Trustiness-based hierarchical decentralized federated learning.
Knowl. Based Syst.(2023)
摘要
Federated Learning (FL) breaks the “data island” and lets clients cooperate in training a shared model with private data locally. And hierarchical framework is used in FL to alleviate the problem of excessive communication overhead. FL provides a certain degree of privacy, but privacy inference and Byzantine attacks still affect the existing FL methods. Moreover, the parameter server brings new security troubles, and the group size limits the model’s robustness. Thus, this paper presents a new decentralized FL framework called Trustiness-based Hierarchical Decentralized Federated Learning (TH-DFL) via a Security Robust Aggregation (SRA) rule, which introduces a trust mechanism. This study provides privacy protection and robustness even if there are malicious nodes. Besides, it somewhat reduces communication overhead. A series of comparative studies are also implemented to evaluate the performance of the model. In this process, the Gaussian, the bit-flipping, and other attacks are launched in the simulated federated learning. The results show that on the basis of ensuring privacy and robustness, TH-DFL seeks a better balance between privacy and robustness when the size of the group changes.
更多查看译文
关键词
learning,trustiness-based
AI 理解论文
溯源树
样例
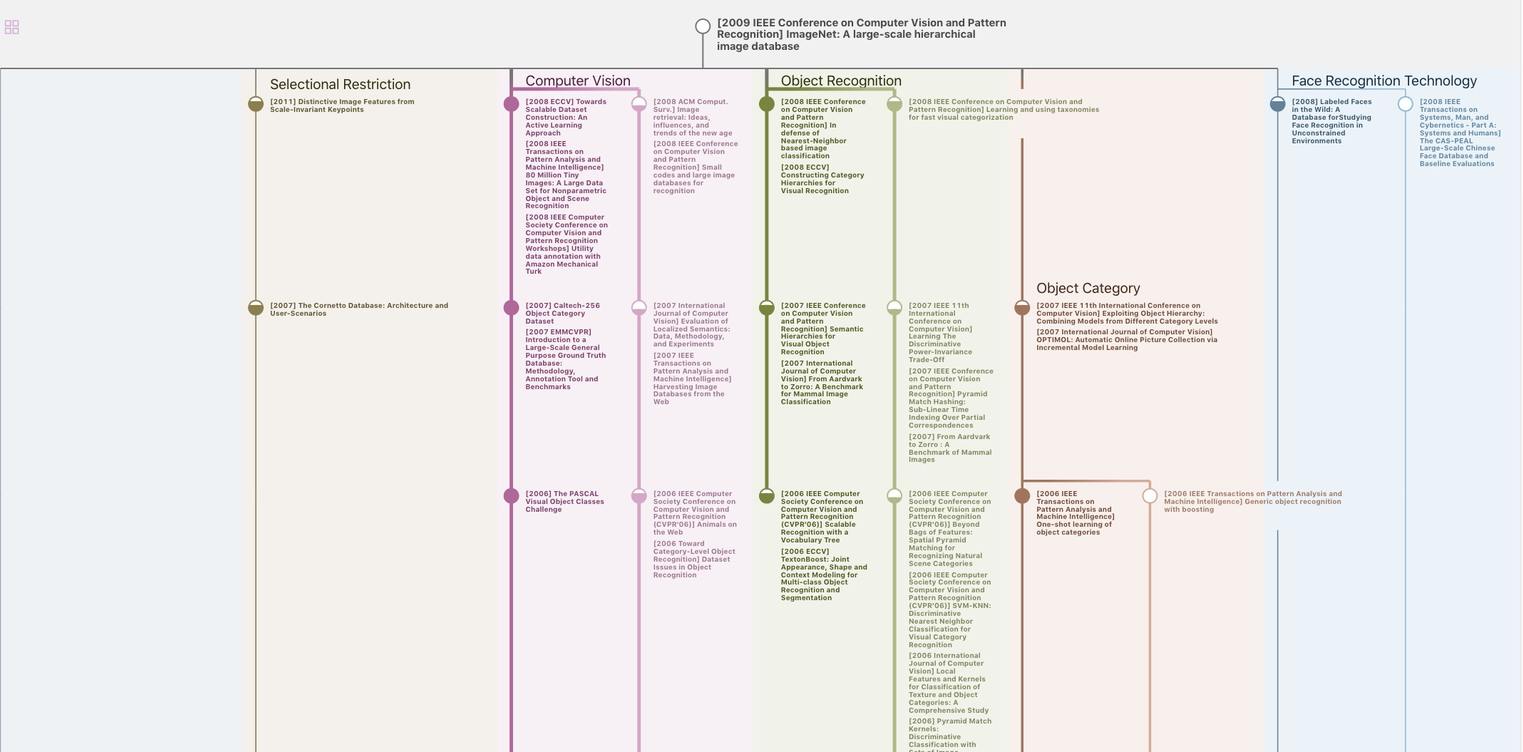
生成溯源树,研究论文发展脉络
Chat Paper
正在生成论文摘要