Machine learning techniques for received signal strength indicator prediction.
Intell. Data Anal.(2023)
摘要
The advances made in wireless communication technology have led to efforts to improve the quality of reception, prevent poor connections and avoid disconnections between wireless and cellular devices. One of the most important steps toward preventing communication failures is to correctly estimate the received signal strength indicator (RSSI) of a wireless device. RSSI prediction is important for addressing various challenges such as localization, power control, link quality estimation, terminal connectivity estimation, and handover decisions. In this study, we compare different machine learning (ML) techniques that can be used to predict the received signal strength values of a device, given the received signal strength values of other devices in the region. We consider various ML methods, such as multi-layer ANN, K nearest neighbors, decision trees, random forest, and the K-means based method, for the prediction challenge. We checked the accuracy level of the learning process using a real dataset provided by a major national cellular operator. Our results show that the weighted K nearest neighbors algorithm, for K = 3 neighbors, achieved, on average, the most accurate RSSI predictions. We conclude that in environments where the size of data is relatively small, and data of close geographical points is available, a method that predicts the coverage of a point using the coverage near geographical points can be more successful and more accurate compared with other ML methods.
更多查看译文
关键词
signal strength indicator prediction,machine learning
AI 理解论文
溯源树
样例
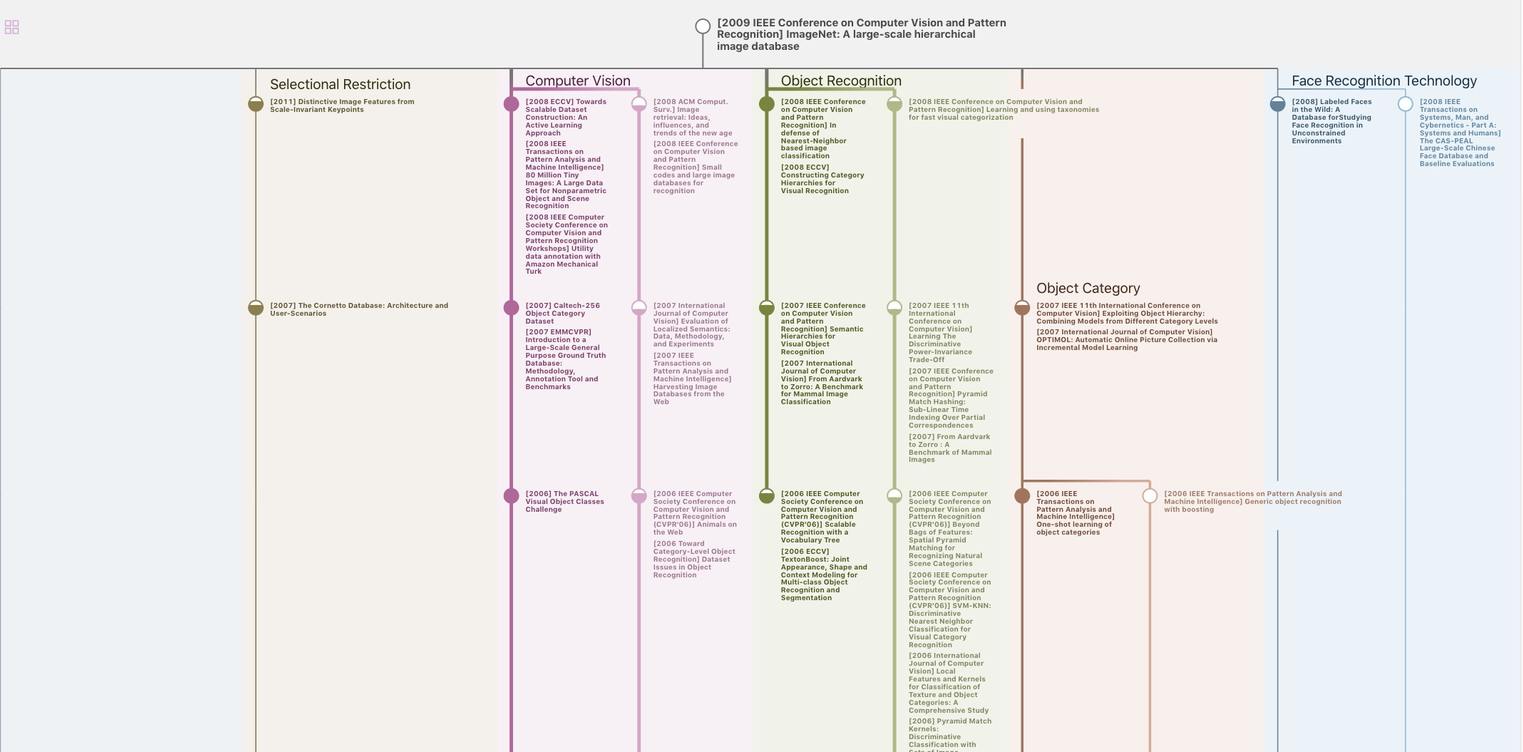
生成溯源树,研究论文发展脉络
Chat Paper
正在生成论文摘要