Multi-omics Cancer Subtype Recognition Based on Multi-kernel Partition Aligned Subspace Clustering.
ICIC (3)(2023)
摘要
With the widespread application of high-throughput technologies, multi-omics data are playing an increasingly important role in cancer subtyping. However, the heterogeneity and high-dimensionality of different omics data make it a challenging task to integrate them into a consistent model. In this paper, we propose a novel multi-omics cancer subtyping method based on Multi-Kernel Partition Alignment Subspace clustering (MKPAS). Given multiple omics datasets, MKPAS first uses multiple kernel functions to generate kernel matrices as the input of multi-view subspace learning model. Second, it uses subspace learning and rank constraint for each omics data learning an ideal graph structure. Third, to make the clustering results consistent from all omics datasets, a common clustering indication matrix is learned by using the multi-view partition alignment method. Finally, MKPAS integrates multi-kernel learning, subspace learning, graph learning, weights correction and partition alignment into a unified framework, making the process of multi-omics data clustering more intuitive and the clustering results more reliable. To validate the effectiveness of MKPAS, we conduct cancer subtyping experiments on multiple TCGA datasets. The experimental results show that MKPAS is effective for cancer subtype prediction.
更多查看译文
关键词
clustering,recognition,cancer,multi-omics,multi-kernel
AI 理解论文
溯源树
样例
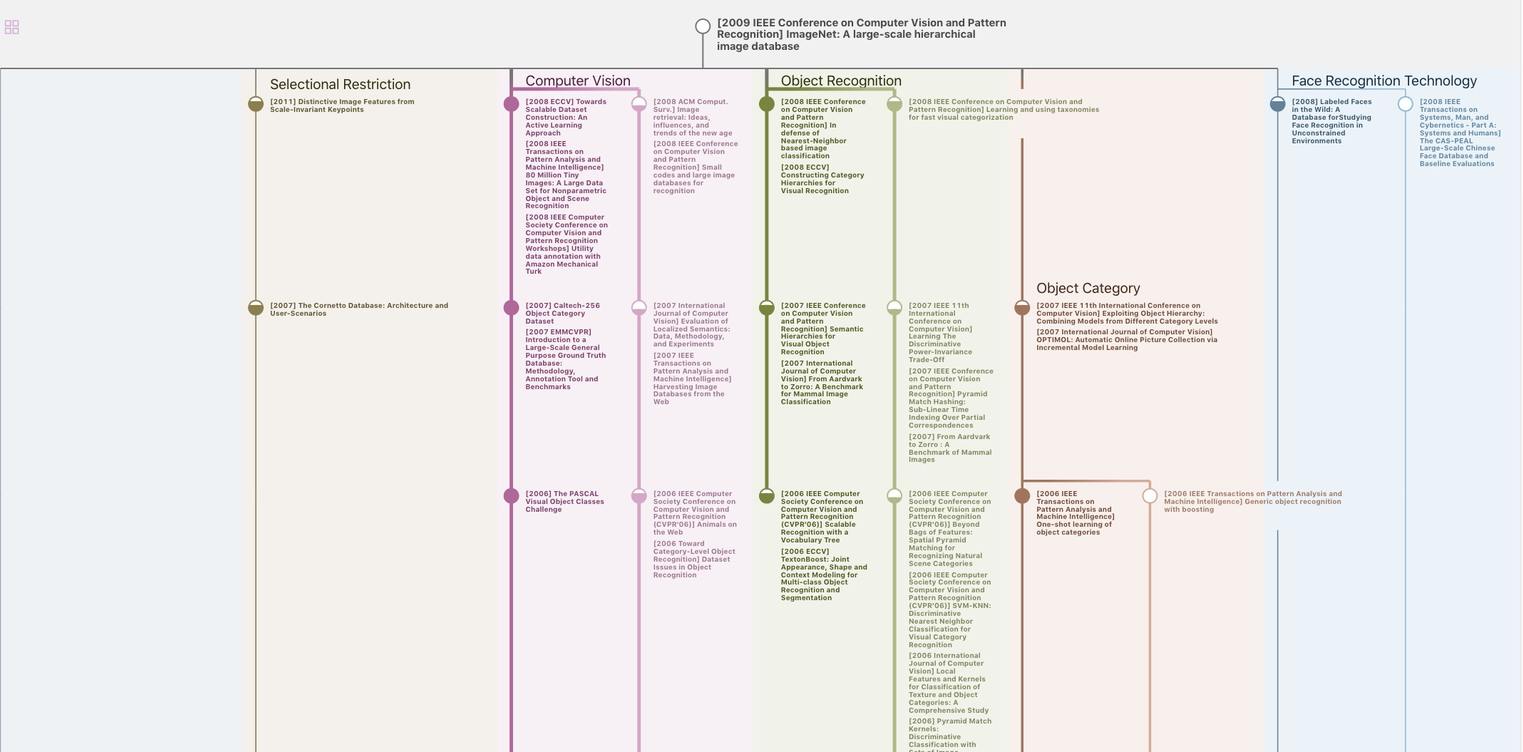
生成溯源树,研究论文发展脉络
Chat Paper
正在生成论文摘要