Multisensor Temporal Unsupervised Domain Adaptation for Land Cover Mapping With Spatial Pseudo-Labeling and Adversarial Learning.
IEEE Trans. Geosci. Remote. Sens.(2023)
摘要
With the huge variety of Earth observation satellite missions available nowadays, the collection of multisensor remote sensing information depicting the same geographical area has become systematic in practice, paving the way to the further breakthroughs in automatic land cover mapping with the aim to support decision makers in a variety of land management applications. In this context, along with the increase in the volume of data available, the availability of ground-truth (GT) data to train supervised models, which is usually time-consuming and costly, may even be more critical. In this scenario, the possibility to transfer a model learned on a particular time span (source domain) to a different period of time (target domain), over the same geographical area, can be advantageous in terms of both cost and time efforts. However, such model transfer is challenging due to different climate, weather, or environmental conditions affecting remote sensing data collected at different time periods, resulting in possible distribution shifts between the source and target domains. With the aim to cope with the multisensor temporal transfer scenario in the context of land cover mapping, where multitemporal and multiscale information are used jointly, we propose a Multisensor, Multitemporal, and Multiscale SPatially Aware Domain Adaptation (M3SPADA) framework, a deep learning methodology that jointly exploits self-training and adversarial learning to transfer a multisensor land cover classifier (MSLCC) from a time period (year) to a different one on the same geographical area. Here, we consider the case in which each domain (source and target) is described by a pair of remote sensing datasets: a satellite image time series (SITS) of optical images and a single very high spatial resolution (VHR) scene. Experimental evaluation on a real-world study case located in Burkina Faso and characterized by operational constraints shows the quality of our proposal to deal with the temporal multisensor transfer in the context of land cover mapping.
更多查看译文
关键词
Data fusion,deep learning,multisensor land cover mapping,satellite image time series (SITS),temporal domain adaptation,very high spatial resolution (VHR) imagery
AI 理解论文
溯源树
样例
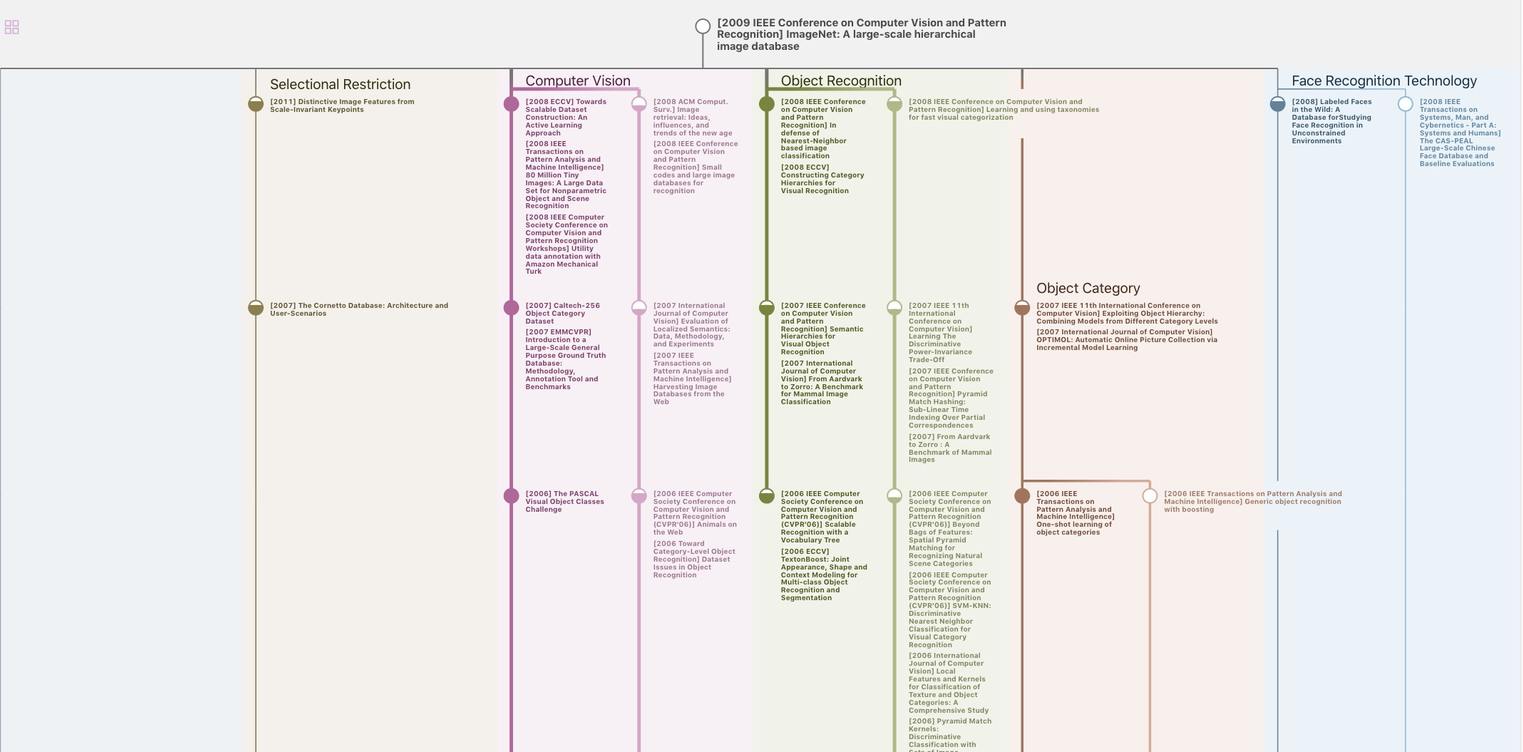
生成溯源树,研究论文发展脉络
Chat Paper
正在生成论文摘要