Structuring meaningful bug-fixing patches to fix software defect.
IET Softw.(2023)
摘要
Currently, software projects require a significant amount of time, effort and other resources to be invested in software testing to reduce the number of code defects. However, this process decreases the efficiency of software development and leads to a significant waste of workforce and resources. To address this challenge, researchers developed various solutions utilising deep neural networks. However, these solutions are frequently challenged by issues, such as a vast vocabulary, network training difficulties and elongated training processes resulting from the handling of redundant information. To overcome these limitations, the authors proposed a new neural network-based model named HopFix, designed to detect software defects that may be introduced during the coding process. HopFix consists of four parts: data preprocessing, encoder, decoder and code generation components, which were used for preprocessing data, extracting information about software defects, analysing defect information, generating software patches and controlling the generation process of software patches, respectively. Experimental studies on Bug-Fix Pairs (BFP) show that HopFix correctly fixed 47.2% (BFPsmall datasets) and 25.7% (BFPmedium datasets) of software defects.
更多查看译文
关键词
software
AI 理解论文
溯源树
样例
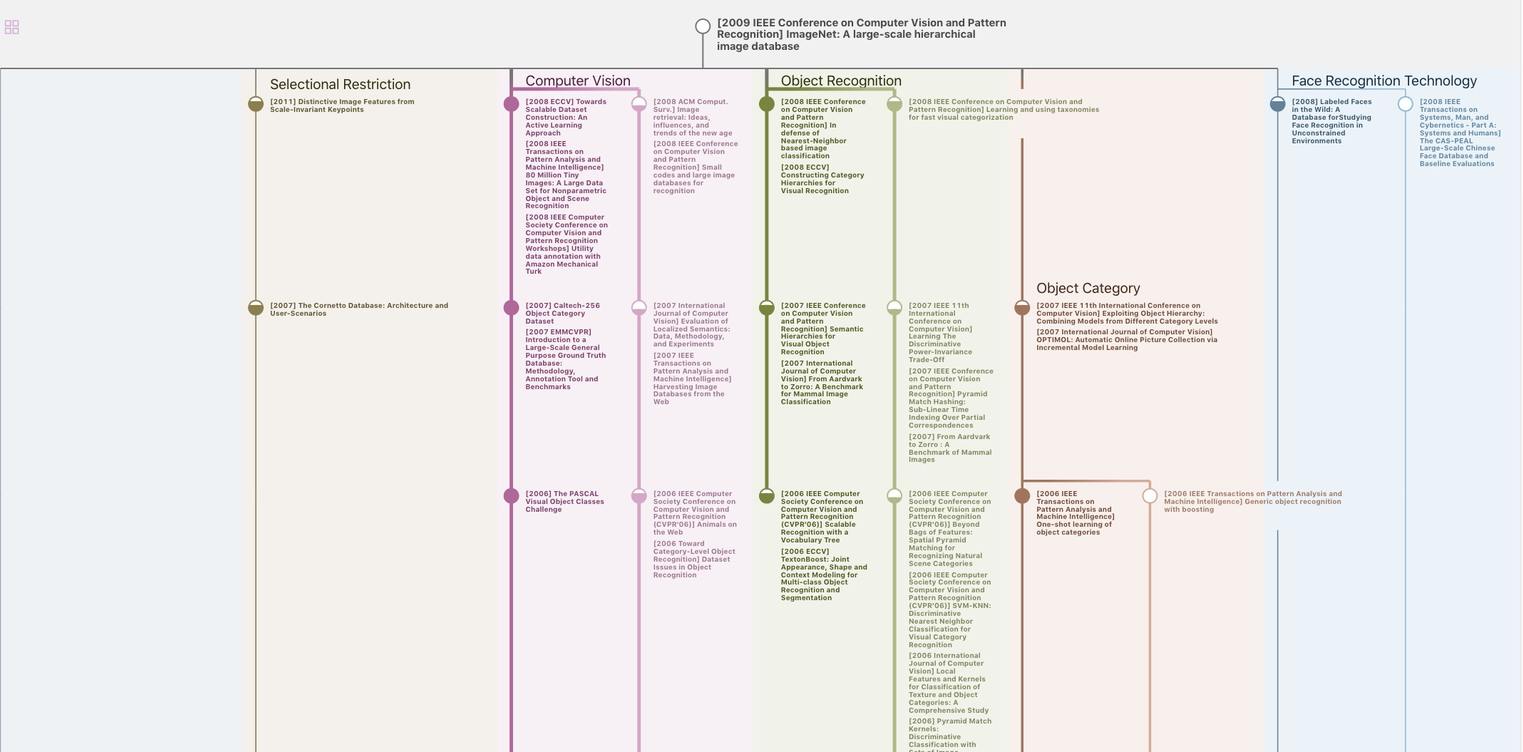
生成溯源树,研究论文发展脉络
Chat Paper
正在生成论文摘要