Dynamic Attention Filter Capsule Network for Medical Images Segmentation.
ICIC (2)(2023)
摘要
In the existing works, Capsule Networks (CapsNets) have been proven to be promising alternatives to Convolutional Neural Networks (CNNs). However, CapsNets perform poorly on complex datasets with RGB backgrounds and cannot handle images with large input sizes. We propose a Dynamic Attention Filter (DAF) method to improve the performance of CapsNets. DAF is a filter unit between low-level capsule layers and voting layers, which can effectively filter the invalid background capsules and improve the classification performance of CapsNet. Besides, we propose DAF-CapsUNet for inputting medical images of large sizes, combining the advantages of the U-shaped encoder-decoder structure and DAF-CapsNet. Specifically, it contains three fundamental operations: an encoder is responsible for extracting shallow feature information, a capsule module is responsible for capturing the detailed feature information lost due to the pooling layer of the CNNs, and a decoder is responsible for fusing the feature information extracted from the two stages. Extensive experiments demonstrate that DAF can improve the performance of CapsNets on complex datasets and reduce the number of parameters, GPU memory cost, and running time of CapsNets. Benefiting from DAF-CapsNet, our model can achieve more useful information for precise localization. The medical experiments show that DAF-CapsUNet achieves SOTA performance compared to other segmentation models.
更多查看译文
关键词
capsule,segmentation,attention,images
AI 理解论文
溯源树
样例
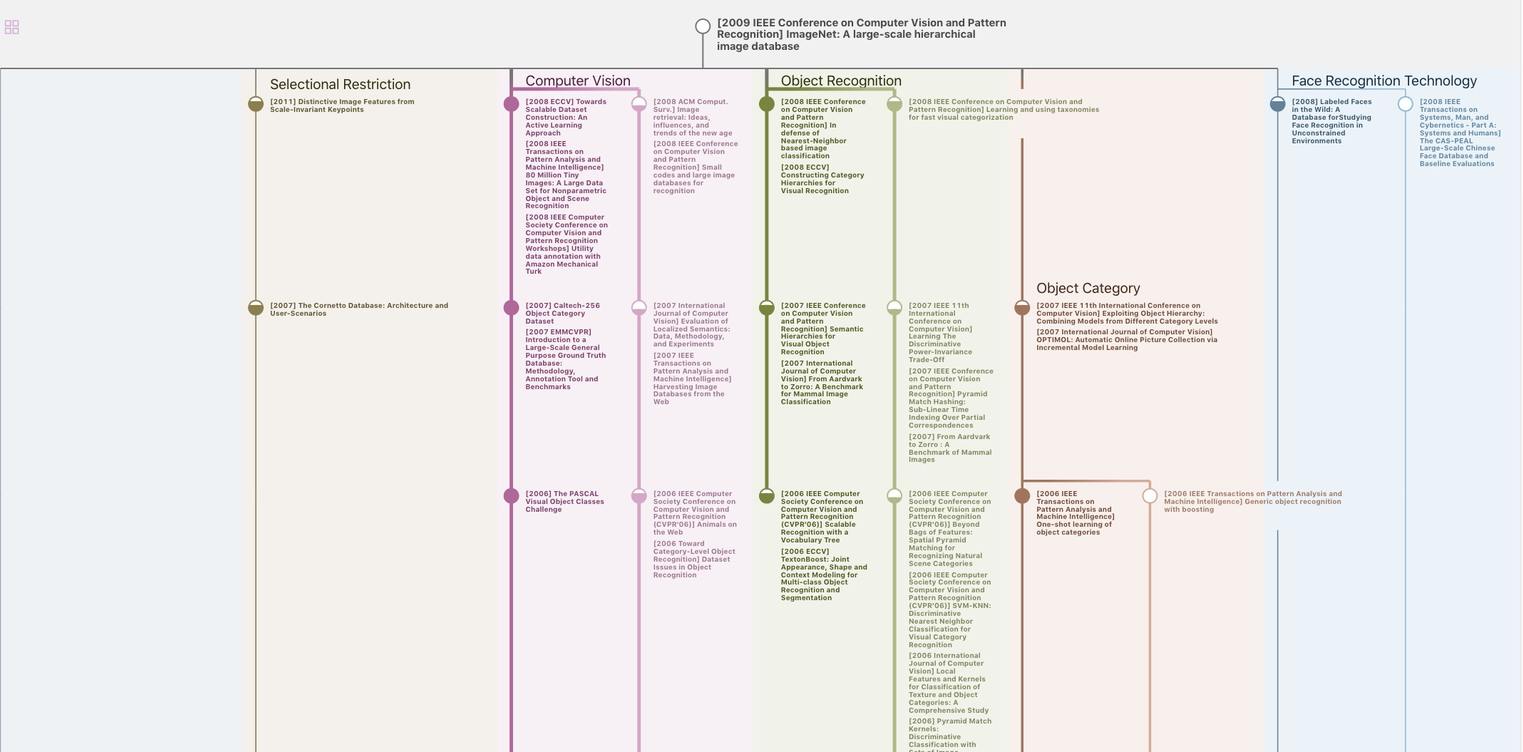
生成溯源树,研究论文发展脉络
Chat Paper
正在生成论文摘要