Web-S4AE: a semi-supervised stacked sparse autoencoder model for web robot detection
Neural Comput. Appl.(2023)
摘要
Web robots are automated computer programs that can be exploited for benign and malicious activities such as website indexing, monitoring, or unauthorized content scraping and scalping. Several methods are available to detect automated web robots through their footprints and behaviors. Although the accuracy and efficiency of existing methods depend highly on the labeled web log data, countless web requests are generated daily with the help of web robots. Exhaustive and accurate manual labeling of reconstructed sessions is time-consuming and challenging. Further, effective detection of web robots is more challenging with unlabeled or partially labeled data. To address the aforementioned issues, we reformulated web robot detection as a semi-supervised learning problem. In this paper, we propose a deep learning-based Semi-Supervised Stacked Sparse AutoEncoder (Web-S4AE) for web robot detection. The proposed model uses content-based features and features extracted from web access log data to effectively classify web robots. The experiments were conducted on publicly available web log data from a library and information portal to assess the performance of Web-S4AE. The Web-S4AE model was trained in two phases. The first phase; comprises training the model with unlabeled data to extract the hidden information, and in the second phase, the model is fine-tuned using labeled data. The results suggest that incorporating more unlabeled data can significantly improve the classifier's performance. The Web-S4AE model’s performance was also compared with other models such as the Decision Tree (DT), Random Forest (RF), eXtreme Gradient Boosting (XGBoost), and Multi-Layer Perceptron (MLP).
更多查看译文
关键词
robot,sparse,detection,semi-supervised
AI 理解论文
溯源树
样例
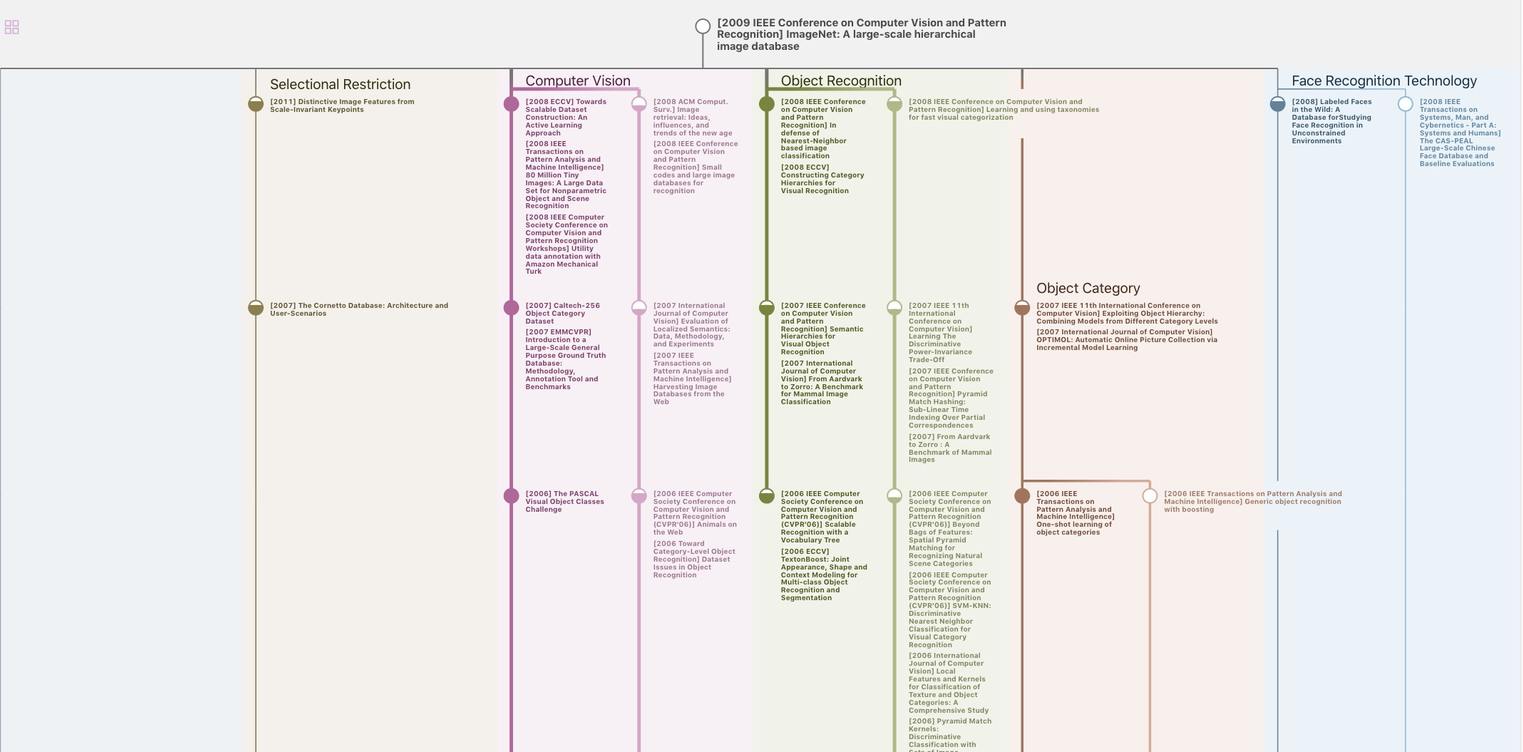
生成溯源树,研究论文发展脉络
Chat Paper
正在生成论文摘要