Bayesian, Multifidelity Operator Learning for Complex Engineering Systems-A Position Paper
J. Comput. Inf. Sci. Eng.(2023)
摘要
Deep learning has significantly improved the state-of-the-art in computer vision and natural language processing, and holds great potential to design effective tools for predicting and simulating complex engineering systems. In particular, scientific machine learning seeks to apply the power of deep learning to scientific and engineering tasks, with operator learning (OL) emerging as a particularly effective tool. OL can approximate nonlinear operators arising in complex engineering systems, making it useful for simulating, designing, and controlling those systems. In this position paper, we provide a comprehensive overview of OL, including its potential applications to complex engineering domains. We cover three variations of OL approaches: deterministic OL for modeling nonautonomous systems, OL with uncertainty quantification (UQ) capabilities, and multifidelity OL. For each variation, we discuss drawbacks and potential applications to engineering, in addition to providing a detailed explanation. We also highlight how multifidelity OL approaches with UQ capabilities can be used to design, optimize, and control engineering systems. Finally, we outline some potential challenges for OL within the engineering domain.
更多查看译文
关键词
multifidelity operator learning,complex engineering systems–a,bayesian
AI 理解论文
溯源树
样例
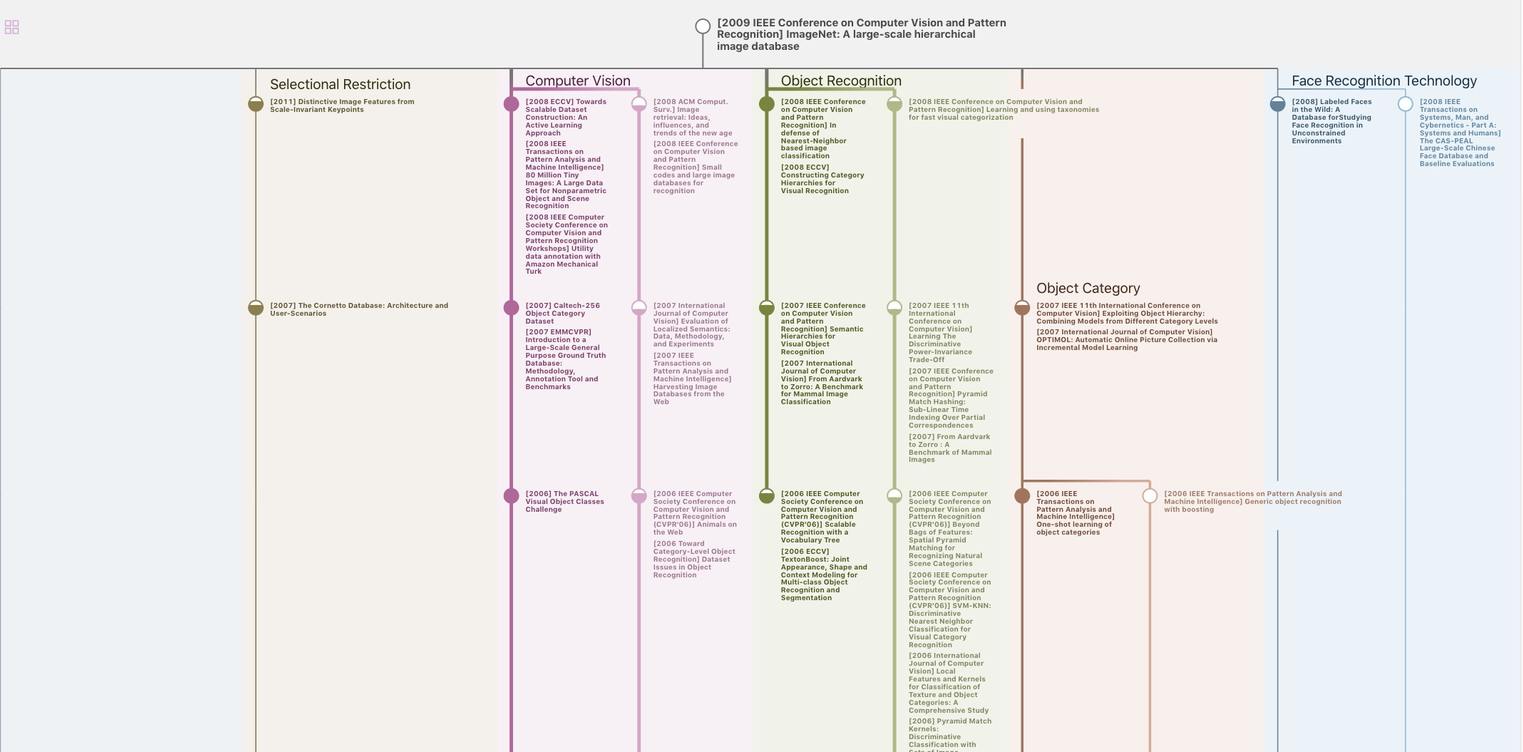
生成溯源树,研究论文发展脉络
Chat Paper
正在生成论文摘要