Towards Workload Trend Time Series Probabilistic Prediction via Probabilistic Deep Learning
PROCEEDINGS OF 2023 18TH INTERNATIONAL SYMPOSIUM ON SPATIAL AND TEMPORAL DATA, SSTD 2023(2023)
摘要
The workloads of autonomous driving traffic accident cloud data centers exhibit high variance and uncertainty. Accurate modeling and prediction of the variance and uncertainty of cloud workloads are crucial for the realization of reliable resource management in cloud data centers. Existing solutions are point prediction methods that can not capture the variance and uncertainty of the cloud workloads. In this paper, we propose a workload probabilistic prediction method with deep learning to model and predict the variance and uncertainty of cloud workload. Our method is a hybrid deep learning model which combines exponential smoothing, bidirectional long short-term memory (BLSTM) and quantile regression. First, a cloud workload pre-processing method based on exponential smoothing is proposed to smooth the high variance feature of cloud workloads. Then, a BLSTM based cloud workload algorithm is introduced. Finally, a differentiable quantile loss function is introduced into the prediction model to generate predictions of multiple quantiles. The experimental results on the Google cluster trace show that our method outperforms other four baseline models.
更多查看译文
关键词
Cloud computing,Probabilistic prediction,Reliable resource management,Distributed system,Deep learning,Exponential smoothing
AI 理解论文
溯源树
样例
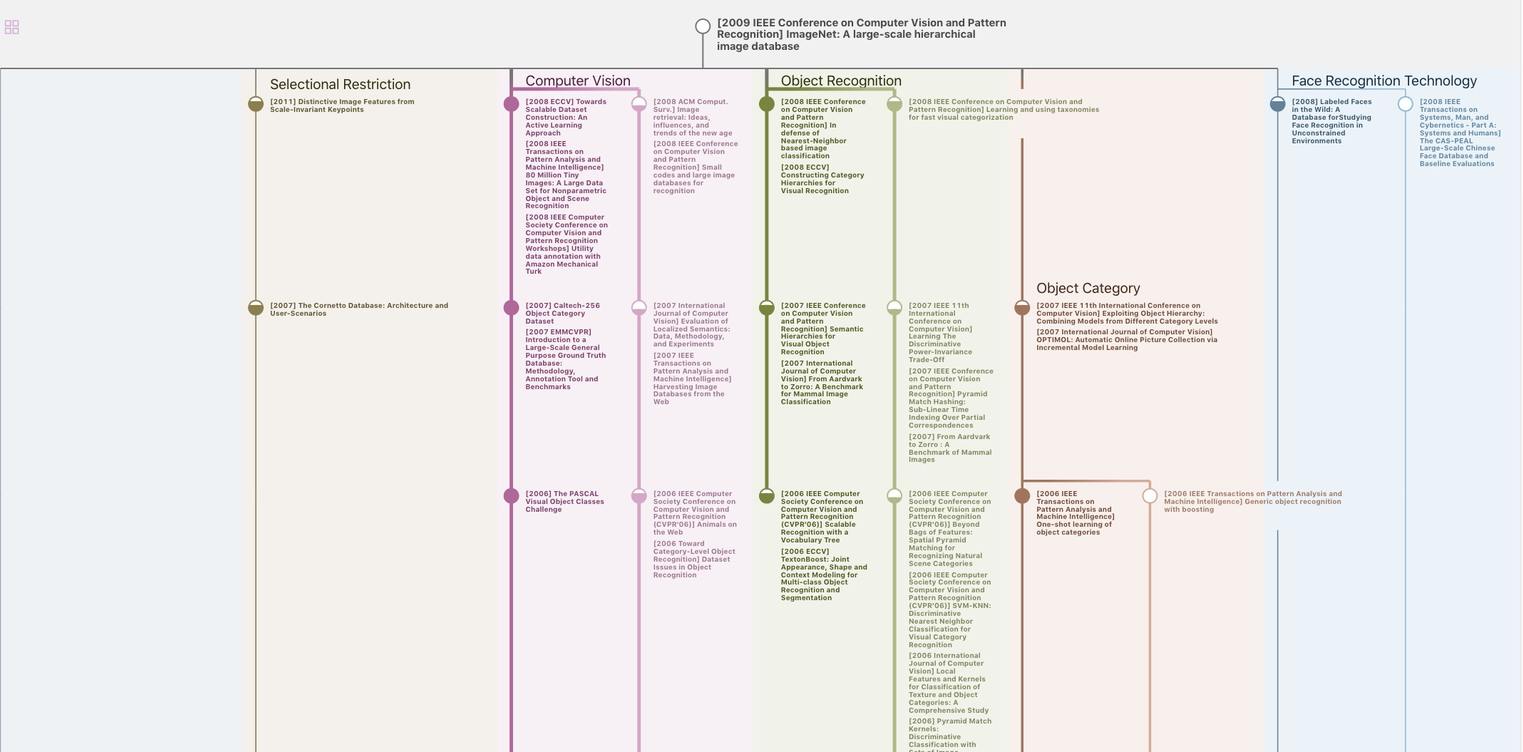
生成溯源树,研究论文发展脉络
Chat Paper
正在生成论文摘要