refers to methods that help human experts understand solutions developed by Artificial Intelligence systems in the form of black-box models, making them transparent and understandable. This paper describes CBR-fox, a post-hoc model-agnostic case-based explanation method for forecasting models. This method generates a case base of explanation examples through a sliding-window technique applied over the time series. Then, these explanation cases can be retrieved using a wide range of well-established metrics for time series comparison. Moreover, we introduce and evaluate a novel similarity metric named Combined Correlation Index. The proposed retrieval approach considers as a signal the similarity series resulting from applying the comparison metrics. This way, the signal can be smoothed using noise removal filters, such as the Hodrick-Prescott and low-pass filters, to avoid maximally similar cases that may overlap or represent a local slice of the source time series.. The resulting signal allows then to foster diversity in the retrieved explanation cases presented to the user The proposed case-based explanation approach is evaluated in the weather forecasting domain using an artificial neural network as the black-box model to be explained.
更多查看译文
关键词
time series forecasting models,time series,explanation method,cbr-fox,case-based
AI 理解论文
溯源树
样例
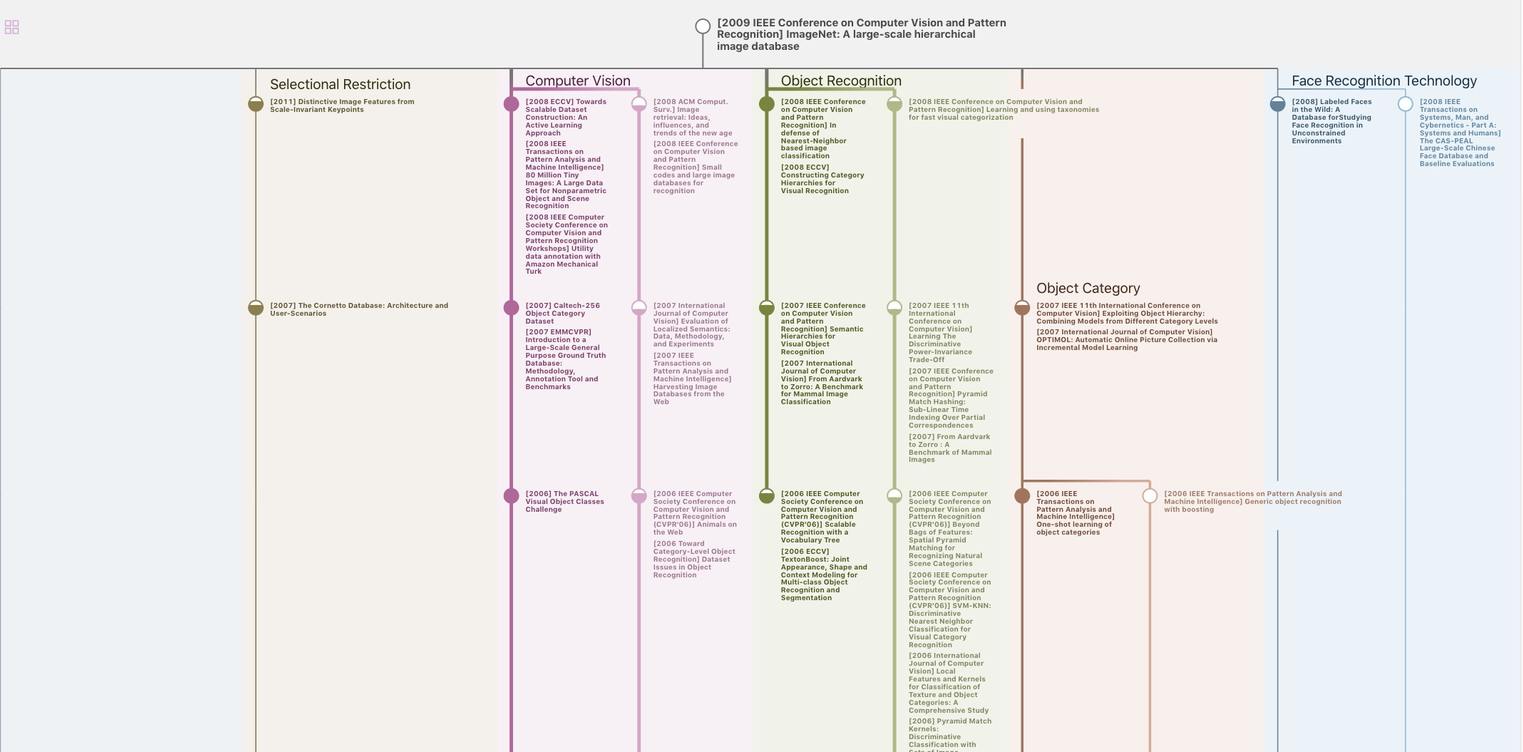
生成溯源树,研究论文发展脉络
Chat Paper
正在生成论文摘要