Cross-Scale-Guided Fusion Transformer for Disaster Assessment Using Satellite Imagery.
IEEE Trans. Geosci. Remote. Sens.(2023)
摘要
When a disaster strikes, accurate disaster information and effective response are critical for saving lives and properties. High-resolution satellite (HRS) imagery provides valuable geographical information that can assist experts in analyzing damage levels in different areas and enacting appropriate relief plans. However, analyzing large HRS images is both time-consuming and inefficient, requiring efficient automated methods to replace expert analysis. Fortunately, deep learning methods have achieved impressive performance on HRS image processing tasks, considerably increasing automation levels. Despite this progress, most HRS-based damage assessment methods only consider a single time series of postdisaster images or simply integrate pre- and postdisaster images, lacking the integration of effective information between pre- and postdisaster images. To alleviate this problem, we propose a two-stage multiscale fusion network that fully exploits the information contained in pre- and postdisaster images. Specifically, we employ a hierarchical transformer to accurately locate buildings by predisaster images in the first stage, and then propose the guided fusion (GF) and cross-scale GF modules in the second stage to efficiently utilize both pre- and postdisaster images. Our method outperforms state-of-the-art (SOTA) methods in building segmentation and building damage assessment on the xBD dataset, and exhibits improved generalization across diverse geographic regions and disaster types.
更多查看译文
关键词
Cross-scale-guided fusion (CSGF),damage assessment,satellite imagery,siamese transformer
AI 理解论文
溯源树
样例
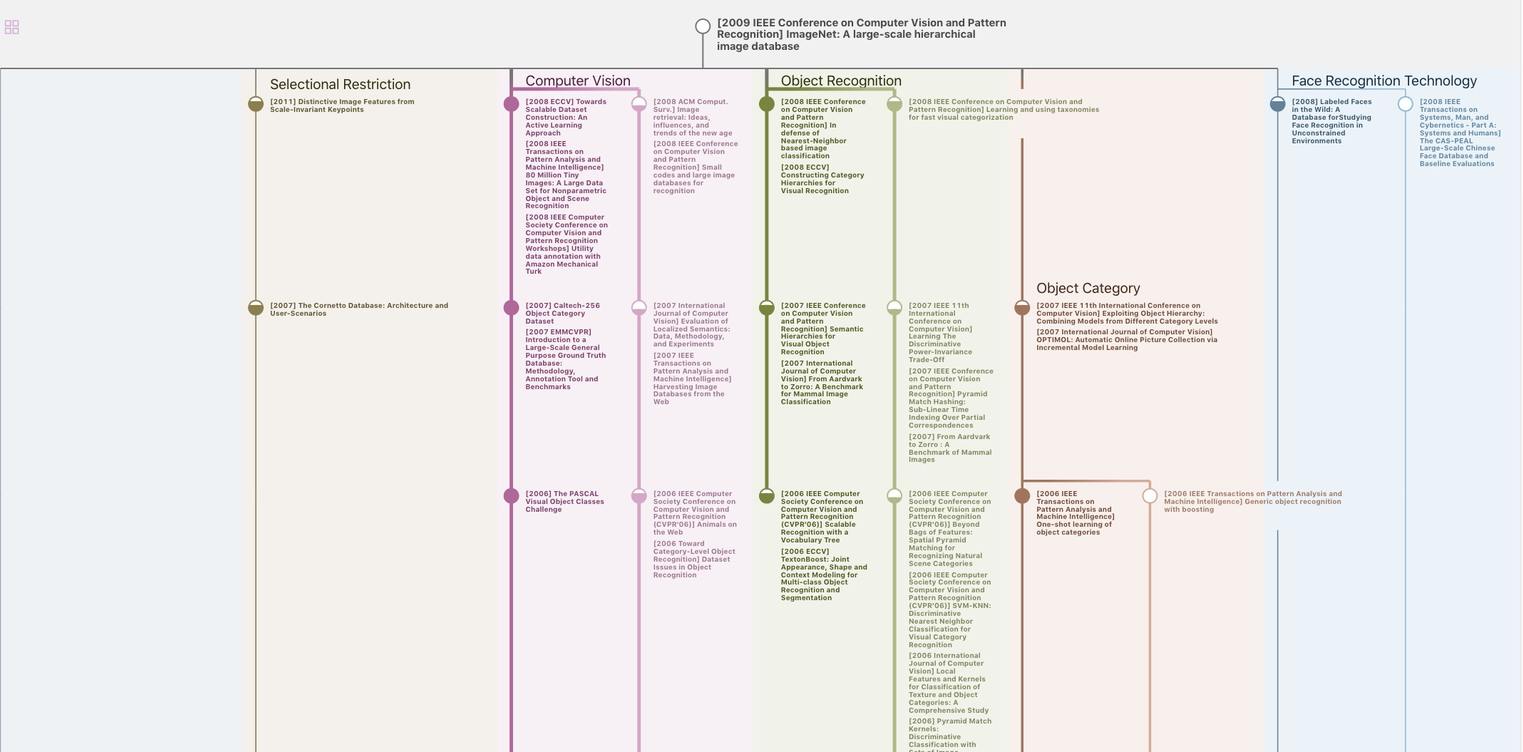
生成溯源树,研究论文发展脉络
Chat Paper
正在生成论文摘要