A Spatiotemporal Model for Global Earthquake Prediction Based on Convolutional LSTM.
IEEE Transactions on Geoscience and Remote Sensing(2023)
摘要
Deep learning has been widely used to address earthquake prediction as a time series problem. However, existing methods are often limited to local areas and lack sufficient consideration of spatial correlation and resolution. To address these limitations, we propose a new approach that uses a sequence-to-sequence framework model with a convolutional long short-term memory (ConvLSTM) network to learn both temporal and spatial correlations of seismic data on a global scale for high-resolution earthquake prediction. The approach offers several advantages over existing methods. First, we create a spatiotemporal series dataset consisting of global high-resolution seismic maps. Second, we address the problem of spatial distortion in global seismic maps by randomly rotating the seismic maps. Third, we incorporate a weighted mean squared error-mean absolute error (MSE-MAE) loss function that considers the weighted map, which helps the approach focus on areas where earthquakes may occur. Finally, our approach can deal with a 4-D dataset that integrates not only the magnitude but also the depth of every earthquake. The results demonstrate that our approach outperforms existing methods, achieving an average recall of 51.83% and precision of 64.54% on the test set when the minimum pixel unit is 72.92 $\times $ 67.71 km (longitude $\times $ latitude). The results indicate that our approach can effectively predict earthquakes with higher resolution and accuracy than previous methods, offering valuable insights into the spatiotemporal patterns of seismic activity at a global scale.
更多查看译文
关键词
Convolutional long short-term memory (ConvLSTM),global earthquake prediction,high-resolution,rotation,the weighted mean squared error-mean absolute error (MSE-MAE) loss function
AI 理解论文
溯源树
样例
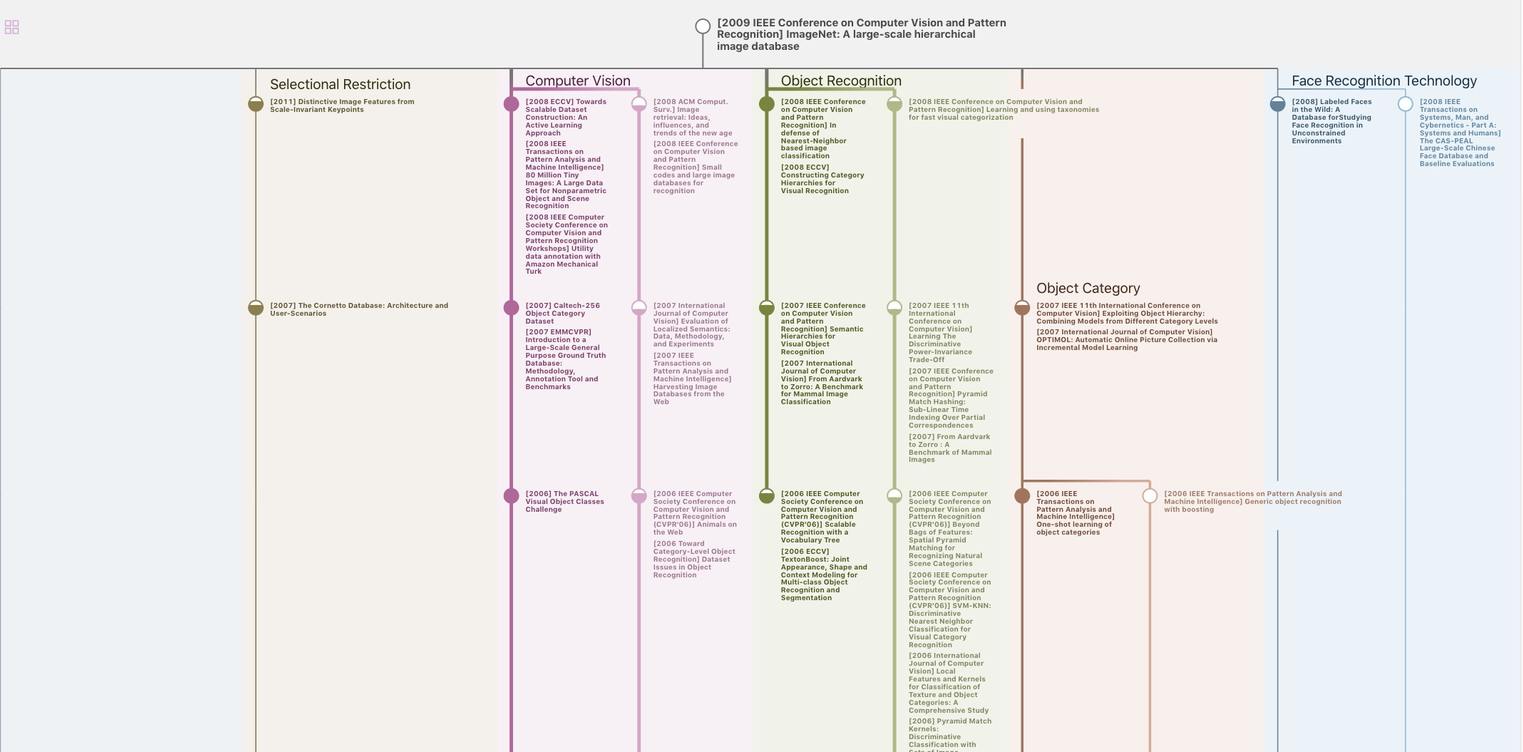
生成溯源树,研究论文发展脉络
Chat Paper
正在生成论文摘要