Zero-Shot Learning Based on Weighted Reconstruction of Hybrid Attribute Groups.
ICIC (4)(2023)
摘要
For the zero-shot learning, the rational description and utilization of attributes are valid approaches to build the bridge between training classes and testing classes. In order to improve the descriptive ability of attributes and further construct the appropriate mapping between attributes and features, a novel zero-shot learning method based on weighted reconstruction of hybrid attribute groups (WRHAG) is proposed. First, original semantic attributes are divided into groups by using the hierarchical clustering, and grouped semantic attributes are further enhanced by the broad learning. The semantic attribute groups and enhanced attribute groups together constitute hybrid attribute groups, which effectively improve the attribute description ability. Then, the mutual mapping between attributes and features is obtained by constructing a weighted autoencoder, in which the structured sparse L 21 norm and attribute group coefficients are adopted to choose the discriminative attributes and consider the differences between attribute groups. Finally, the zero-shot classification is achieved by calculating the similarity between features of testing sample and predicted class features in the feature space. Comparative experiments on typical CUB dataset demonstrate that the proposed WRHAG model yields better performance in zero-shot image classification.
更多查看译文
关键词
hybrid attribute groups,weighted reconstruction,learning,zero-shot
AI 理解论文
溯源树
样例
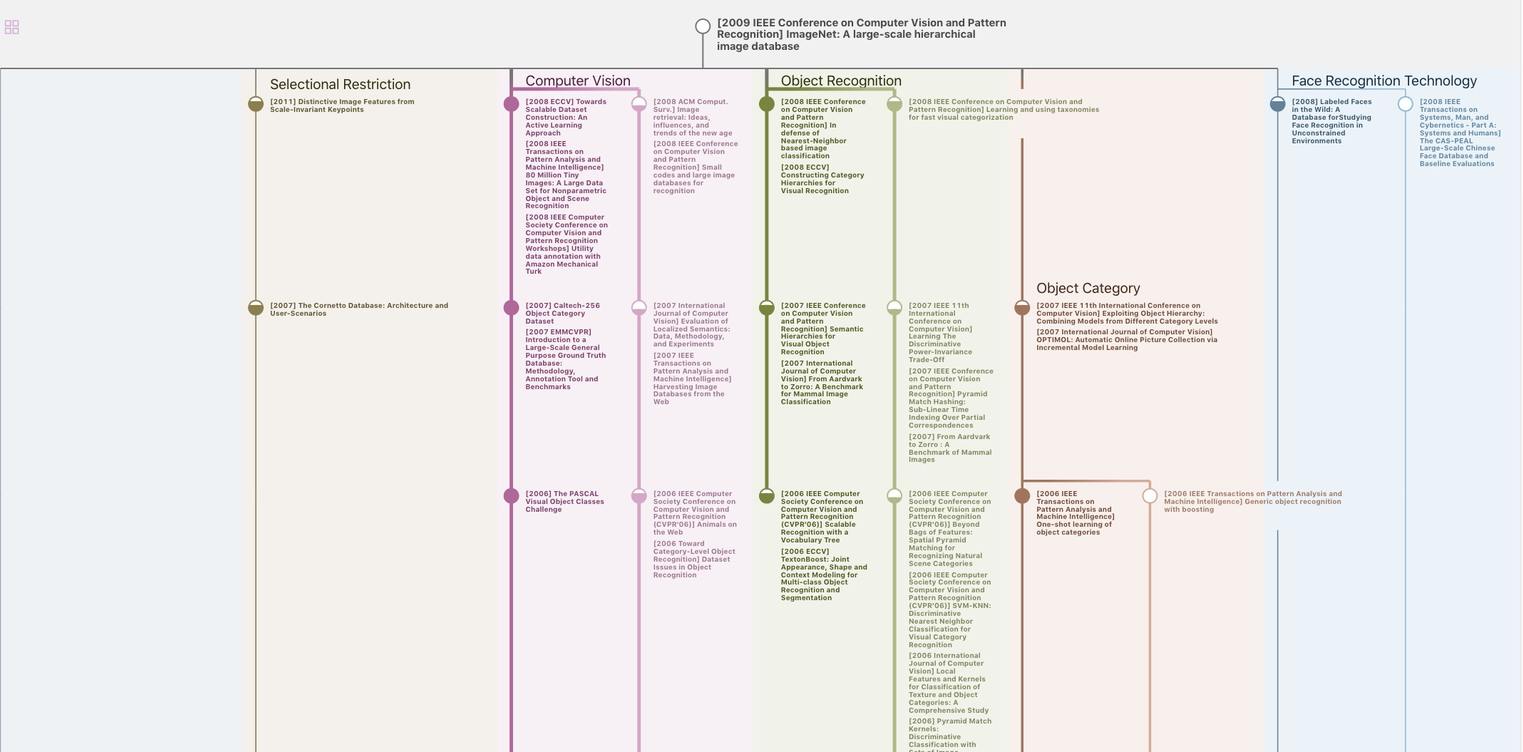
生成溯源树,研究论文发展脉络
Chat Paper
正在生成论文摘要