An Unsupervised Domain Adaptive Network Based on Category Prototype Alignment for Medical Image Segmentation.
ICIC (3)(2023)
Abstract
Computer-aided diagnosis is driven by data, the difference in the distribution of training and testing data leads to significant degradation in semantic segmentation model performance, known as the domain shift phenomenon. The unsupervised domain adaption method has become one of the solutions. Unsupervised domain adaption based on feature alignment is the mainstream method to reinforce feature alignment by reducing the variance of domain invariant features within a batch. However, when processing unsupervised domain adaption segmentation tasks of medical images, the feature alignment-based methods cannot learn domain invariant features stably due to the characteristics of a considerable distribution distance between features in the same category in medical images, resulting in degraded segmentation performance. To address this problem, we propose the Category Prototype Alignment Network(CPAN). First, we refine the category representative features from the domain invariant features. Second, unlike previous feature alignment methods, we construct category prototypes updated by multiple representative features to represent category features, and perform category alignment by reducing the distribution differences of category prototypes across domains, thus stabilizing feature alignment and enabling the network to learn category representative features. Experimental results of cross-domain thyroid ultrasound image segmentation show that our proposed method achieves comparable or even higher performance than current state-of-the-art methods.
MoreTranslated text
Key words
unsupervised domain adaptive network,medical image segmentation,category prototype alignment
AI Read Science
Must-Reading Tree
Example
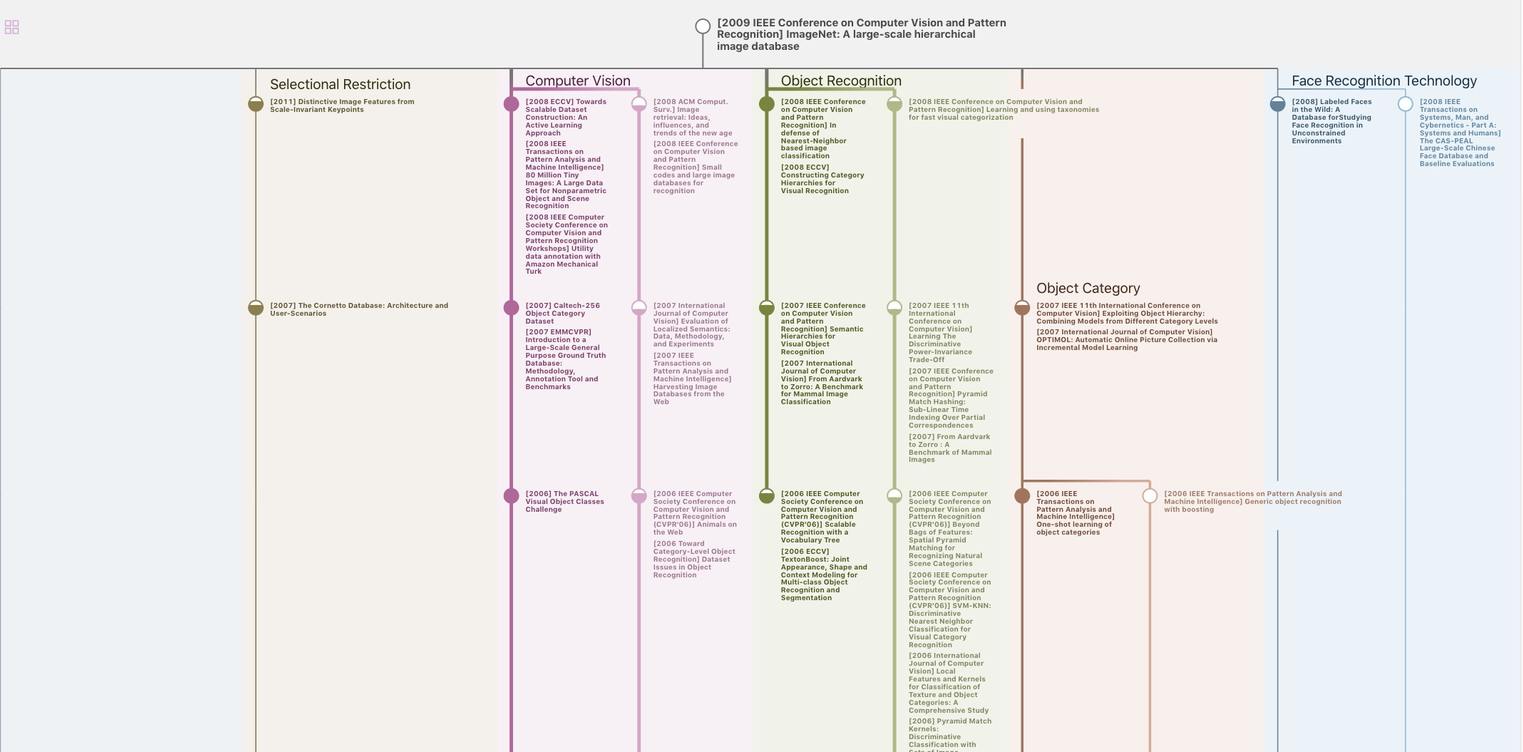
Generate MRT to find the research sequence of this paper
Chat Paper
Summary is being generated by the instructions you defined