Functional Properties of PDE-Based Group Equivariant Convolutional Neural Networks.
GSI (1)(2023)
摘要
We build on the recently introduced PDE-G-CNN framework, which proposed the concept of non-linear morphological convolutions that are motivated by solving HJB-PDEs on lifted homogeneous spaces such as the homogeneous space of 2D positions and orientations isomorphic to . PDE-G-CNNs generalize G-CNNs and are provably equivariant to actions of the roto-translation group SE (2). Moreover, PDE-G-CNNs automate geometric image processing via orientation scores and allow for a meaningful geometric interpretation. In this article, we show various functional properties of these networks: PDE-G-CNNs satisfy crucial geometric and algebraic symmetries: they are semiring quasilinear, equivariant, invariant under time scaling, isometric, and are solved by semiring group convolutions. PDE-G-CNNs exhibit a high degree of data efficiency: even under limited availability of training data they show a distinct gain in performance and generalize to unseen test cases from different datasets. PDE-G-CNNs are extendable to well-known convolutional architectures. We explore a UNet variant of PDE-G-CNNs which has a new equivariant U-Net structure with PDE-based morphological convolutions. We verify the properties and show favorable results on various datasets.
更多查看译文
关键词
neural networks,group,pde-based
AI 理解论文
溯源树
样例
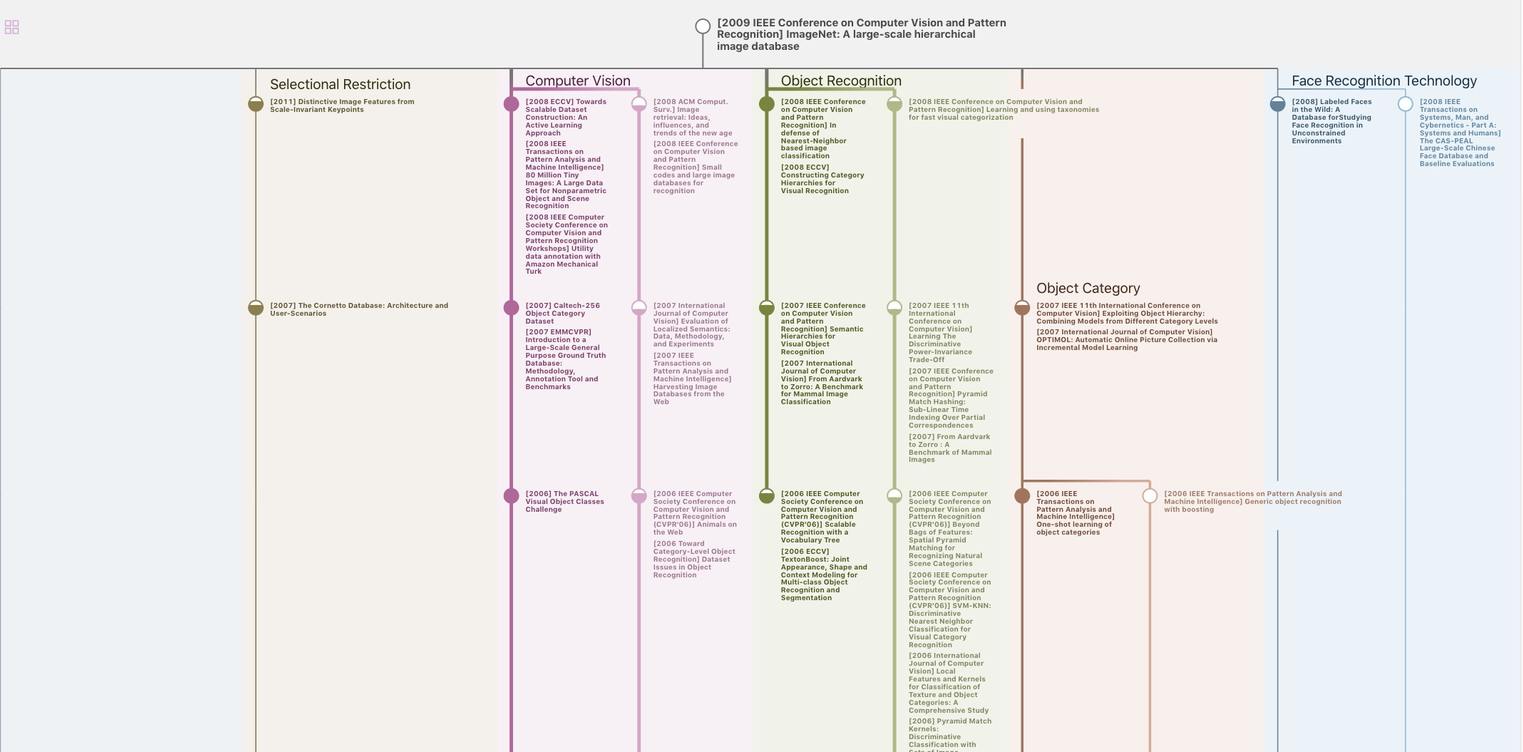
生成溯源树,研究论文发展脉络
Chat Paper
正在生成论文摘要