How to Learn Collaboratively - Federated Learning to Peer-to-Peer Learning and What's at Stake.
DSN-S(2023)
摘要
Standard ML relies on training using a centrally collected dataset, while collaborative learning techniques such as Federated Learning (FL) enable data to remain decentralized at client locations. In FL, a central server coordinates the training process, reducing computation and communication expenses for clients. However, this centralization can lead to server congestion and heightened risk of malicious activity or data privacy breaches. In contrast, Peer-to-Peer Learning (P2PL) is a fully decentralized system where nodes manage both local training and aggregation tasks. While P2PL promotes privacy by eliminating the need to trust a single node, it also results in increased computation and communication costs, along with potential difficulties in achieving consensus among nodes. To address the limitations of both FL and P2PL, we propose a hybrid approach called Hubs-and-Spokes Learning (HSL). In HSL, hubs function similarly to FL servers, maintaining consensus but exerting less control over spokes. This paper argues that HSL’s design allows for greater availability and privacy than FL, while reducing computation and communication costs compared to P2PL. Additionally, HSL maintains consensus and integrity in the learning process.
更多查看译文
关键词
federated-learning,peer-to-peer-learning,consensus,privacy,availability,communication-cost
AI 理解论文
溯源树
样例
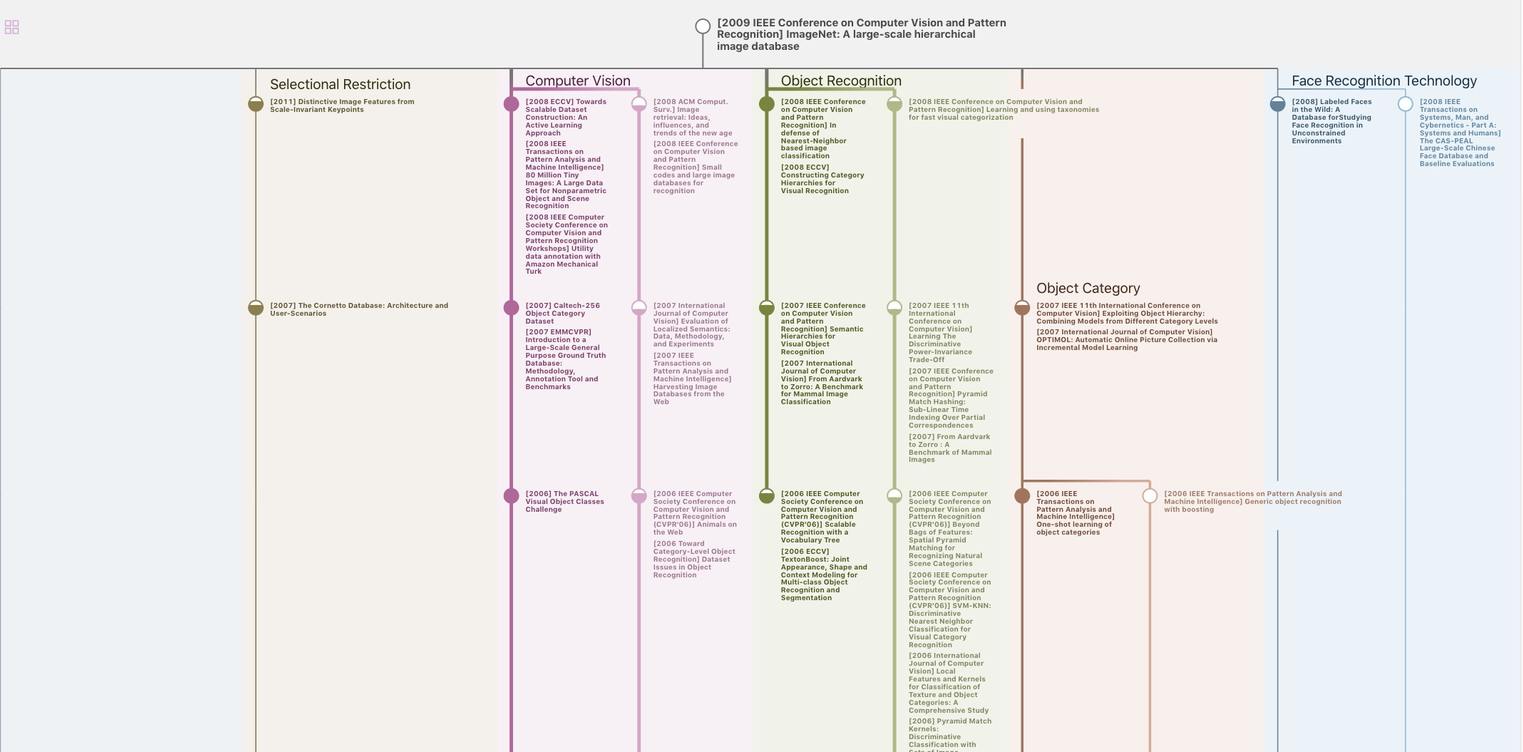
生成溯源树,研究论文发展脉络
Chat Paper
正在生成论文摘要