Deep Multi-view Clustering Based on Graph Embedding.
ICIC (1)(2023)
摘要
In recent decades, multi-view clustering has garnered more attention in the fields of machine learning and pattern recognition. Traditional multi-view clustering algorithms typically aim to identify a common latent space among the multi-view data and subsequently utilize k-means or spectral clustering techniques to derive clustering outcomes. These methods are time and space consuming while leading to splitting feature extraction and clustering. To address these problems, deep multi-view clustering based on graph embedding is proposed in this paper. First, multiple autoencoders are used to mine complementary information from multi-view and simultaneously seek the common latent representations. In addition, both the validity of the nearest neighbor correlation and the local geometric structure of multi-view data are taken in account and a novel graph embedding scheme is proposed. Specifically, the affinity graph information of the original data is directly applied to the soft assignment of data, which is consistent with the clustering loss, thus improving the performance of multi-view clustering. Numerous experiments conducted on various datasets exhibit the efficacy of our algorithm.
更多查看译文
关键词
deep,graph,multi-view
AI 理解论文
溯源树
样例
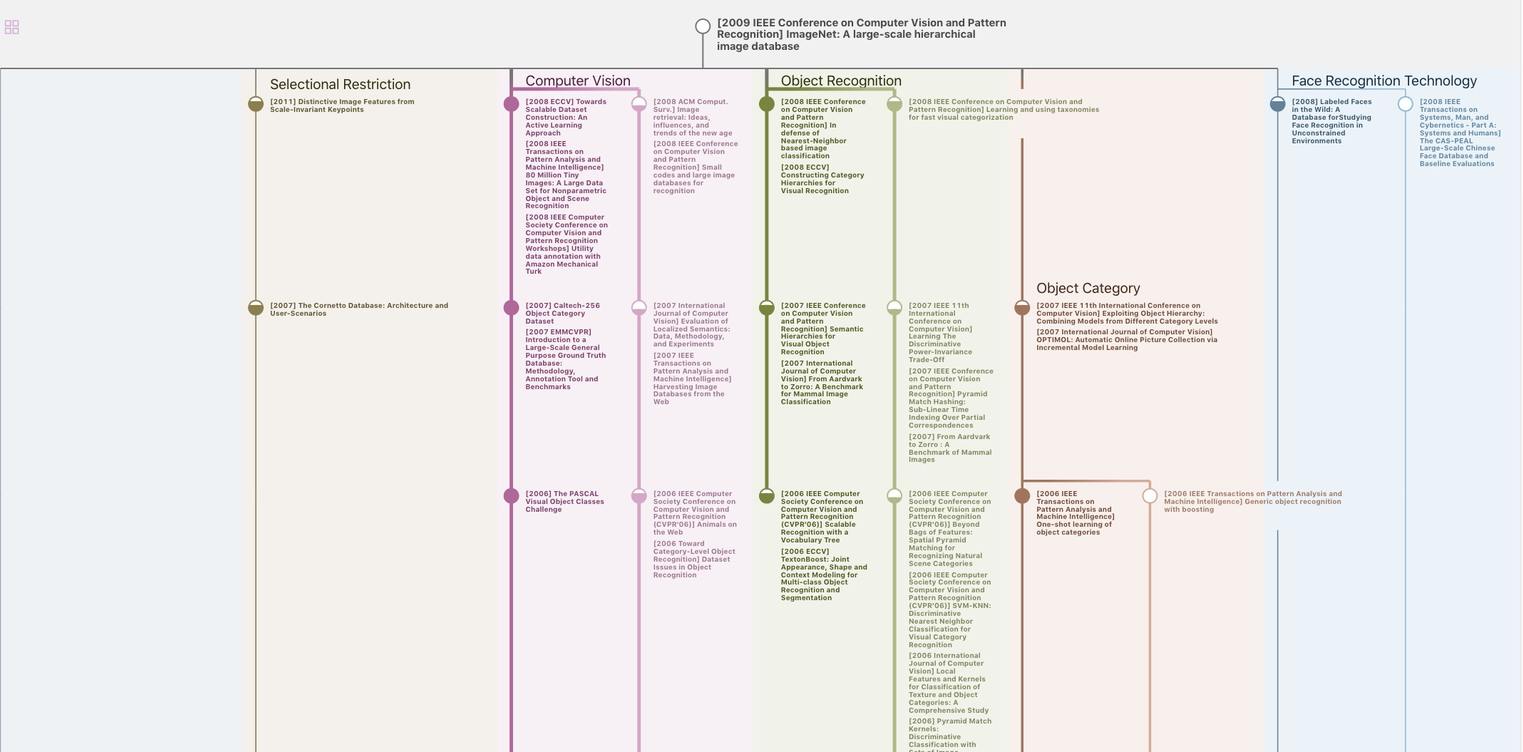
生成溯源树,研究论文发展脉络
Chat Paper
正在生成论文摘要